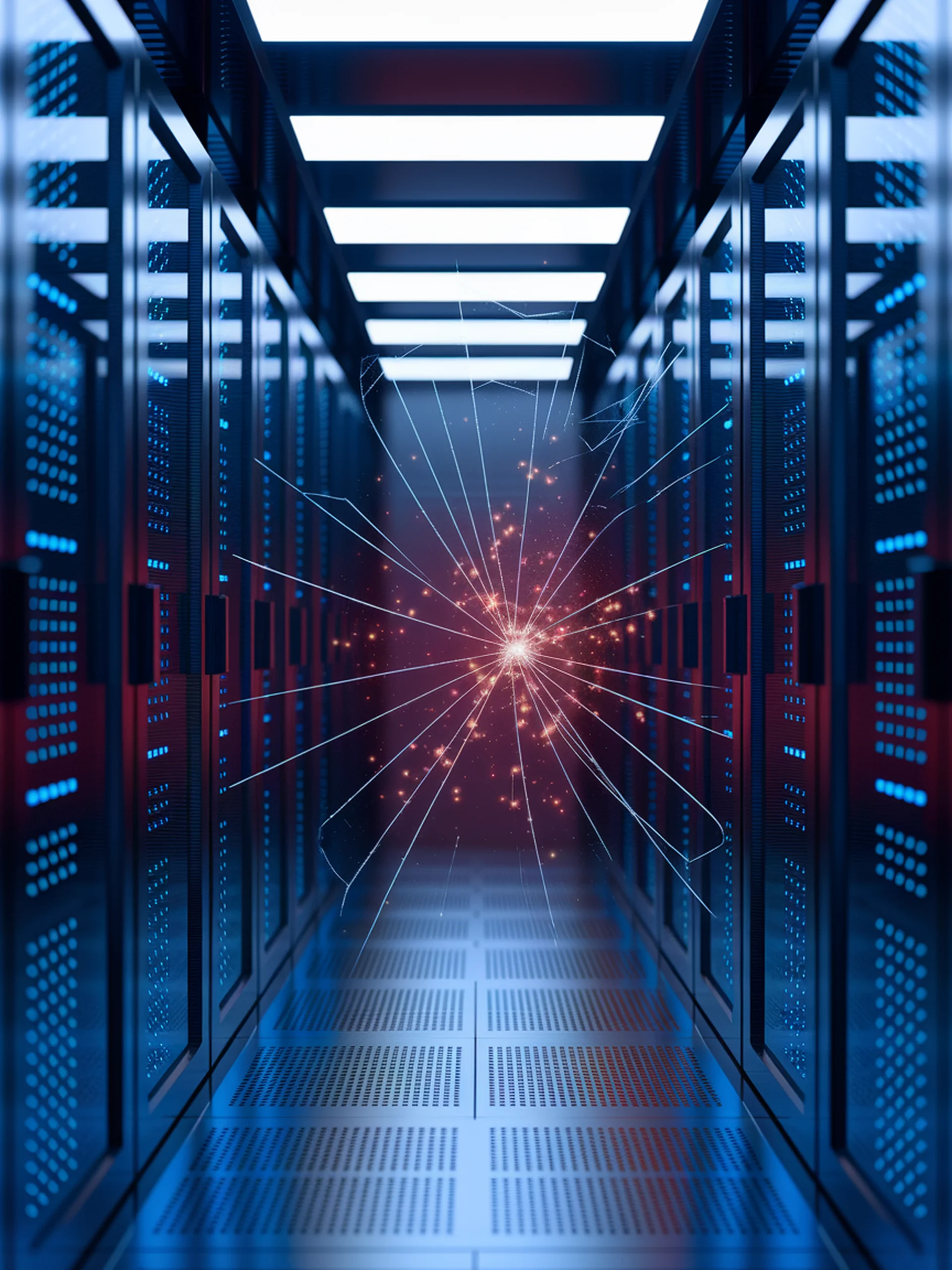
When Privacy Attacks Actually Work on LLMs
New evidence shows large language models are vulnerable to specific membership inference attacks
This research challenges the prevailing belief that membership inference attacks (MIA) are ineffective against large language models, identifying specific conditions where these privacy attacks succeed.
- High-precision attacks are possible when targeting memorized data and using appropriate attack methods
- Model size matters - larger models exhibit stronger vulnerability to membership inference
- Repetition in training data significantly increases attack success rates
- Effective MIA remains highly challenging for non-memorized data portions
This work has critical security implications as it demonstrates real privacy vulnerabilities in LLMs, potentially enabling detection of unauthorized training data usage and copyright violations.
Scaling Up Membership Inference: When and How Attacks Succeed on Large Language Models