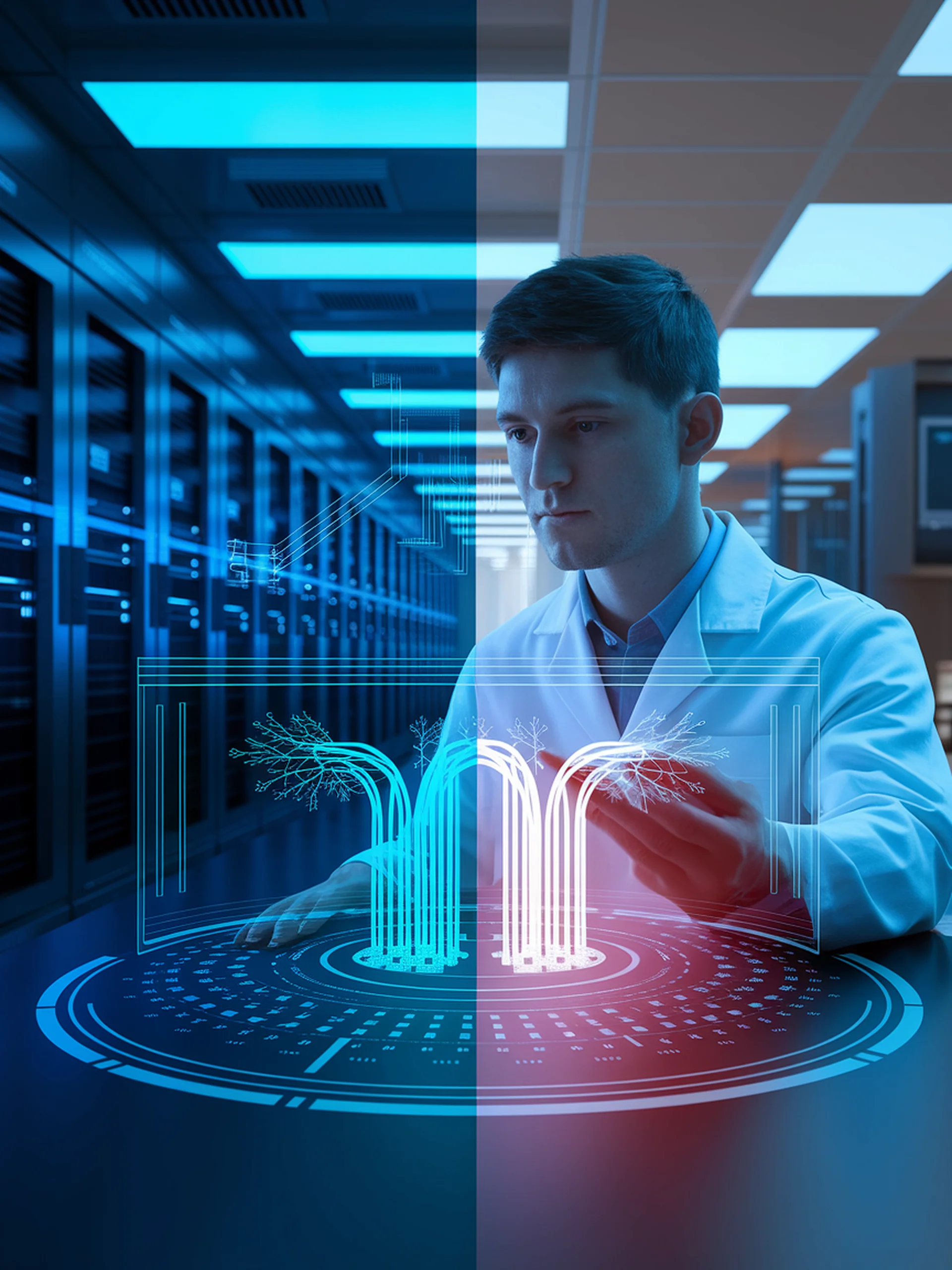
Making AI Reward Models Transparent
Enhancing trust in LLMs through contrastive explanations
This research proposes a novel approach to explain AI reward models that guide large language models, making AI alignment more transparent and trustworthy.
- Uses contrastive explanations to reveal why reward models prefer certain responses
- Enables better understanding of AI decision-making processes
- Addresses critical security concerns by making reward models less of a "black box"
- Supports more reliable AI alignment with human values
By improving transparency in how LLMs are evaluated and guided, this research helps organizations deploy AI systems that are more accountable and aligned with intended use cases, reducing potential security and trust issues.
Interpreting Language Reward Models via Contrastive Explanations