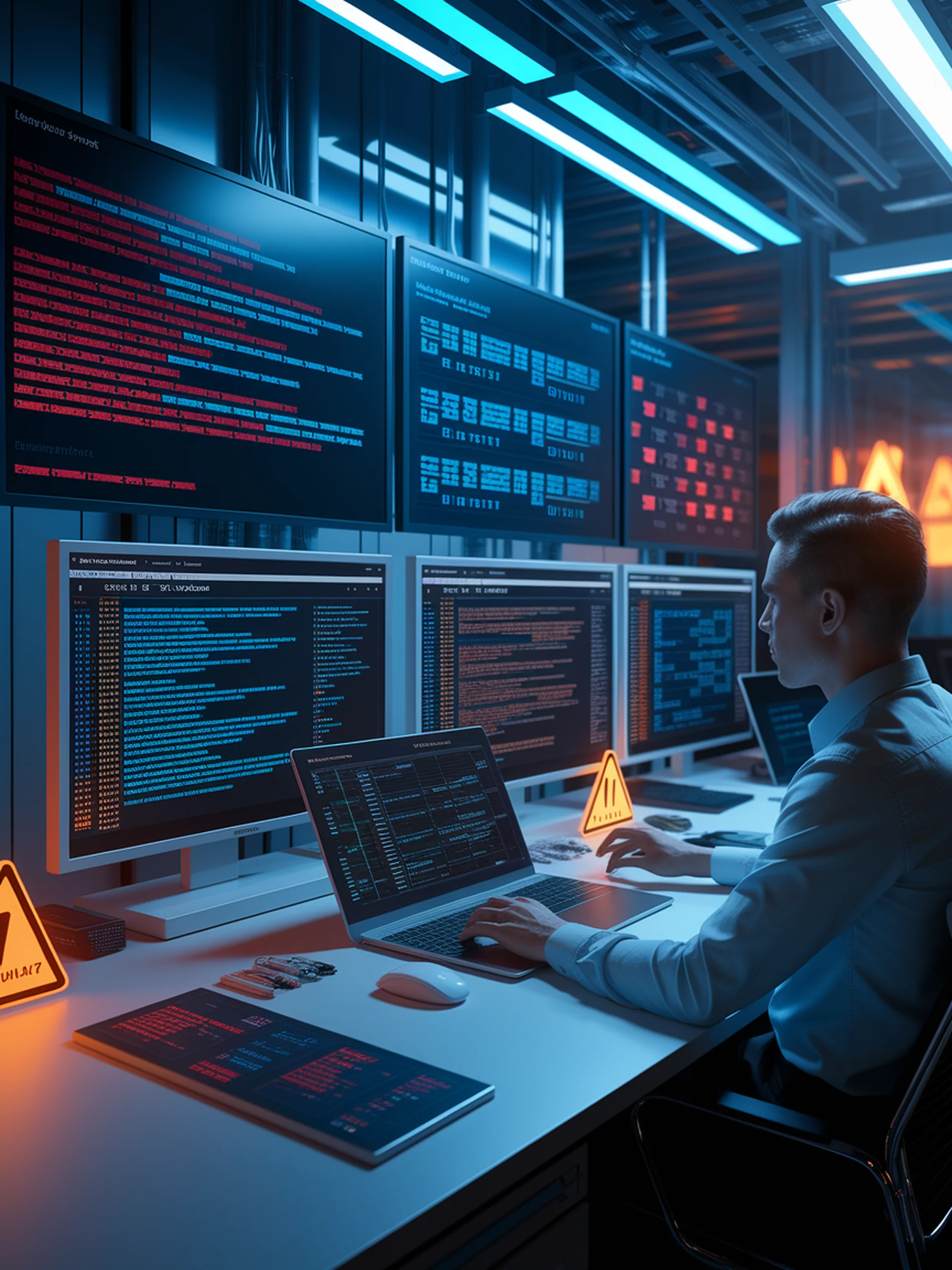
Defending Against LLM Manipulations
How to detect and reverse malicious knowledge edits in LLMs
This research introduces techniques to identify and reverse in-context knowledge edits that could manipulate LLM responses without users' awareness.
- Demonstrates that malicious knowledge edits in LLMs can be effectively detected using length-based indicators and edit reversal prompts
- Provides methods to restore LLMs to their original behavior after being manipulated
- Shows that detection methods work across multiple LLM types (including GPT-4 and Llama 2)
For security professionals, this research is crucial as it addresses vulnerabilities in AI systems that could be exploited to spread misinformation or offensive content through seemingly trustworthy interfaces.
How to Make LLMs Forget: On Reversing In-Context Knowledge Edits