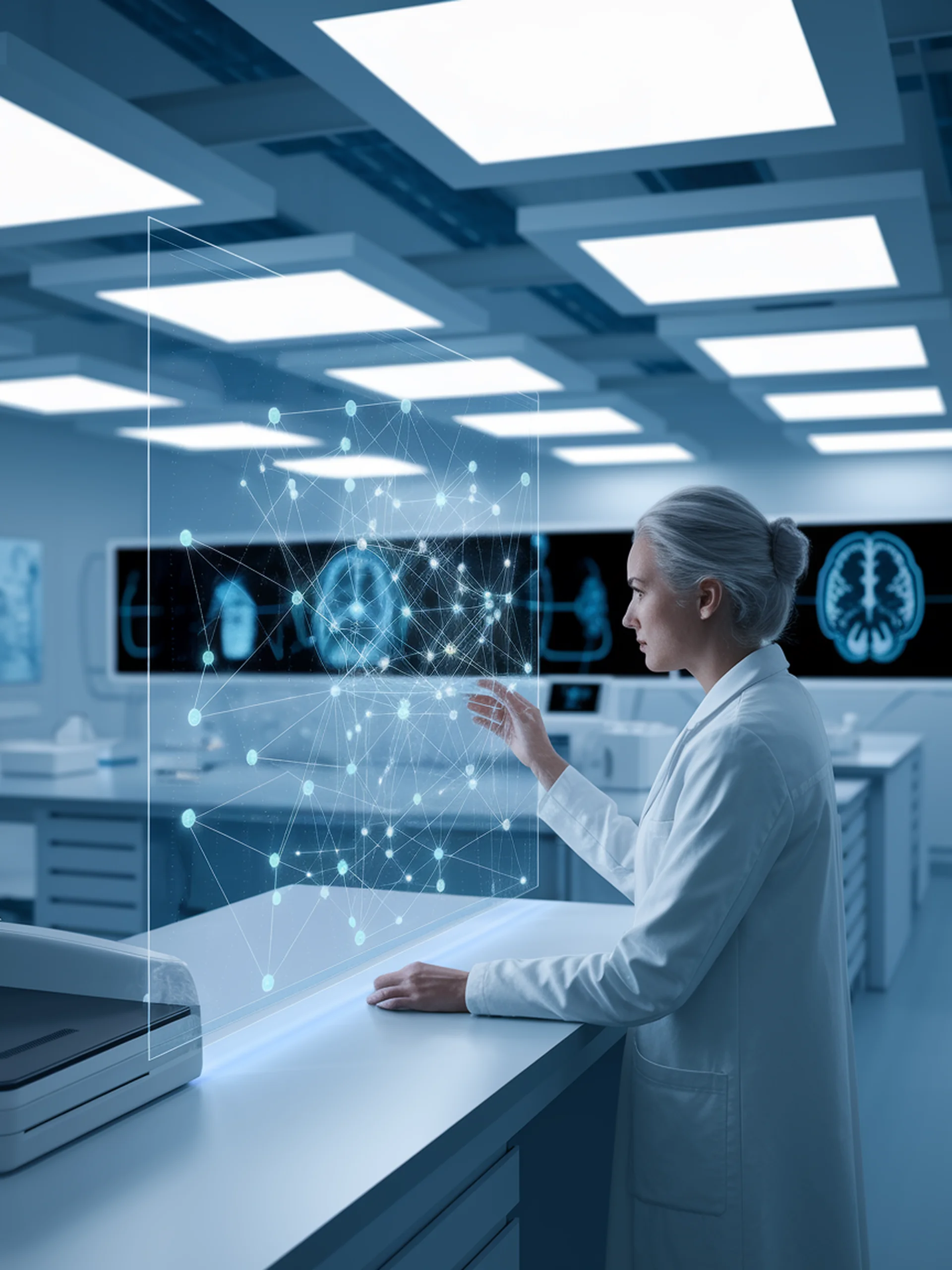
Enhancing LLMs for Causal Reasoning
Evaluating how large language models understand causal relationships in graphs
This research explores how well Large Language Models (LLMs) can understand and reason with causal graphs - a critical capability for scientific problem-solving.
- LLMs are evaluated on their ability to encode causal relationships and answer questions about variables' interactions
- The study introduces novel benchmarks for testing causal reasoning capabilities in language models
- Results reveal both strengths and limitations in how current LLMs process causal information
Medical Relevance: Understanding causal relationships is fundamental in medical research for establishing connections between treatments and outcomes. This research helps evaluate whether AI systems can assist medical professionals in analyzing complex causal networks and potentially discovering new relationships between variables.