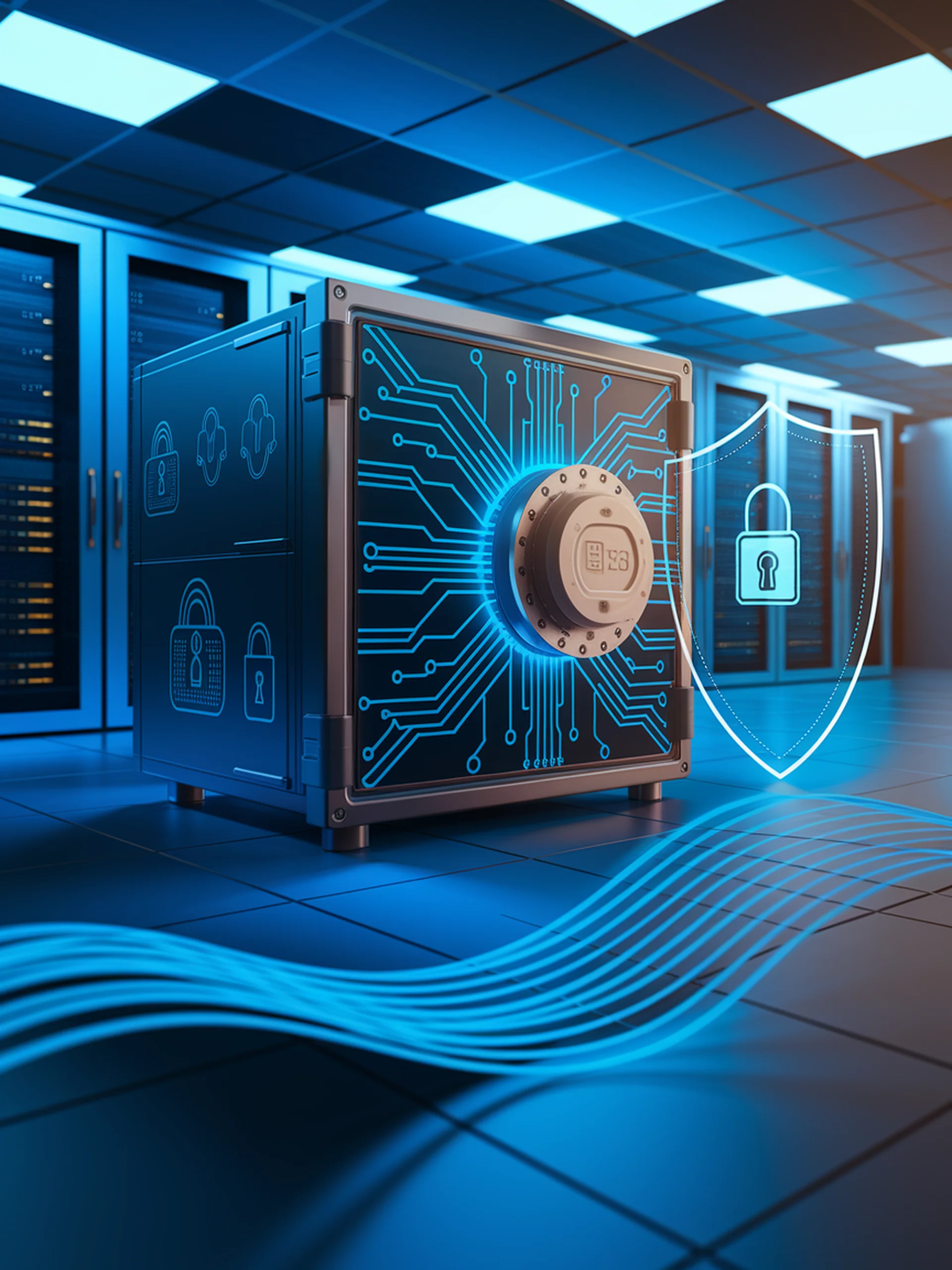
Securing LLM Interactions
A cryptographic approach to protecting sensitive information in prompts
Pr$εε$mpt introduces a formal privacy protection system for sensitive information in LLM prompts while maintaining response quality.
- Implements prompt sanitizers that transform input prompts to protect sensitive tokens
- Utilizes format-preserving encryption and differential privacy techniques
- Provides a cryptographically-inspired solution to critical security concerns in LLM APIs
- Addresses the growing challenge of privacy leakage during LLM inference
This research is critical for organizations using third-party LLM services, as it offers a practical framework for protecting proprietary or sensitive information while still leveraging powerful AI capabilities.