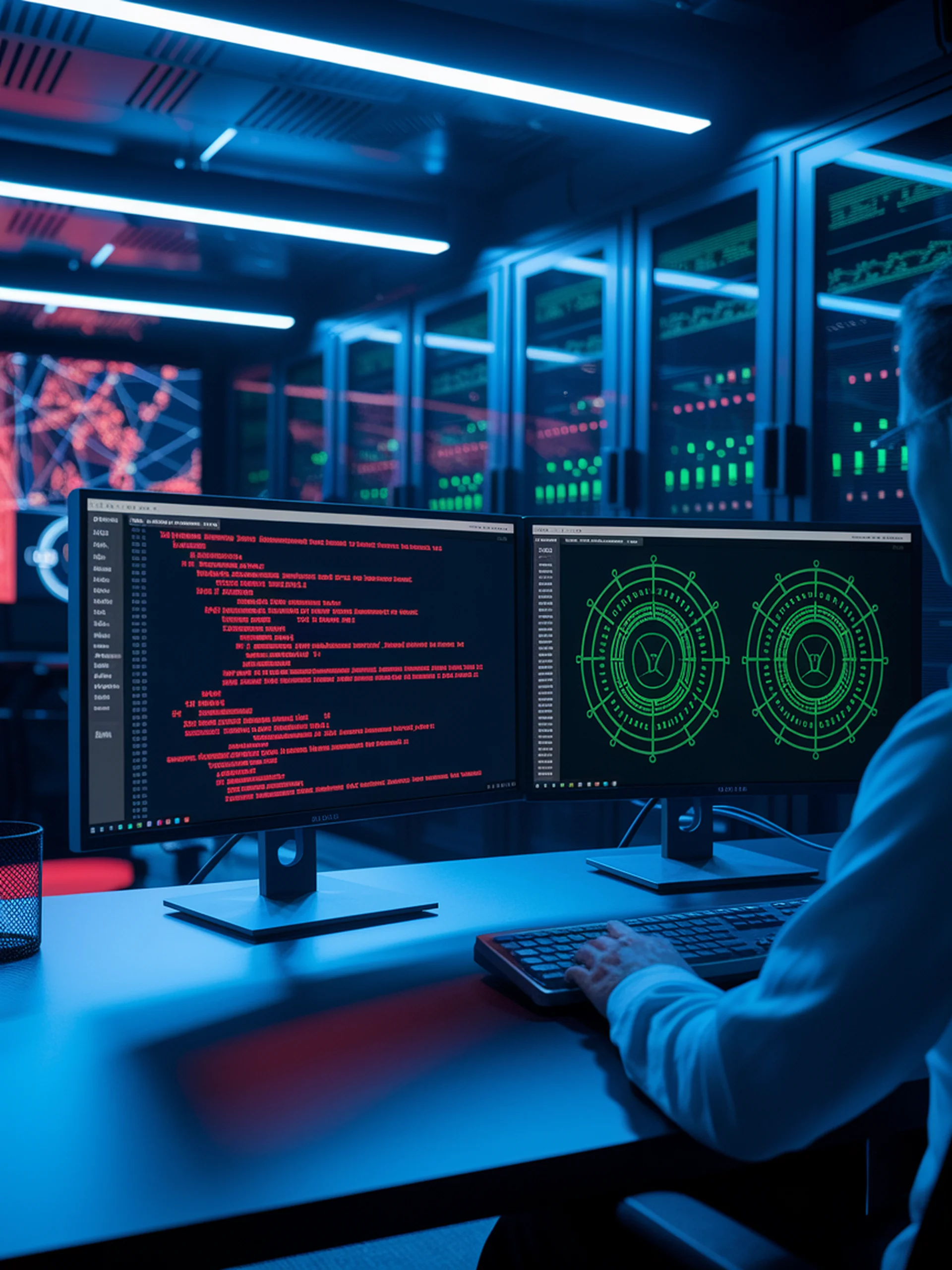
Defending LLMs Against Prompt Injection
Novel defense techniques using attackers' own methods
This research develops an innovative approach to protect LLM-integrated applications by turning attack techniques into defense mechanisms.
- Creates a defense framework that actively detects and mitigates prompt injection attacks
- Employs reverse-engineering of attack patterns to strengthen defenses
- Demonstrates practical implementation with significant improvement in security posture
- Shows potential for real-world application in commercial LLM systems
This work addresses critical security vulnerabilities in conversational AI systems that can lead to unauthorized actions, data leakage, and compliance violations when exploited through carefully crafted prompts.
Defense Against Prompt Injection Attack by Leveraging Attack Techniques