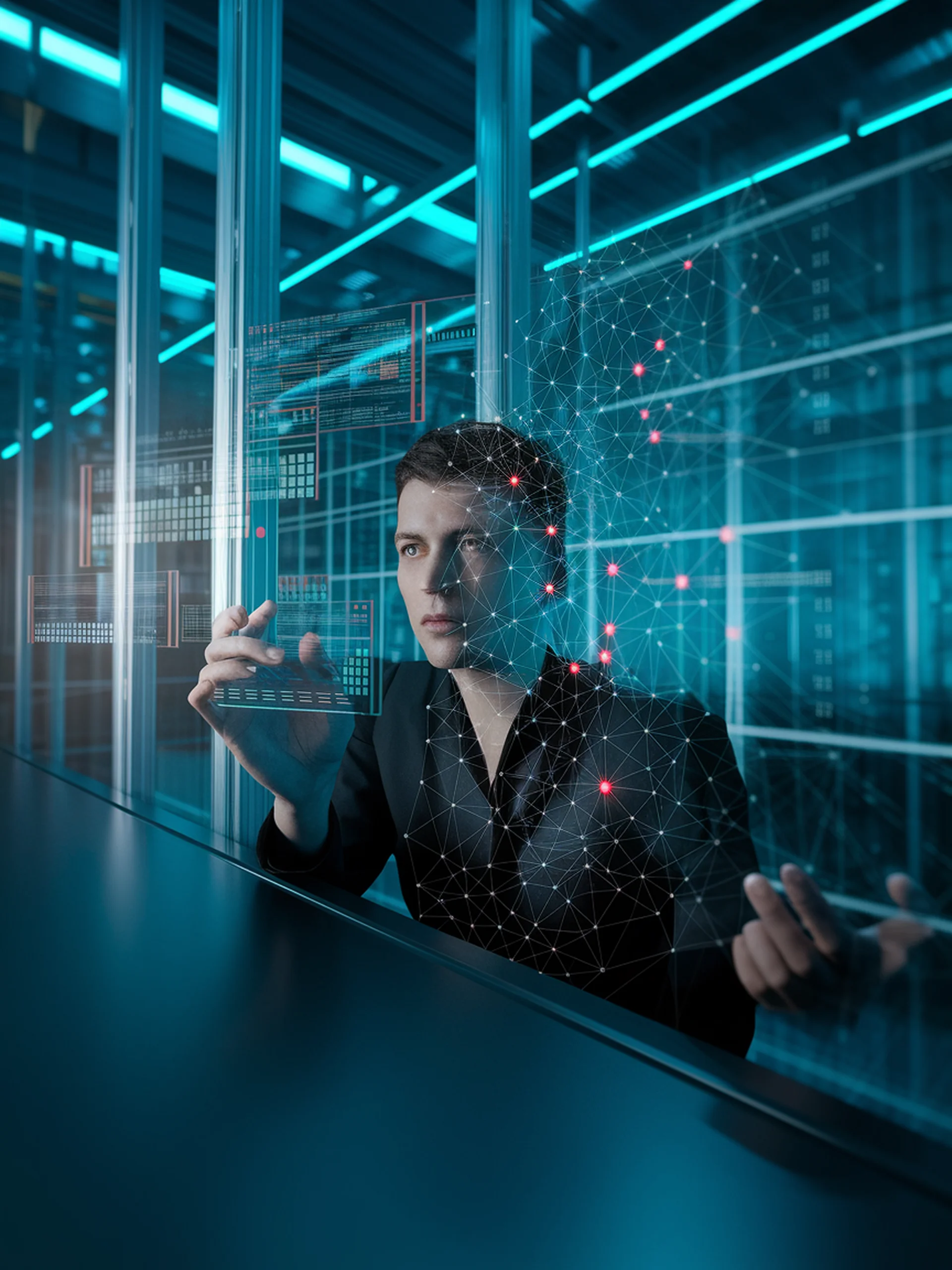
Enhancing LLM Security Through Knowledge Boundary Perception
Using internal states to prevent confident yet incorrect responses
This research explores how to leverage internal states within Large Language Models to help them recognize when they're operating outside their knowledge boundaries.
- Introduces Consistency-based Confidence Calibration to improve LLM reliability
- Enables LLMs to estimate confidence before generating a full response
- Significantly reduces the risk of delivering incorrect information with high confidence
- Improves efficiency by potentially avoiding unnecessary computations
For security professionals, this approach offers a crucial advancement in making LLMs more trustworthy by helping models recognize and indicate when they lack sufficient knowledge—reducing the risk of harmful or misleading outputs in critical applications.
Towards Fully Exploiting LLM Internal States to Enhance Knowledge Boundary Perception