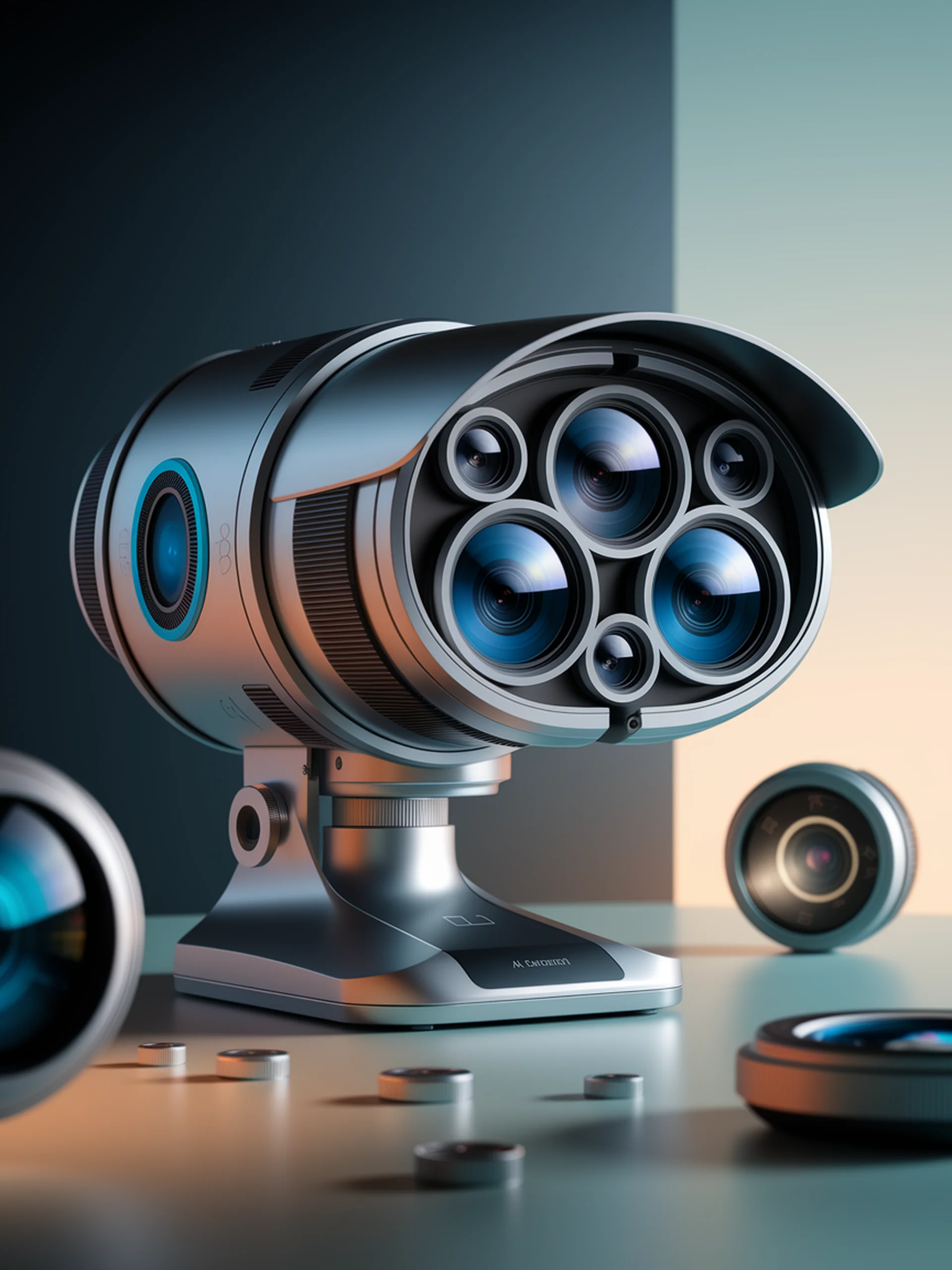
Eagle: Advancing Visual Understanding in AI
Optimizing multimodal LLMs through mixture of vision encoders
Eagle explores how to significantly enhance the visual perception capabilities of large language models by systematically analyzing the design choices for combining multiple vision encoders in multimodal systems.
- Reduces hallucinations and improves performance on resolution-sensitive tasks like optical character recognition
- Provides the first systematic comparison of different vision encoder combinations to identify optimal architectures
- Demonstrates how specialized encoders working together can outperform single-encoder approaches across diverse visual understanding tasks
For creators and designers, this research enables AI systems that more accurately interpret visual content, recognize text in images, and understand complex visual compositions—opening new possibilities for creative tools with deeper visual comprehension.
Eagle: Exploring The Design Space for Multimodal LLMs with Mixture of Encoders