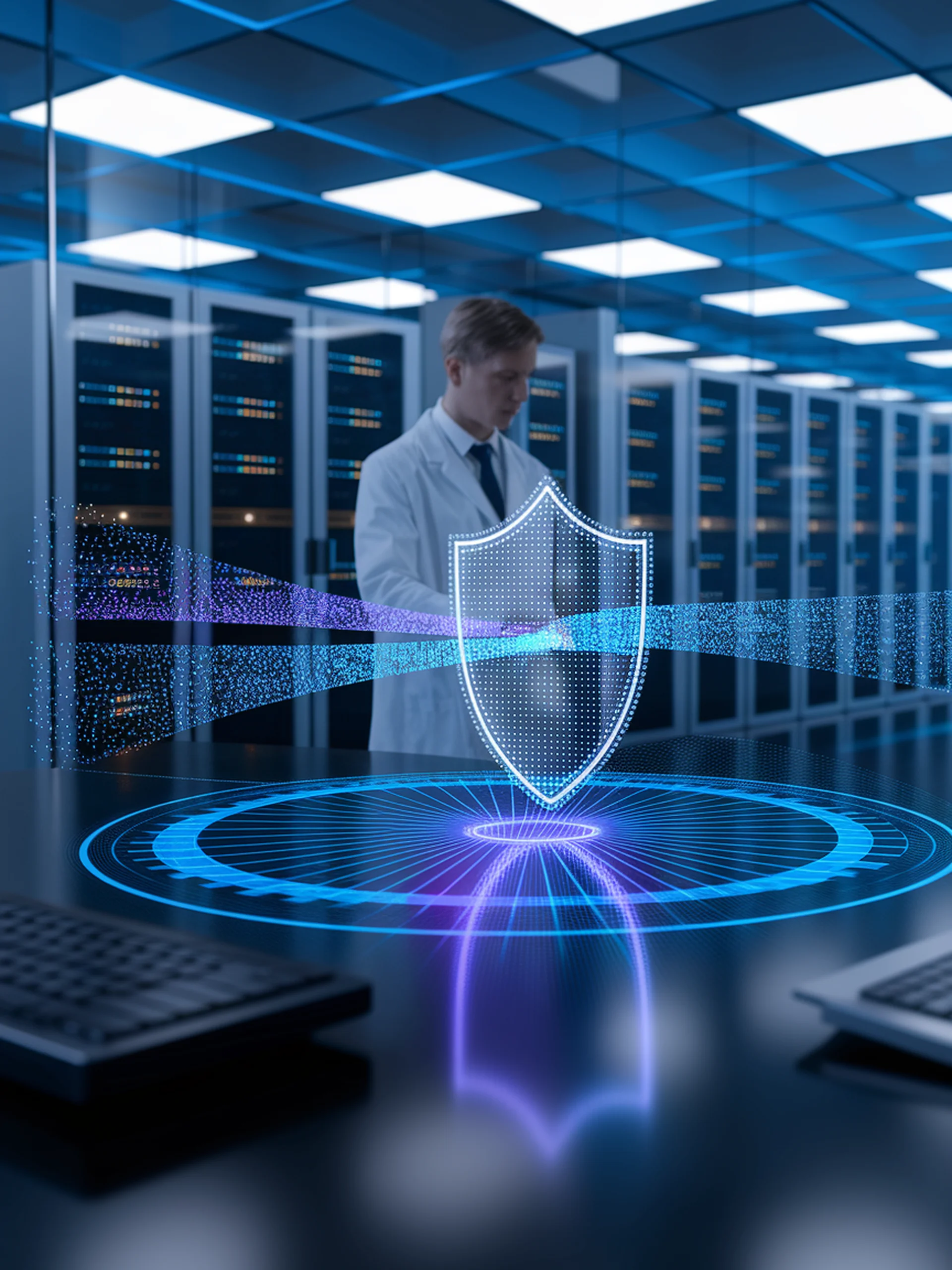
Privacy-Preserving Synthetic Data
Generating high-quality data while protecting privacy through multi-model fusion
This research introduces a novel approach for synthesizing private data that maintains differential privacy guarantees while improving data quality through weighted fusion of multiple pre-trained language models.
- Addresses the challenge of generating high-quality synthetic data in data-deficient scenarios
- Implements Differential Privacy (DP) techniques to provide formal privacy guarantees
- Uses contrastive learning with multiple pre-trained models to enhance synthetic data quality
- Particularly valuable for security-sensitive applications where both data quality and privacy protection are essential
This innovation enables organizations to train models on sensitive data while maintaining stringent privacy protections, creating new possibilities for secure AI development in regulated industries.
Contrastive Private Data Synthesis via Weighted Multi-PLM Fusion