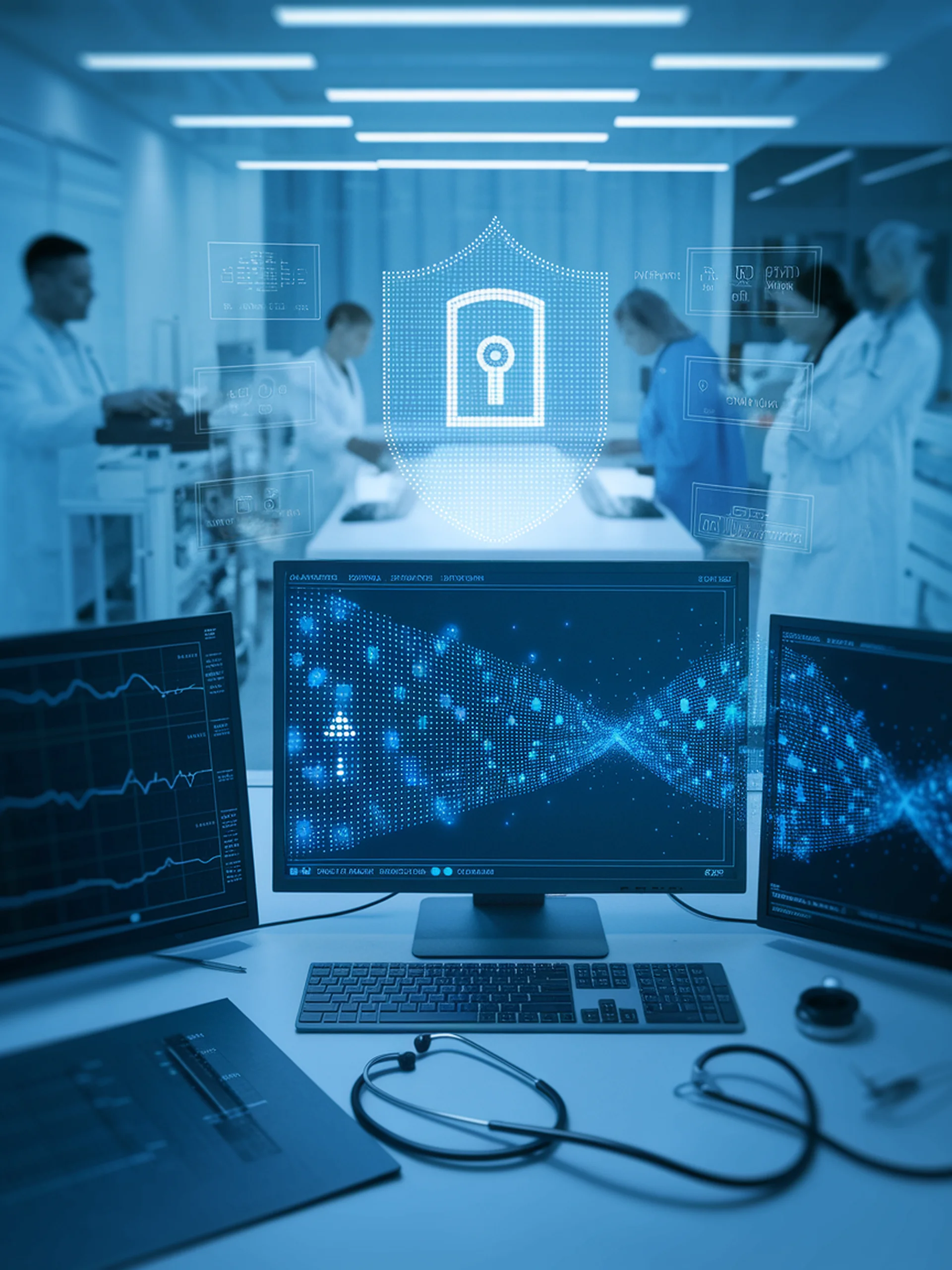
Privacy-Preserving LLMs in Healthcare
Generating high-quality synthetic data for sensitive domains
RewardDS is a novel framework that enables fine-tuning LLMs with synthetic data while preserving privacy in sensitive domains like healthcare and finance.
- Uses a reward-driven approach to synthesize high-quality training data with Differential Privacy guarantees
- Identifies and removes flawed synthetic data through an innovative filtering mechanism
- Achieves superior performance compared to existing privacy-preserving methods
- Maintains model utility while providing strong privacy protection
This research is particularly valuable for the medical sector, where organizations can fine-tune domain-specific LLMs without exposing patient data, enabling advanced AI applications while maintaining regulatory compliance and patient trust.
RewardDS: Privacy-Preserving Fine-Tuning for Large Language Models via Reward Driven Data Synthesis