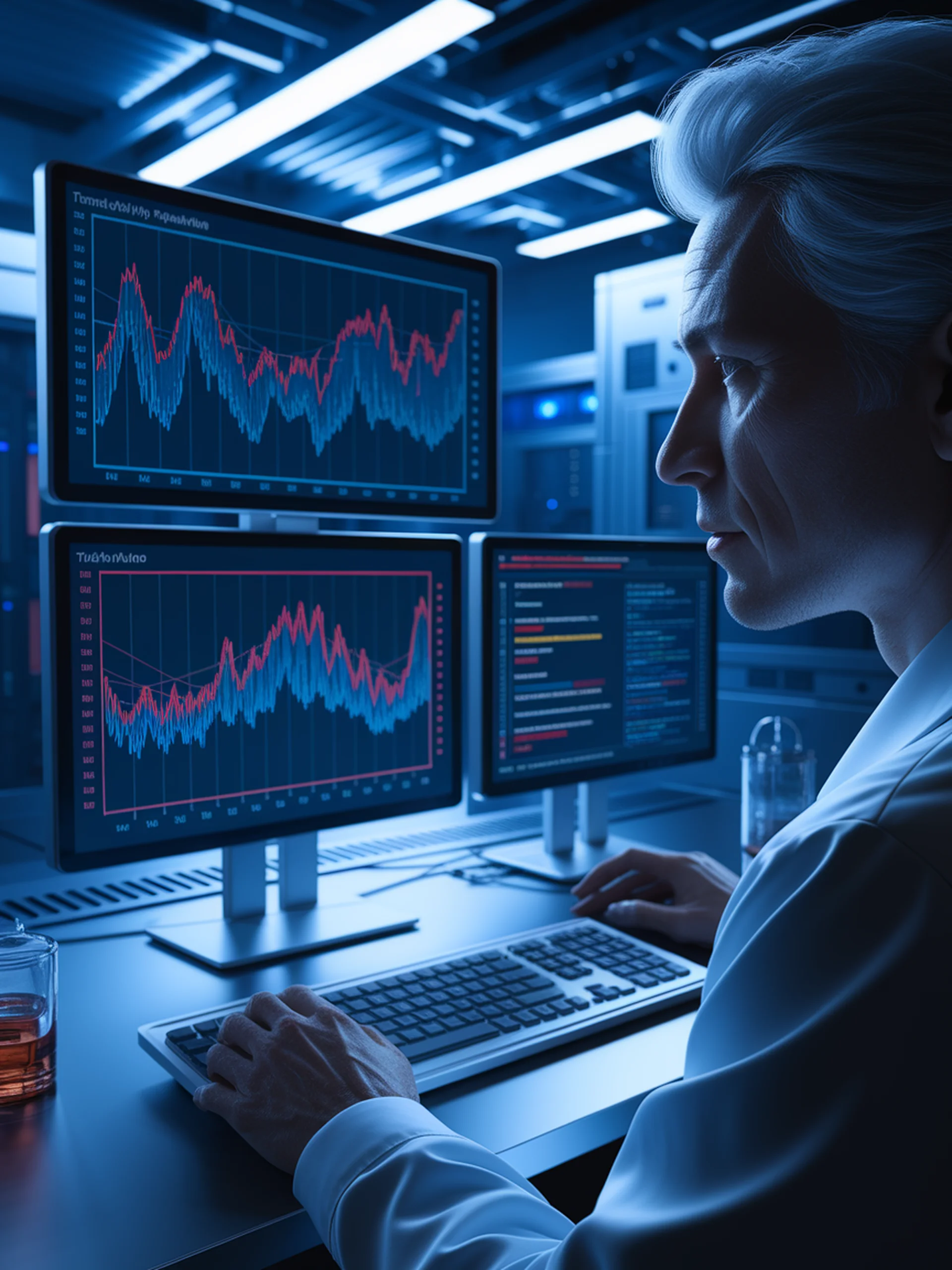
Making LLMs Safer Through Better Uncertainty Estimation
A more robust approach to measuring AI confidence
This research introduces Monte Carlo Temperature - a novel sampling strategy that improves how we measure uncertainty in large language models.
Key findings:
- Traditional uncertainty quantification methods are highly sensitive to temperature selection
- The new Monte Carlo Temperature approach samples across multiple temperatures, providing more robust uncertainty estimates
- This method significantly outperforms fixed-temperature approaches in critical applications
- Enables more reliable risk assessment when deploying LLMs in high-stakes environments
Security Implications: By providing more accurate uncertainty estimates, this research helps prevent overconfident but incorrect AI outputs in sensitive applications like healthcare, finance, and security - directly addressing a critical safety concern for enterprise LLM deployment.
Monte Carlo Temperature: a robust sampling strategy for LLM's uncertainty quantification methods