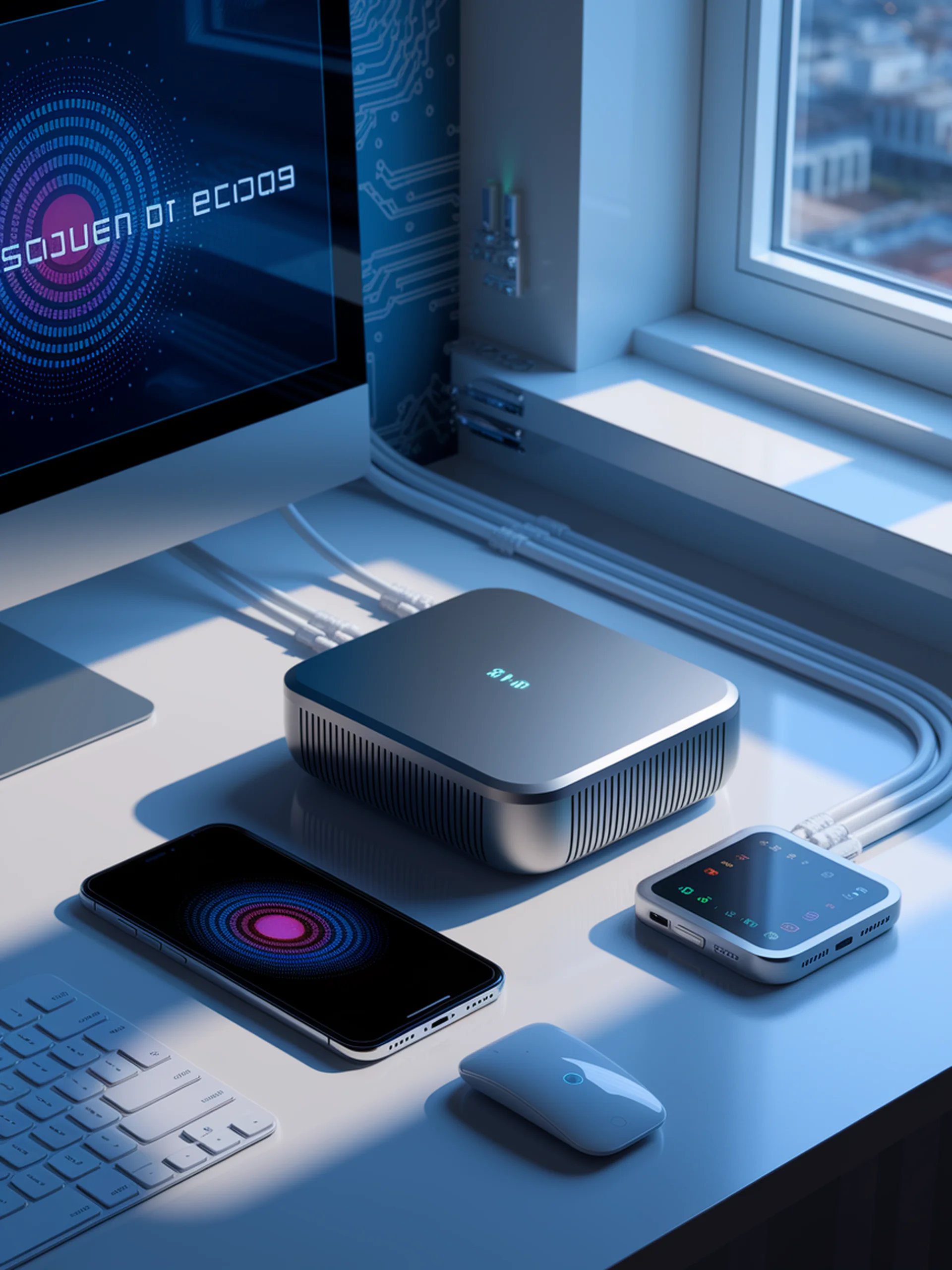
Detecting AI Hallucinations on Edge Devices
A lightweight entropy-based framework for resource-constrained environments
ShED-HD introduces a novel lightweight framework for detecting hallucinations in Large Language Models without requiring additional computational resources.
- Leverages Shannon entropy distributions to identify factual inconsistencies in LLM outputs
- Achieves comparable accuracy to more resource-intensive methods while requiring significantly less computation
- Designed specifically for edge devices with limited processing power
- Enables real-time hallucination detection for security-critical applications
Security Impact: This research addresses a critical vulnerability in AI deployment by enabling trustworthy LLM use in high-stakes domains where factual accuracy is essential, without requiring cloud connectivity or substantial computing resources.