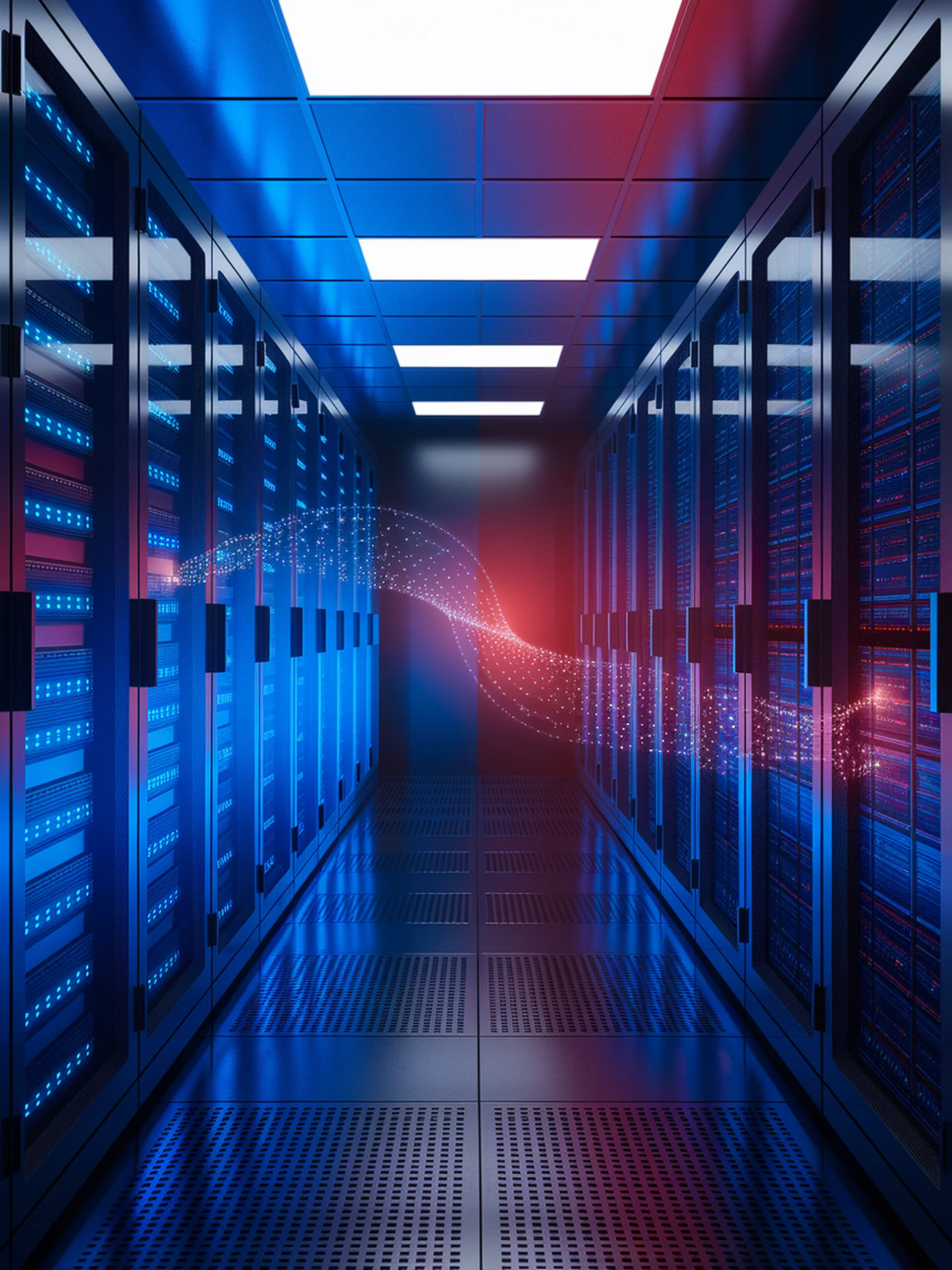
Security Vulnerabilities in Distributed LLM Inference
How attackers can reconstruct private prompts from intermediate outputs
This research reveals critical security vulnerabilities in frameworks that distribute LLM inference across multiple devices to overcome resource constraints.
- Identifies prompt inference attacks that can reconstruct user prompts from transmitted intermediate outputs
- Demonstrates three effective attack scenarios with varying levels of adversary knowledge
- Shows that attackers can accurately recover substantial portions of user prompts, compromising privacy
- Highlights an urgent need for secure design principles in distributed LLM inference systems
As distributed LLM deployment grows for resource-constrained environments, these findings emphasize the critical importance of implementing robust privacy safeguards before widespread adoption.
Prompt Inference Attack on Distributed Large Language Model Inference Frameworks