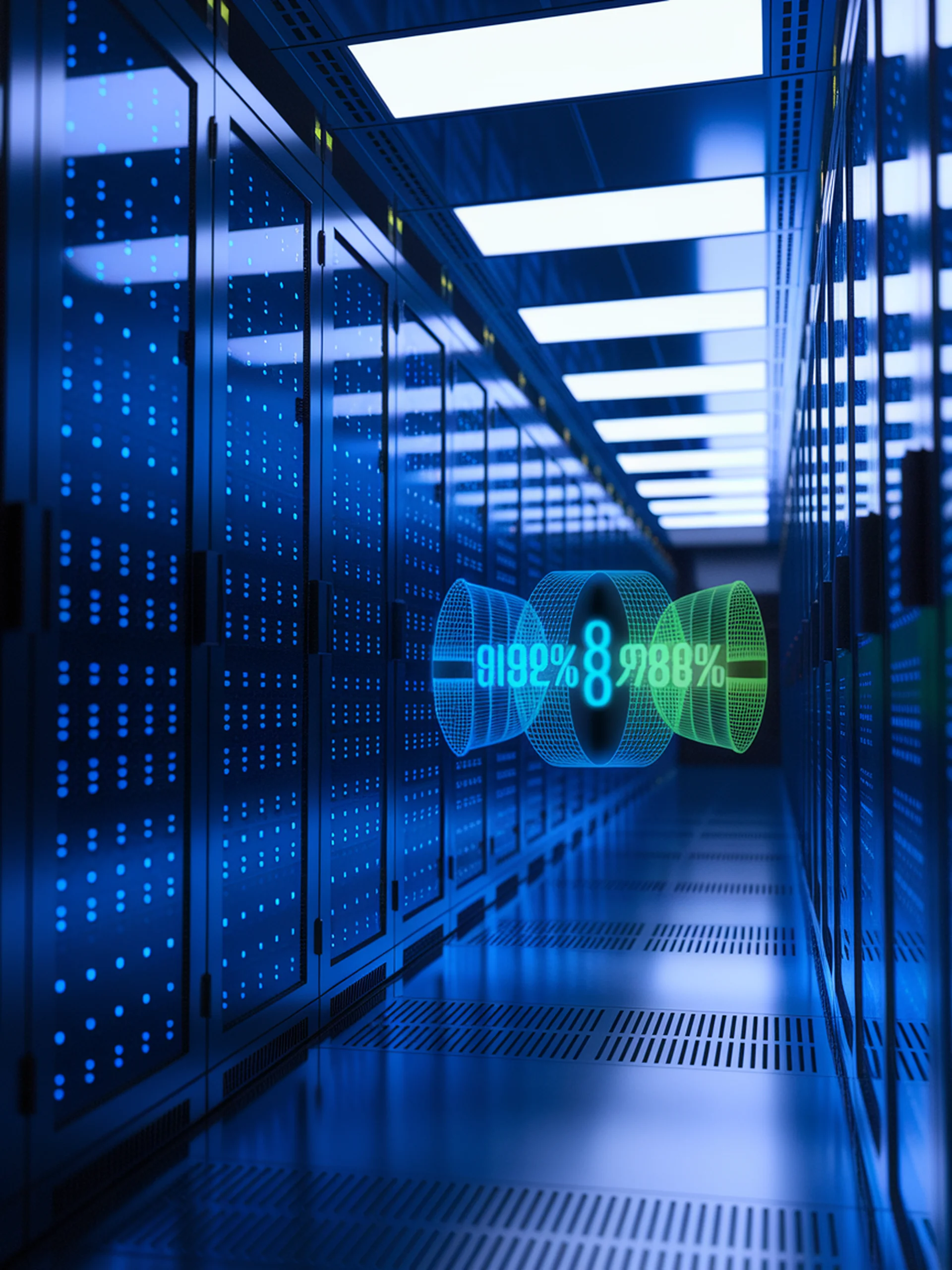
Smarter Model Storage for Privacy-Preserving AI
Balancing Storage Efficiency with Privacy Guarantees
Novel approach to deduplicate AI models trained with privacy-preserving techniques while maintaining strict privacy guarantees and reducing storage costs.
- Introduces a privacy-aware deduplication framework that identifies shared model components without compromising privacy budgets
- Achieves up to 80% reduction in storage requirements while preserving model utility
- Provides mathematical guarantees for privacy preservation across deduplicated model versions
- Enables organizations to efficiently manage multiple model versions with varying privacy-utility tradeoffs
This research is crucial for security teams deploying privacy-preserving ML at scale, allowing them to maintain compliance with privacy regulations while optimizing infrastructure costs.