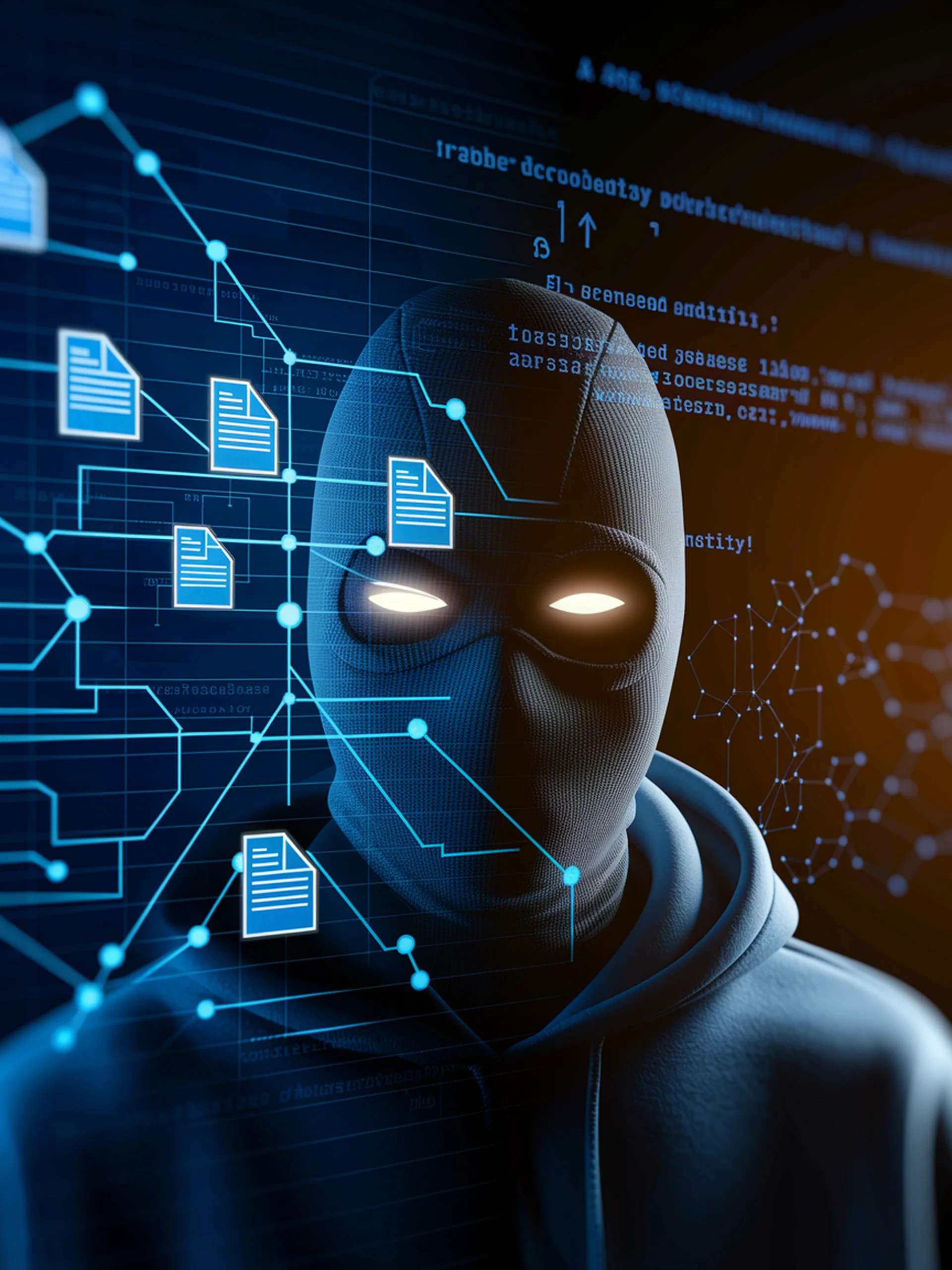
Unmasking the Vulnerabilities in RAG Systems
Novel attack methods reveal security risks in retrieval-augmented LLMs
This research introduces Mask-based Membership Inference Attacks (M-MIAs), exposing critical security vulnerabilities in Retrieval-Augmented Generation (RAG) systems that can reveal whether specific documents are stored in knowledge databases.
- Demonstrates three novel attack methods with success rates up to 94% in detecting document presence
- Reveals that RAG systems can leak information about stored documents through response patterns
- Shows how attackers can exploit these vulnerabilities without requiring direct database access
- Proposes potential defensive measures to protect sensitive or copyrighted information
These findings highlight significant privacy and intellectual property risks as companies increasingly store sensitive or copyrighted data in RAG knowledge bases rather than directly fine-tuning LLMs.
Mask-based Membership Inference Attacks for Retrieval-Augmented Generation