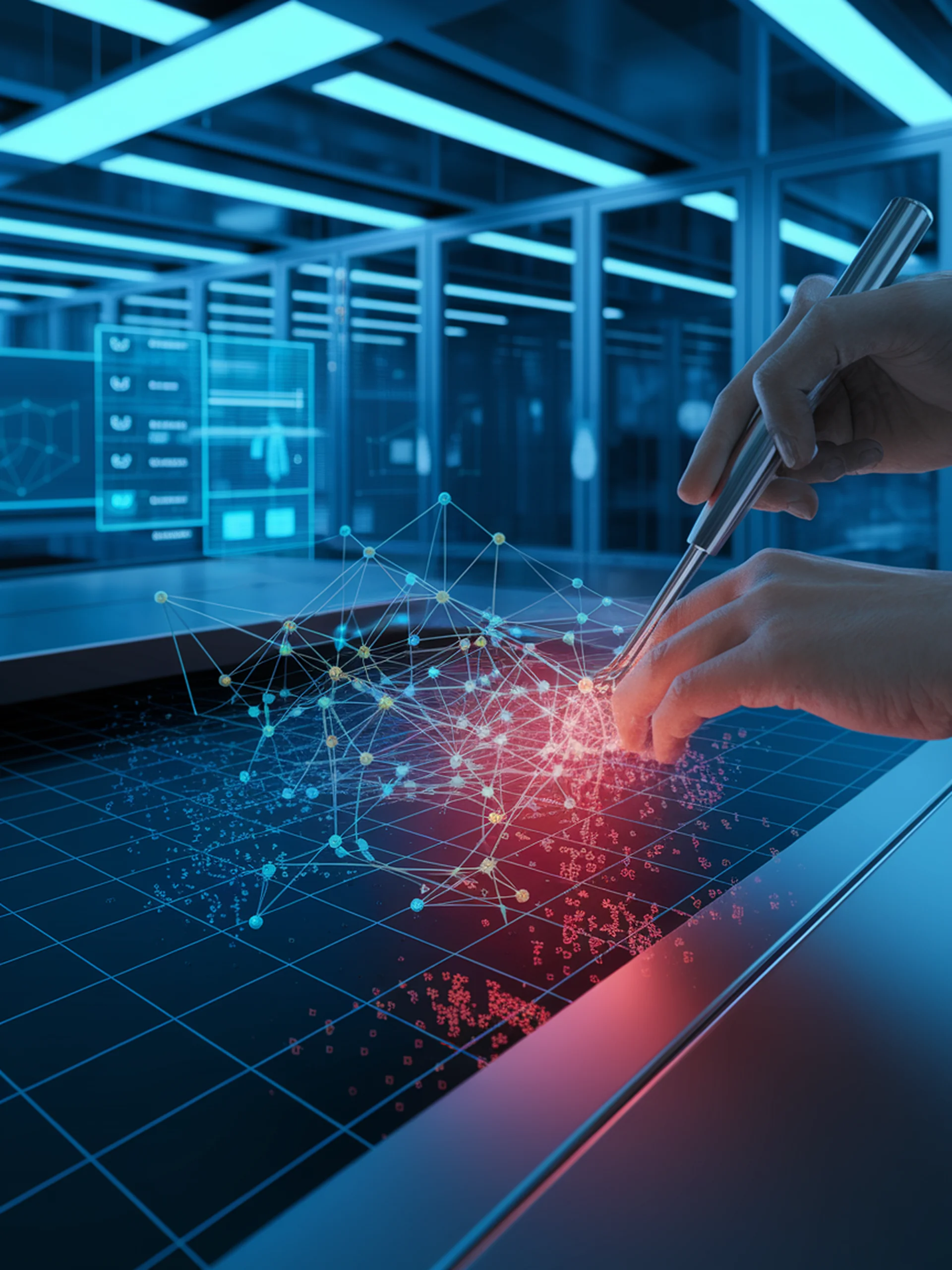
Pruning: A Simple Defense Against AI Memory Leaks
How model pruning reduces data memorization in Large Language Models
This research demonstrates how simple pruning techniques can significantly reduce unwanted data memorization in LLMs, offering an effective defense against privacy attacks.
- Pruning effectively decreases the extent to which LLMs reproduce memorized training data
- Positions model pruning as a practical approach to mitigate membership inference attacks
- Provides a straightforward yet powerful technique for enhancing LLM privacy and security
- Demonstrates important tradeoffs between model utility and memorization reduction
For security professionals, this research offers a viable technique to address privacy concerns in AI deployment without requiring complex architectural changes or expensive retraining processes.
Pruning as a Defense: Reducing Memorization in Large Language Models