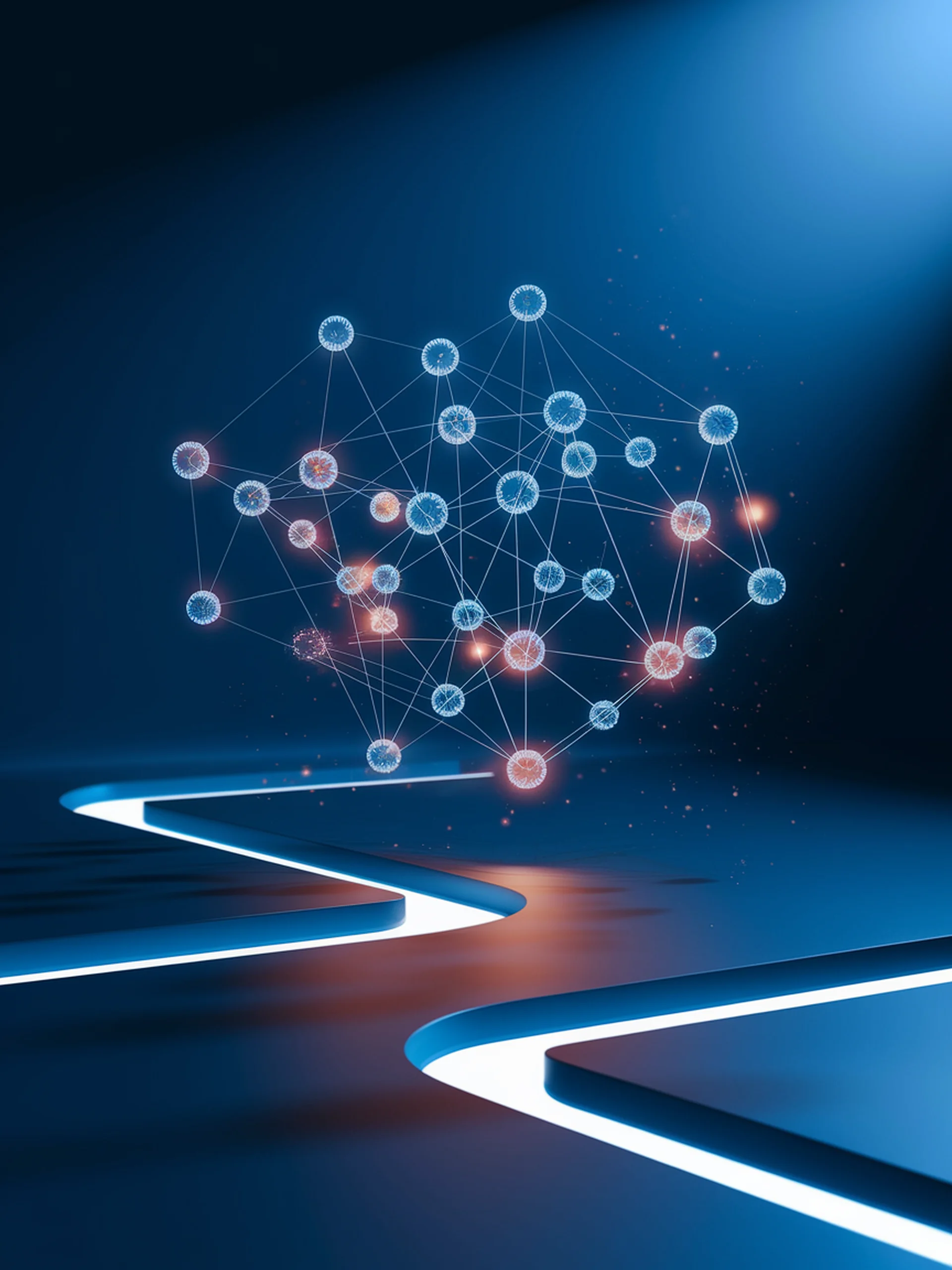
Detecting LLM Hallucinations with Semantic Graphs
An innovative approach to uncertainty modeling that improves hallucination detection
This research proposes a novel semantic graph-based uncertainty modeling technique to detect hallucinations in large language models without relying on external knowledge or expensive sampling.
- Integrates semantic relationships between tokens rather than evaluating each token's uncertainty in isolation
- Constructs a semantic graph where nodes represent tokens and edges capture their contextual relationships
- Achieves superior detection performance compared to existing uncertainty-based methods
- Provides a more efficient and accurate way to identify potentially fabricated or non-factual information in LLM outputs
For security applications, this method enhances trustworthiness of LLM-powered systems by identifying unreliable content before it reaches users, reducing risks in critical domains like healthcare, finance, and legal applications.
Enhancing Uncertainty Modeling with Semantic Graph for Hallucination Detection