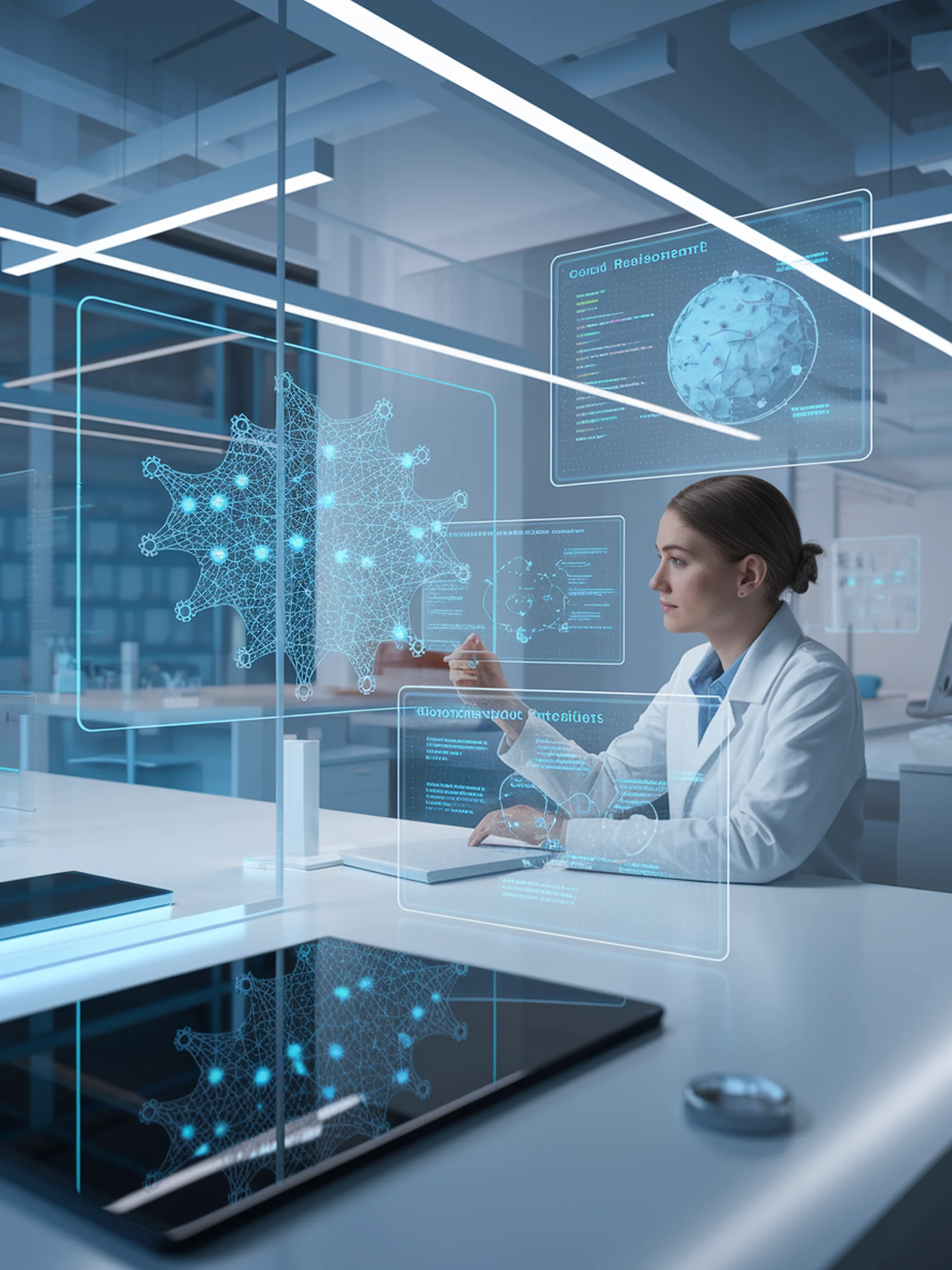
Harnessing LLMs for Better Causal Discovery
Enhancing reliability in causal relationship identification
This research introduces a novel approach that leverages Large Language Models to identify causally consistent relationships while mitigating hallucinations and contradictions.
- Combines LLM capabilities with algorithmic constraints to ensure maximally consistent causal orders
- Develops a two-step methodology: extraction of causal pairs from text followed by mathematical verification
- Demonstrates superior performance compared to traditional causal discovery methods on real-world health datasets
- Addresses fundamental LLM reliability issues through formal consistency verification
For medical applications, this approach enables more reliable extraction of causal relationships from clinical literature and health records, supporting evidence-based decision-making while reducing the risk of contradictory causal conclusions.
Original Paper: Discovery of Maximally Consistent Causal Orders with Large Language Models