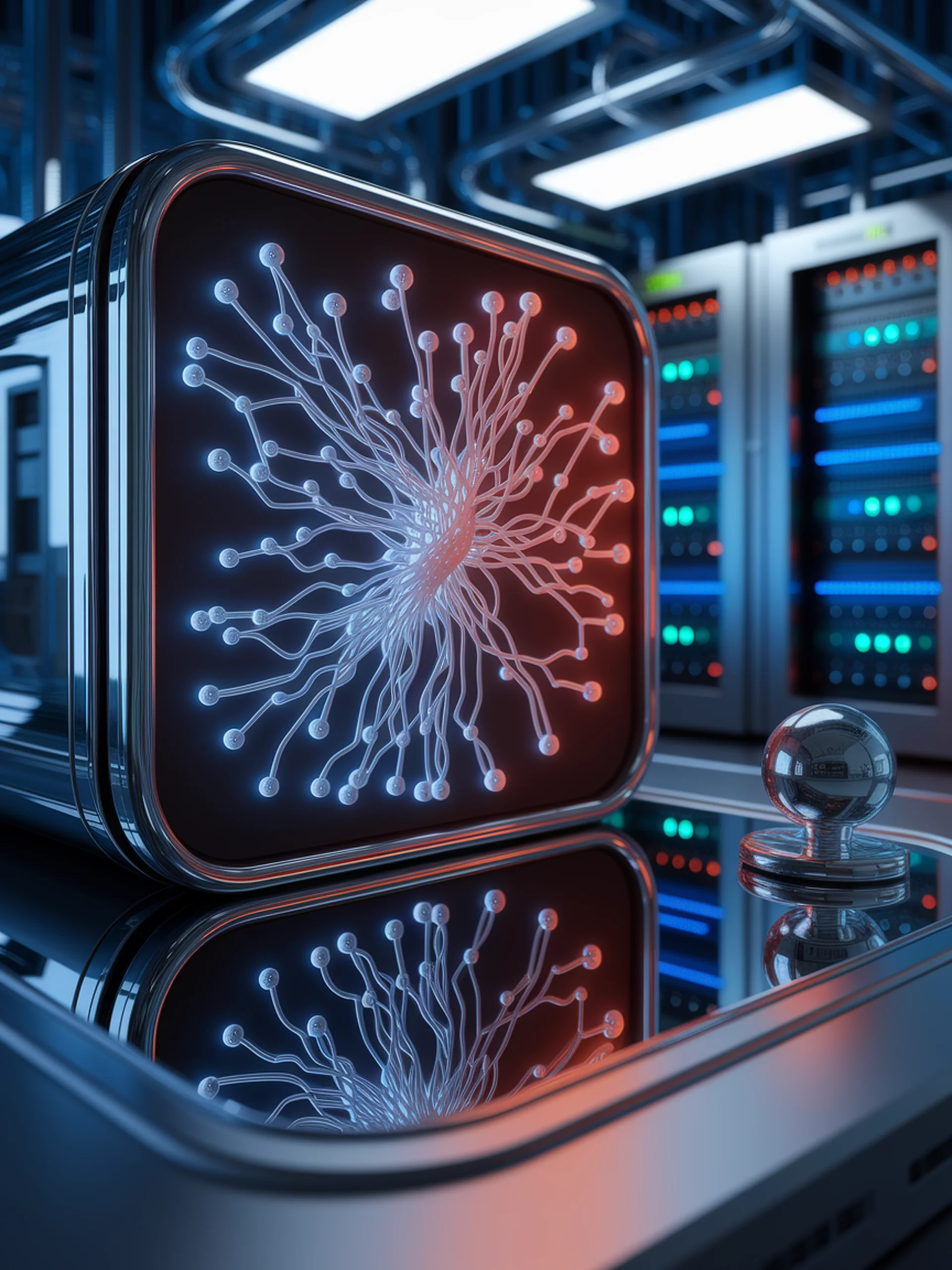
Preserving Safety in Compressed LLMs
Using Mechanistic Interpretability to Improve Refusal Behaviors
This research identifies a specific direction in model activation space responsible for refusal behaviors in compressed language models, offering novel methods to preserve safety without performance loss.
- Discovered a key refusal direction in model activation space that enables better understanding of safety mechanisms
- Demonstrated that compressed models experience a significant drop in refusal capabilities while maintaining other performance aspects
- Developed a targeted intervention technique that enhances refusal behaviors without degrading general model performance
- Established a new approach for safety alignment preservation during the model compression process
This research advances security by providing concrete mechanisms to maintain trustworthiness in smaller, more deployable language models - critical for widespread, safe AI adoption in business and public sectors.
Towards Understanding and Improving Refusal in Compressed Models via Mechanistic Interpretability