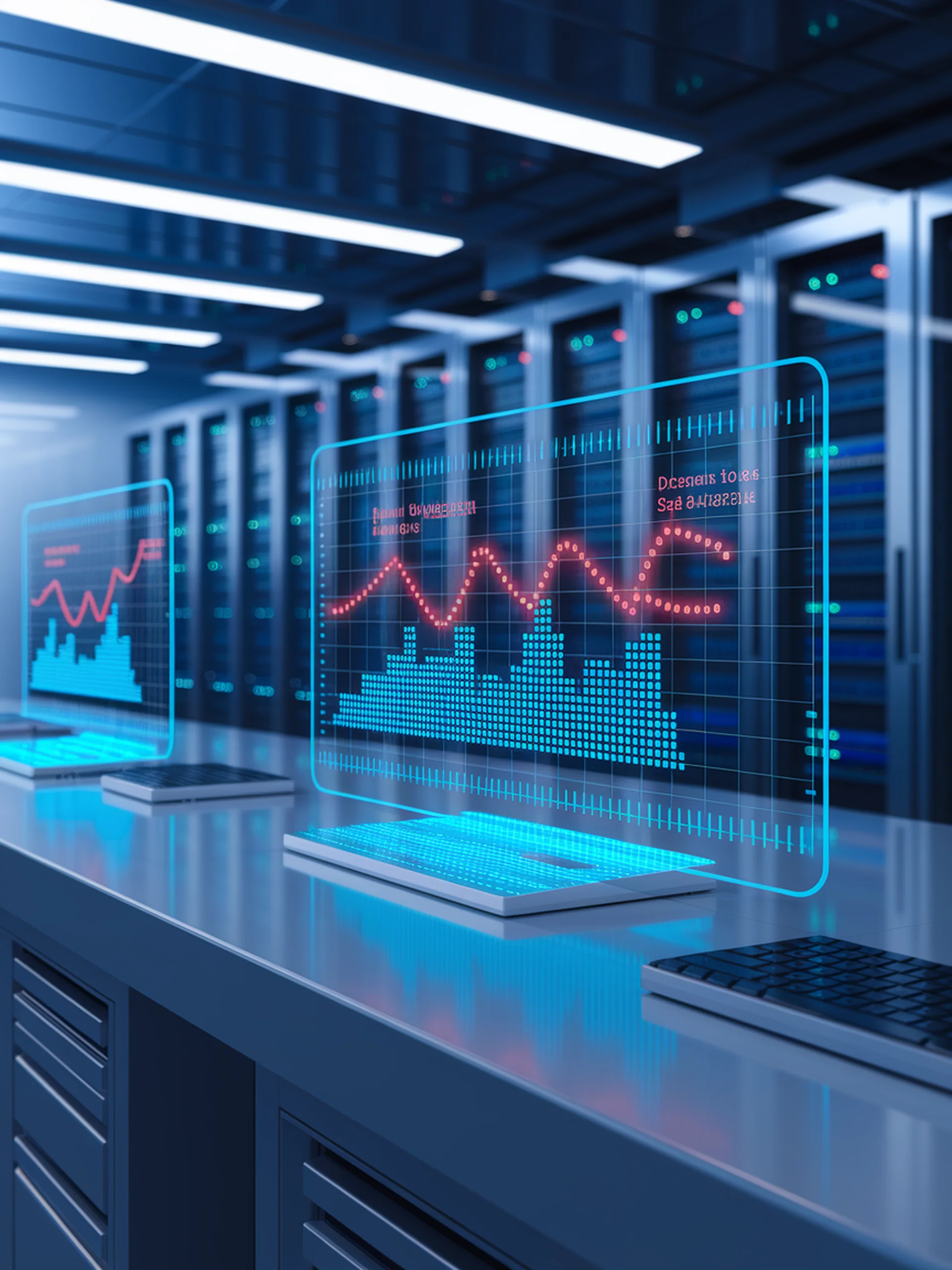
Detecting Benchmark Contamination in LLMs
A new statistical approach to ensure fair model evaluation
PaCoST introduces a novel method to detect when language models have been inadvertently trained on benchmark data, compromising evaluation integrity.
- Identifies benchmark contamination that causes artificially high performance scores
- Employs paired confidence testing to detect statistical anomalies in model responses
- Provides a practical detection framework following key requirements for real-world application
- Helps maintain trustworthy evaluation of AI systems
Why it matters: Benchmark contamination threatens the security and reliability of AI evaluation, potentially hiding model limitations and creating false confidence in capabilities that don't transfer to real-world applications.