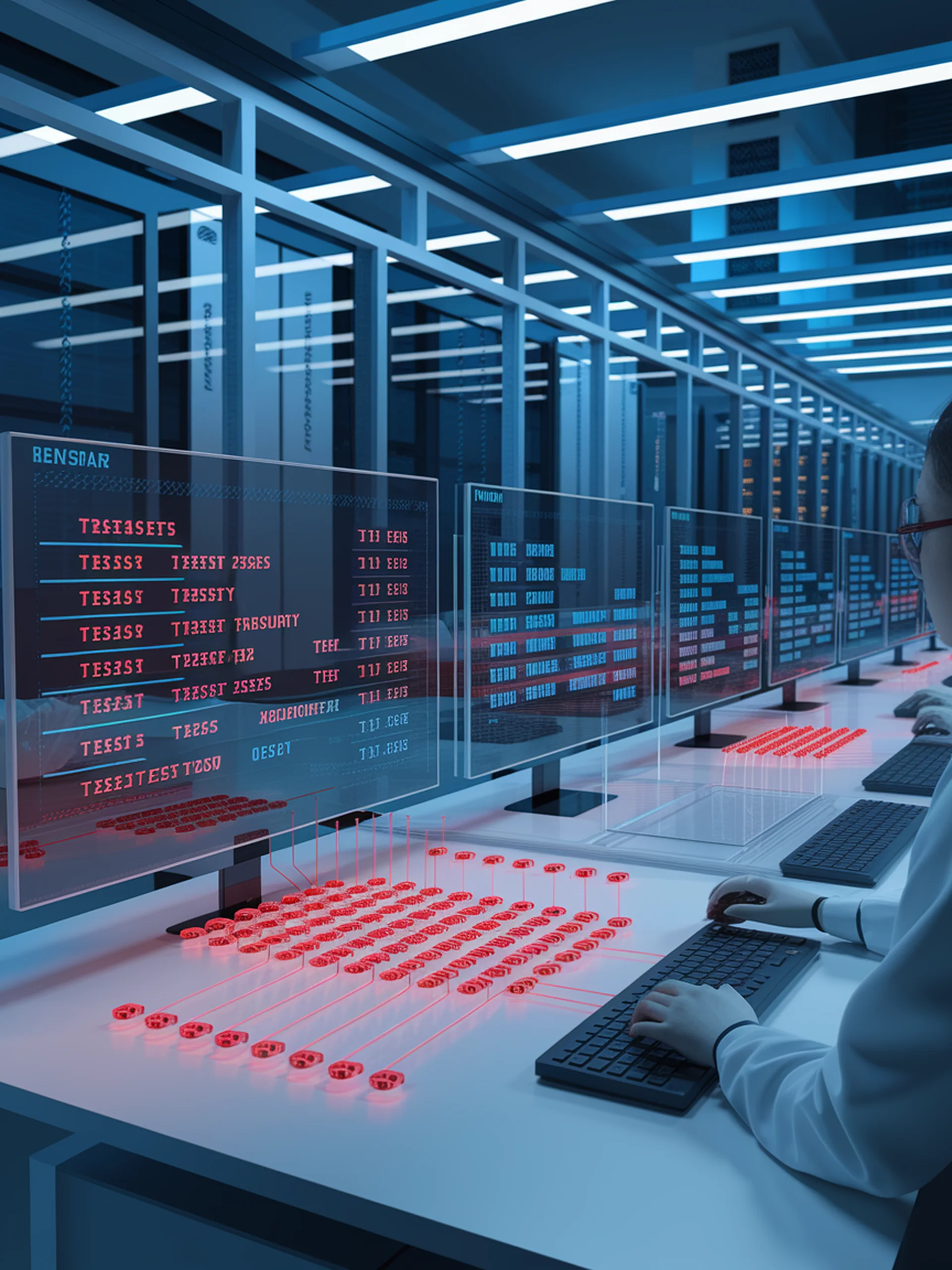
Detecting Benchmark Contamination in LLMs
Protecting evaluation integrity through data leakage detection
This research addresses a critical vulnerability in AI evaluation: benchmark contamination occurs when models are trained on test data, invalidating assessment results.
- Proposes automated methods to detect when LLMs have been exposed to benchmark test data
- Demonstrates how benchmark contamination undermines reliable model performance evaluation
- Establishes protocols to protect the integrity of AI benchmarking systems
- Highlights the security implications of data leakage in model training
For security professionals, this work provides essential tools to verify AI evaluation reliability and maintain assessment integrity in an era where pre-training data remains largely opaque.