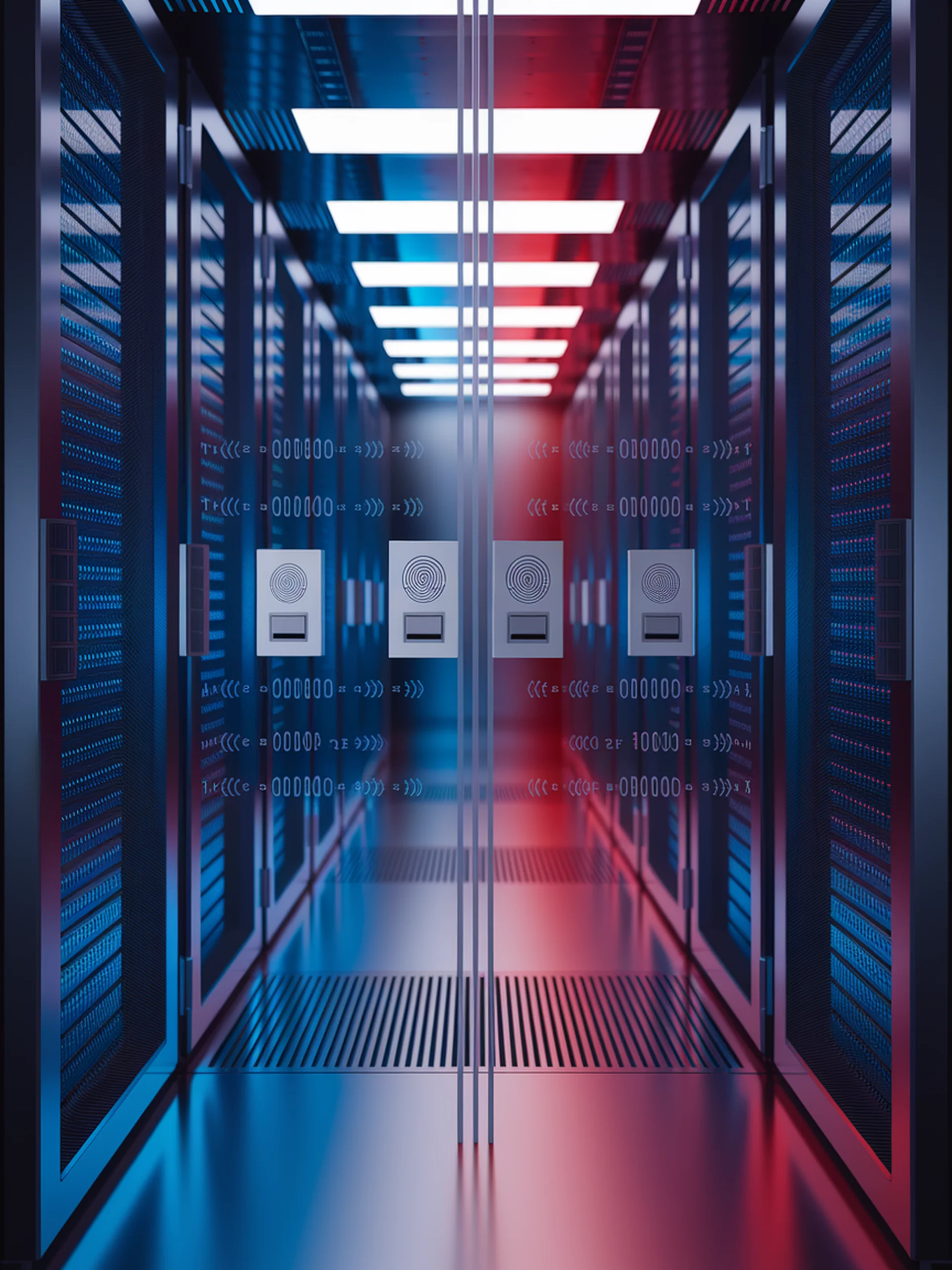
Securing LLMs with Access Control
Protecting sensitive data while maintaining model performance
DOMBA introduces a novel technique for preventing LLMs from leaking restricted information while preserving their functionality for authorized users.
- Creates two separate models - one trained on public data, another on restricted data
- Employs minimum-bounded aggregation to combine model outputs based on user access privileges
- Provides mathematical security guarantees against information leakage to unauthorized users
- Maintains high utility for authorized users with minimal performance degradation
This research addresses critical security challenges for organizations deploying LLMs on sensitive internal data, allowing controlled information sharing while protecting confidential content.
DOMBA: Double Model Balancing for Access-Controlled Language Models via Minimum-Bounded Aggregation