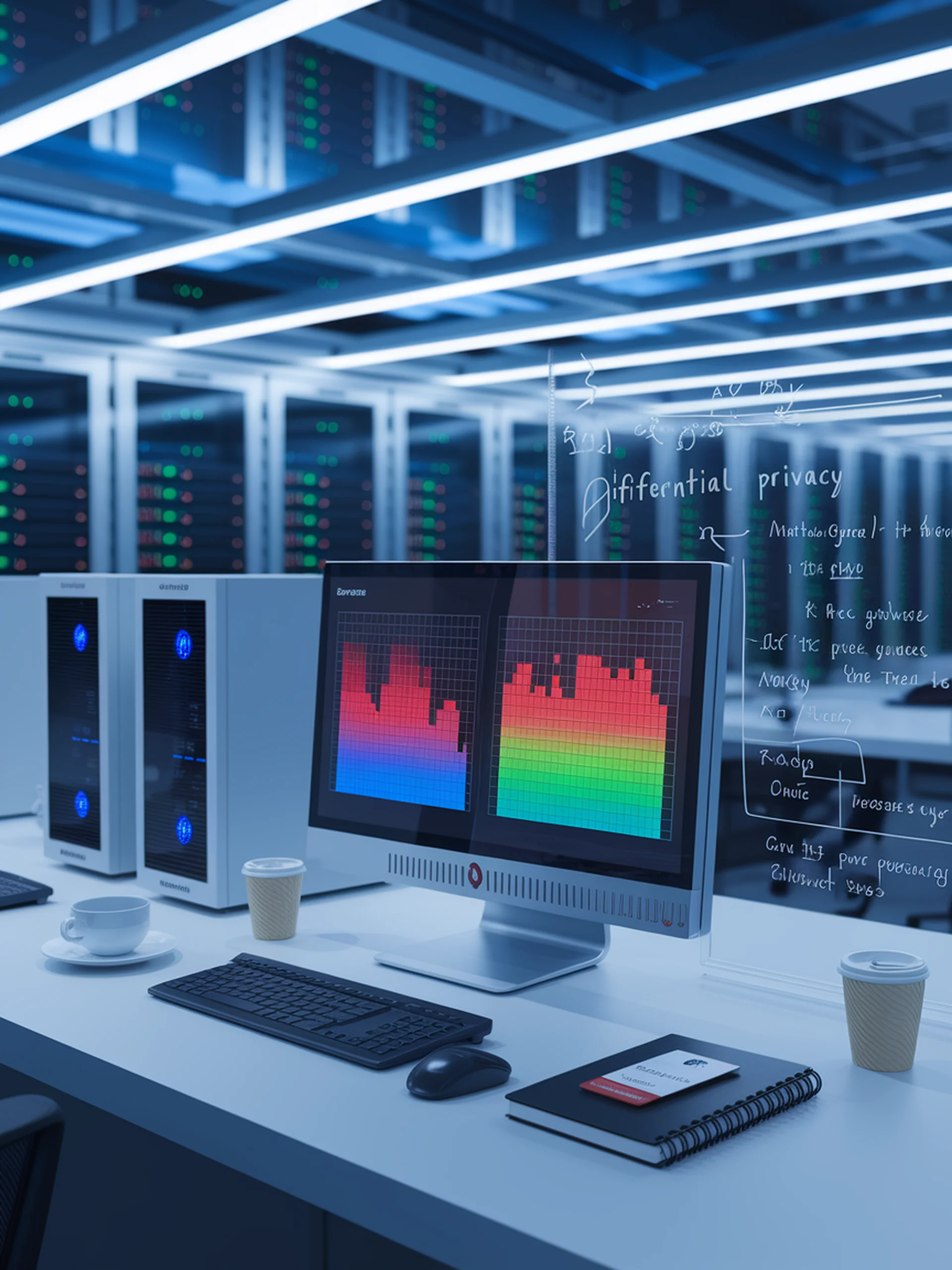
Improving Privacy in Machine Learning Models
A new heuristic analysis for DP-SGD's last iterate advantage
This research presents a novel heuristic for estimating privacy leakage in differentially private machine learning, focusing on the last iterate of stochastic gradient descent.
- Introduces a simple linear heuristic to predict privacy leakage when only the final model is released
- Experimentally validates the heuristic through privacy auditing across various training procedures
- Provides a practical way to estimate privacy risks before training begins
- Demonstrates the security advantages of releasing only the last iterate rather than all intermediate models
For security professionals, this research offers a practical tool to assess privacy vulnerabilities in machine learning deployments without expensive post-training audits, helping organizations better protect sensitive data used in AI development.