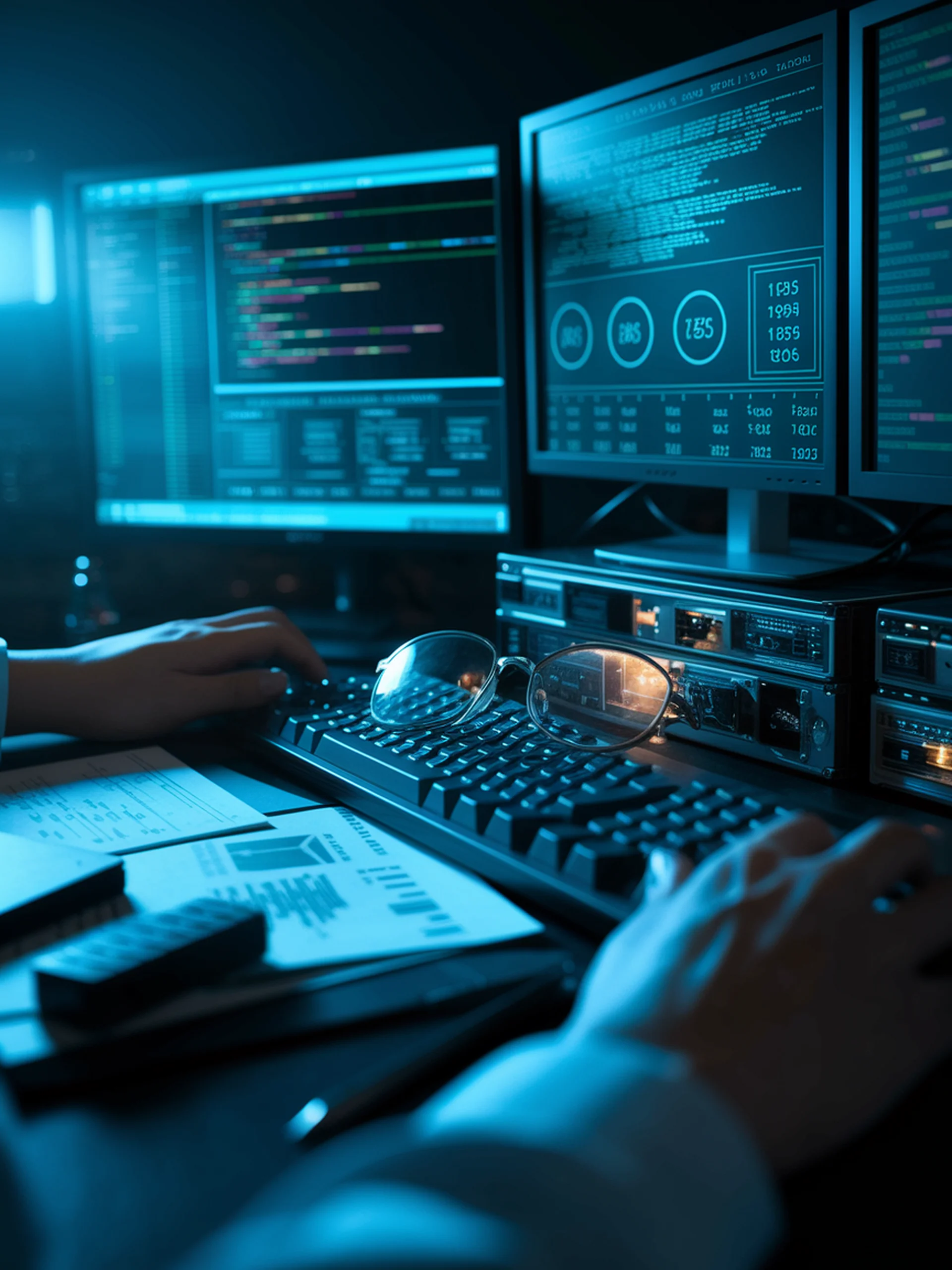
Detecting LLM Training Data
New Fine-tuning Method Improves Detection of Pretraining Data
This research introduces a novel approach to determine if specific data was used to train large language models, addressing a critical security gap in AI evaluation and use.
- Proposes Fine-tuned Score Deviation (FSD) technique that improves detection accuracy
- Demonstrates that fine-tuning smaller models on known data creates better detection capabilities
- Achieves significantly better results than traditional methods like perplexity testing
- Provides a practical tool for auditing LLMs and reducing ethical risks
This work matters for security professionals as it offers a robust method to verify model training compliance, detect potential copyright violations, and ensure fair model comparisons - crucial steps toward more transparent and trustworthy AI systems.
Fine-tuning can Help Detect Pretraining Data from Large Language Models