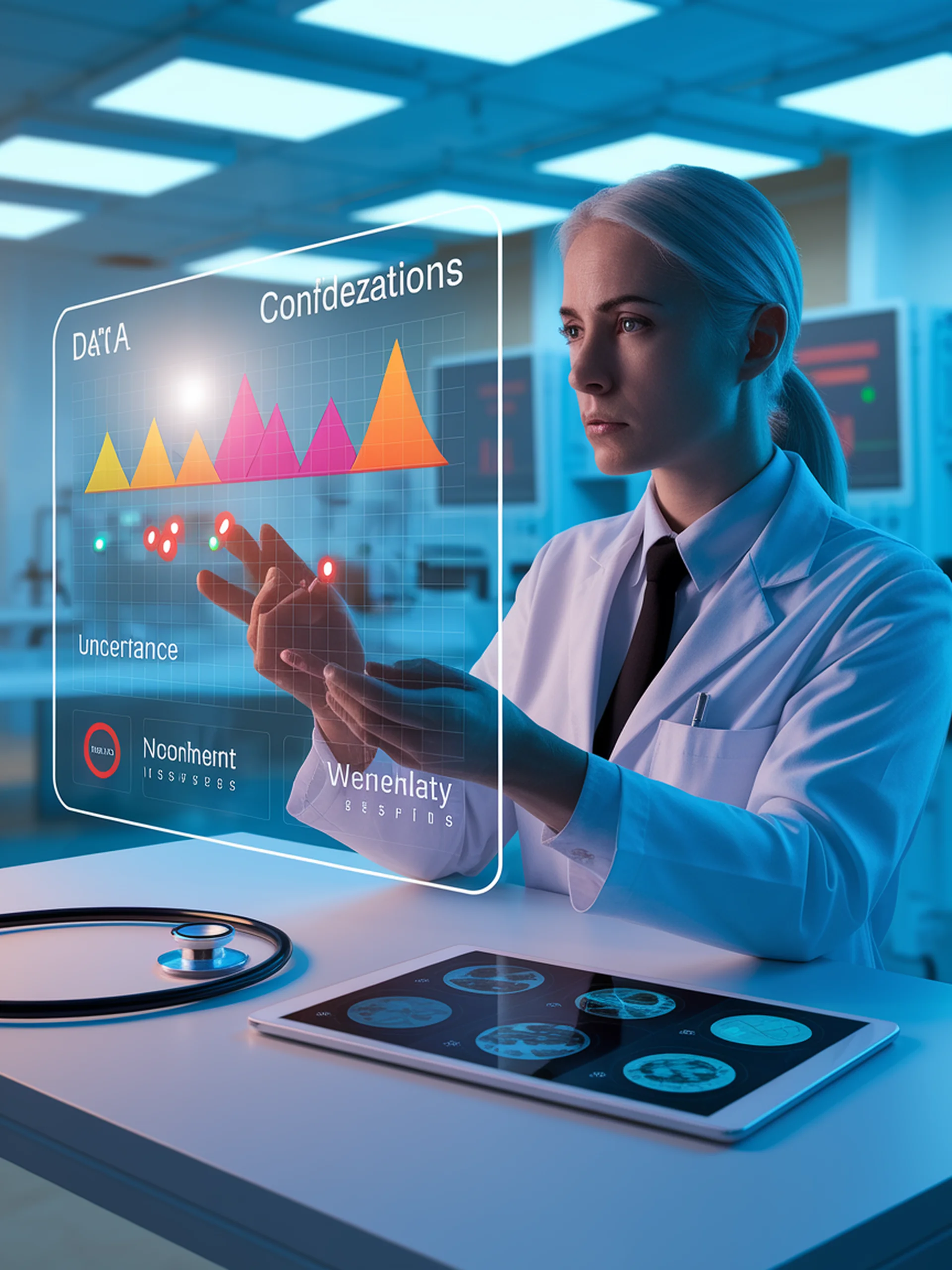
Trustworthy AI: The Confidence Challenge
Improving reliability of LLMs in high-stakes domains
This research explores uncertainty quantification (UQ) methods to enhance trustworthiness of large language models when deployed in critical applications.
- LLMs often produce plausible but incorrect responses in high-stakes domains
- Uncertainty quantification estimates confidence in outputs, enabling risk mitigation
- Traditional UQ methods face significant challenges when applied to modern LLMs
- Research surveys approaches to achieve reliable confidence calibration in LLM responses
Medical Impact: For healthcare applications, properly calibrated confidence measures are crucial for clinical decision support, reducing the risk of harmful outcomes, and establishing appropriate trust levels among medical professionals.
Uncertainty Quantification and Confidence Calibration in Large Language Models: A Survey