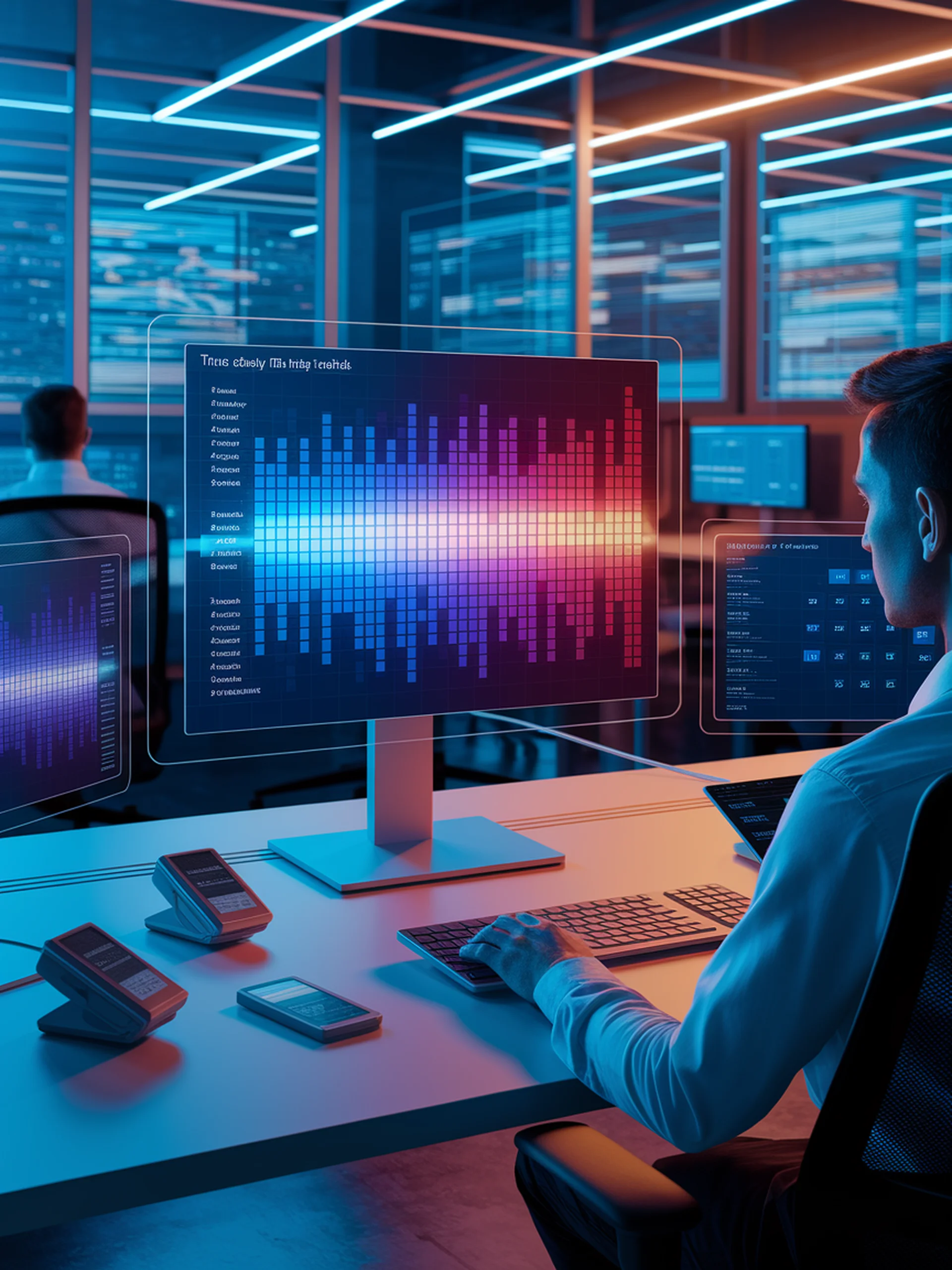
Adaptive Guardrails for LLMs
Trust-based security frameworks for diverse user needs
This research introduces adaptive guardrails for large language models that dynamically adjust content filtering based on user trust levels.
- Implements a sociotechnical approach to LLM safety, balancing protection with access rights
- Utilizes trust modeling to customize guardrail strictness for different user groups
- Enhances security through in-context learning mechanisms that adapt to interactions
- Delivers a flexible framework for managing sensitive content exposure in AI systems
This advancement is critical for security professionals as it enables fine-grained control over LLM outputs without implementing rigid, one-size-fits-all restrictions that hamper legitimate use cases.
Trust-Oriented Adaptive Guardrails for Large Language Models