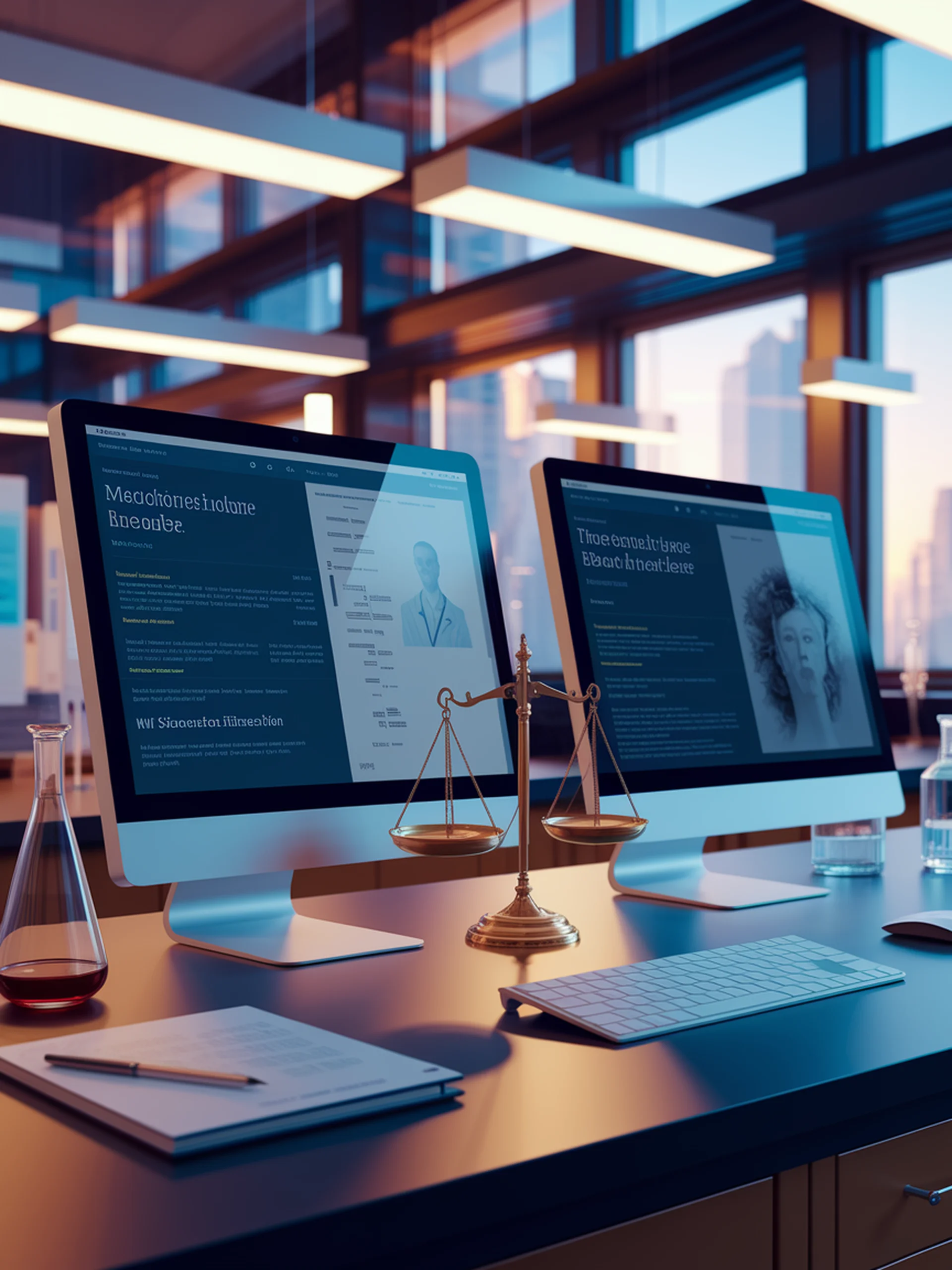
When LLMs Clash With Evidence
Measuring how language models balance prior knowledge against retrieved information
This research evaluates how language models handle contradictions between their internal knowledge and external evidence in retrieval-augmented generation (RAG) systems.
- Introduces ClashEval, a framework to quantify the tug-of-war between LLM priors and retrieved information
- Analyzes how different models react to incorrect information in RAG contexts
- Reveals variation in how models handle evidence across domains like medicine and education
- Provides insights for developing more robust RAG systems that appropriately weigh evidence
Medical Impact: Critical for medical AI applications where models must properly evaluate drug dosage information to prevent harmful outcomes from incorrect retrieved content.
ClashEval: Quantifying the tug-of-war between an LLM's internal prior and external evidence