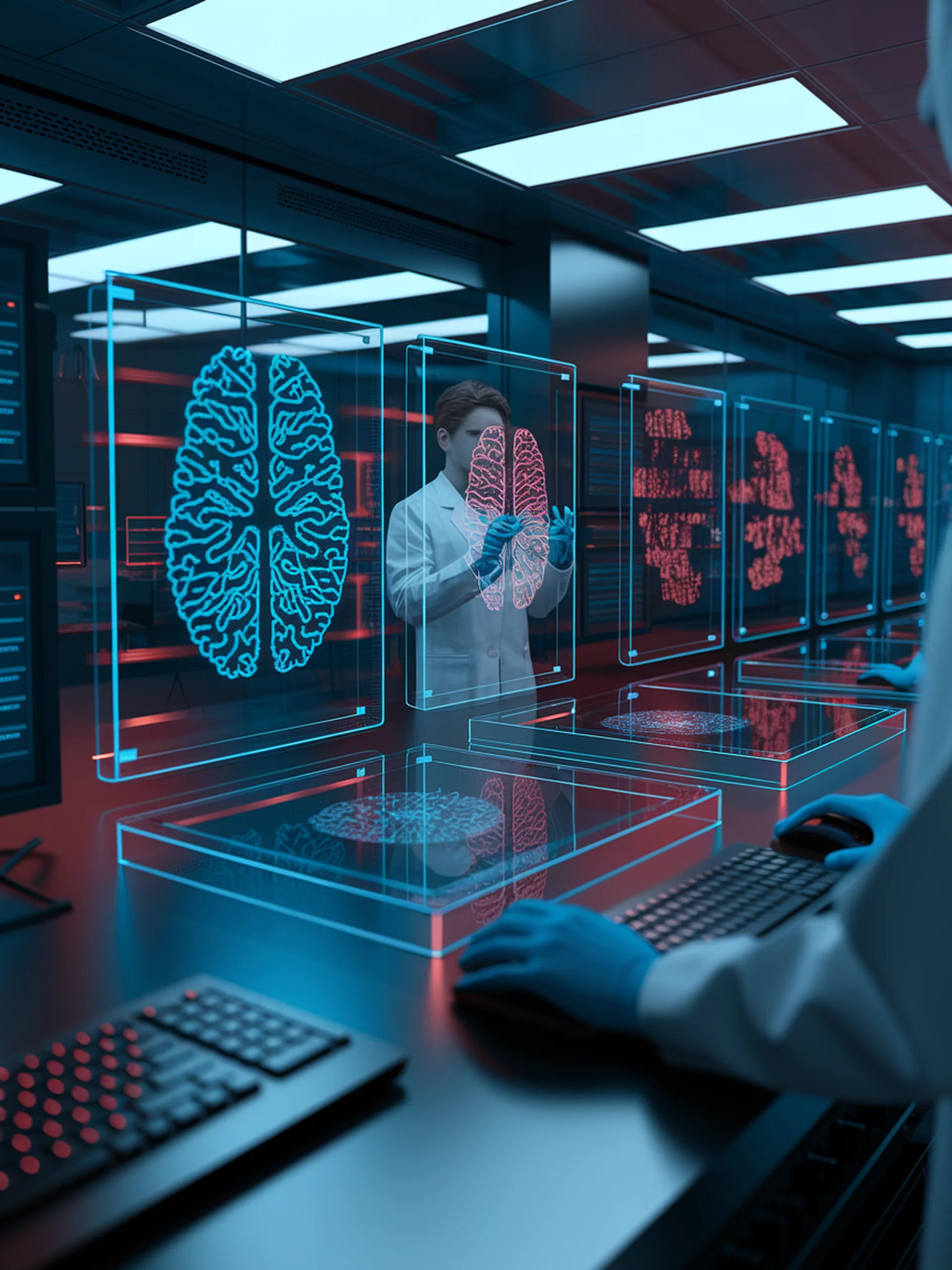
Detecting LLM Manipulation
A Novel Approach to Identify Prompt Injections Using Activation Patterns
This research introduces Activation Delta Detection, a method to identify when large language models are being manipulated away from their intended tasks by malicious inputs.
- Uses patterns in hidden layer activations to detect when an LLM is being diverted from its original task
- Works without requiring knowledge of the attack content or the exact tasks
- Achieved over 90% accuracy in detecting prompt injections across various attack scenarios
- Provides a defense mechanism that doesn't require model retraining or extensive computational resources
This research is crucial for security as LLMs become more integrated with external data sources in applications like search engines and email plugins, where prompt injection attacks can lead to data exfiltration or manipulated outputs.
Get my drift? Catching LLM Task Drift with Activation Deltas