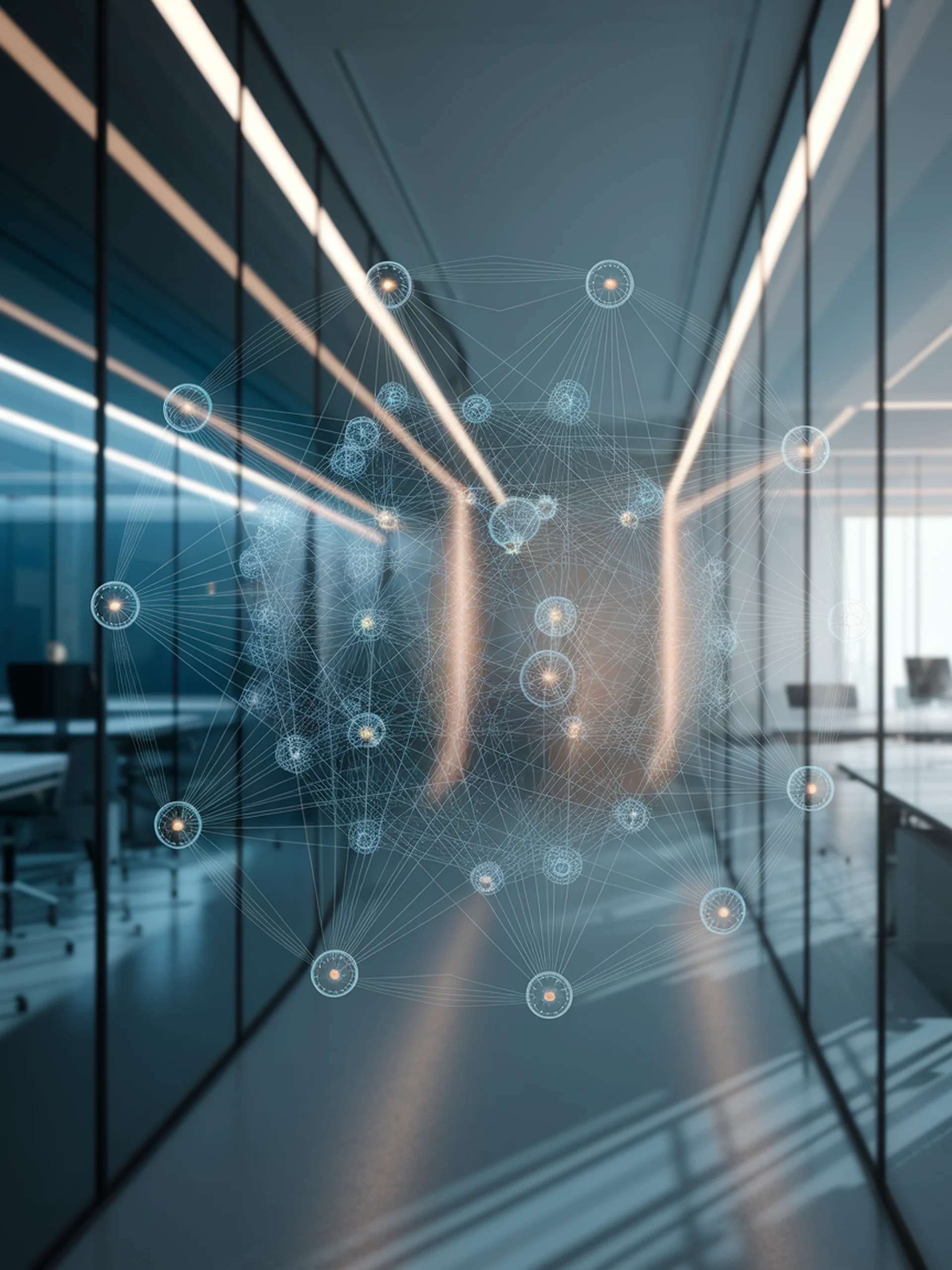
Verifiable Commonsense Reasoning in LLMs
Enhancing knowledge graph QA with transparent reasoning paths
This research introduces a novel framework for verifiable commonsense reasoning in Large Language Models when answering questions using Knowledge Graphs.
- Develops a specialized approach for commonsense questions beyond factual queries
- Implements traceable reasoning procedures to verify LLM responses
- Significantly reduces hallucination by 76% compared to existing methods
- Creates the first benchmark dataset for commonsense knowledge graph QA
For security applications, this advancement offers crucial transparency in AI decision-making, enabling verification of reasoning paths and increasing trust in LLM outputs in critical information systems.