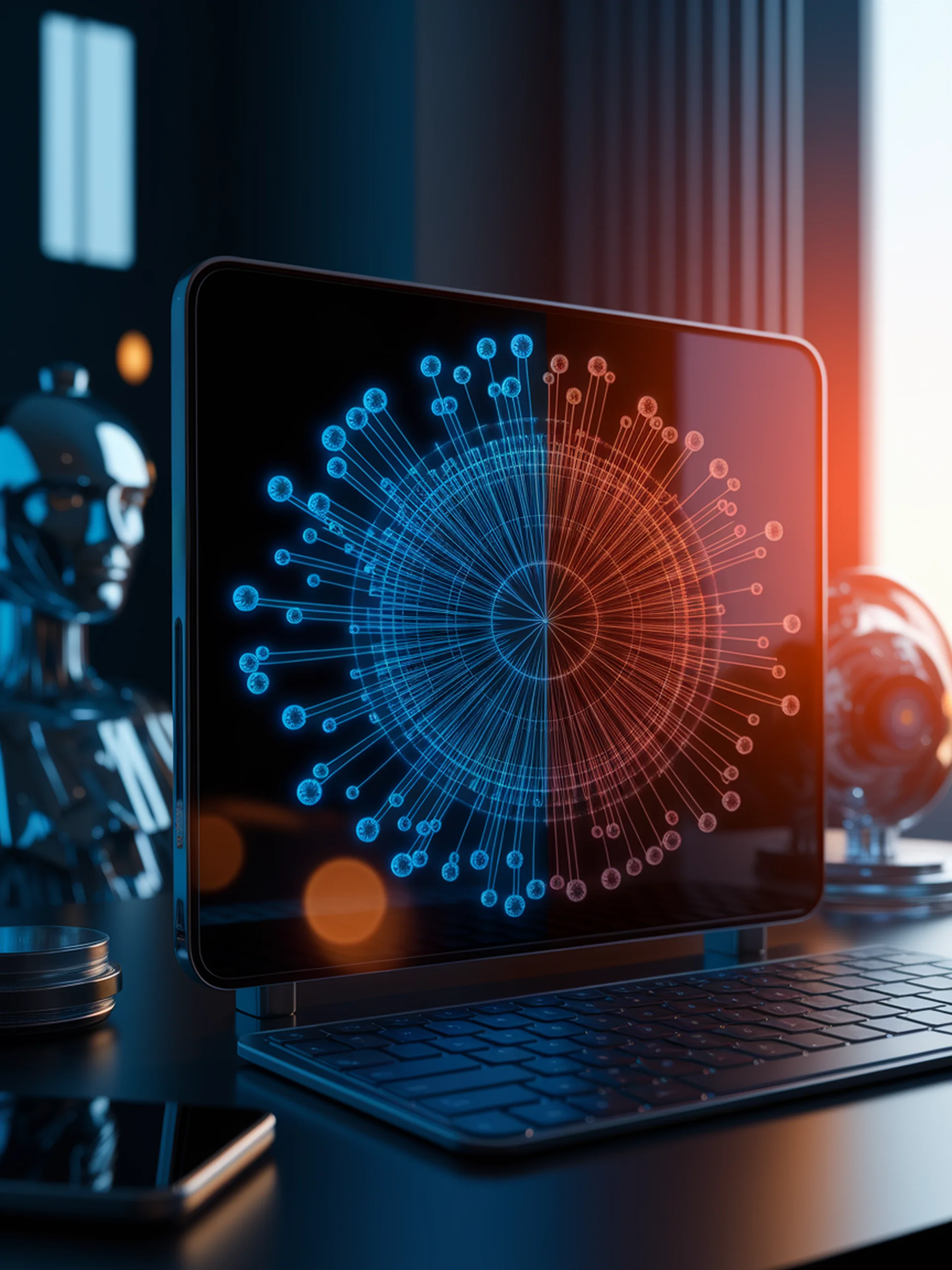
The Safety-Capability Dilemma in LLMs
Understanding the inevitable trade-offs in fine-tuning language models
This research establishes a theoretical framework explaining why enhancing an LLM's capabilities through fine-tuning often compromises its safety guardrails.
- Analyzes two primary safety-aware fine-tuning strategies and their fundamental limitations
- Demonstrates that safety-capability trade-offs are inherent to current fine-tuning approaches
- Provides mathematical foundations for understanding the tension between performance and safety
- Offers insights for developing more balanced fine-tuning methods
For security professionals, this work illuminates why seemingly well-trained models can unexpectedly generate harmful outputs, helping organizations better assess risks when deploying fine-tuned LLMs in production environments.
Fundamental Safety-Capability Trade-offs in Fine-tuning Large Language Models