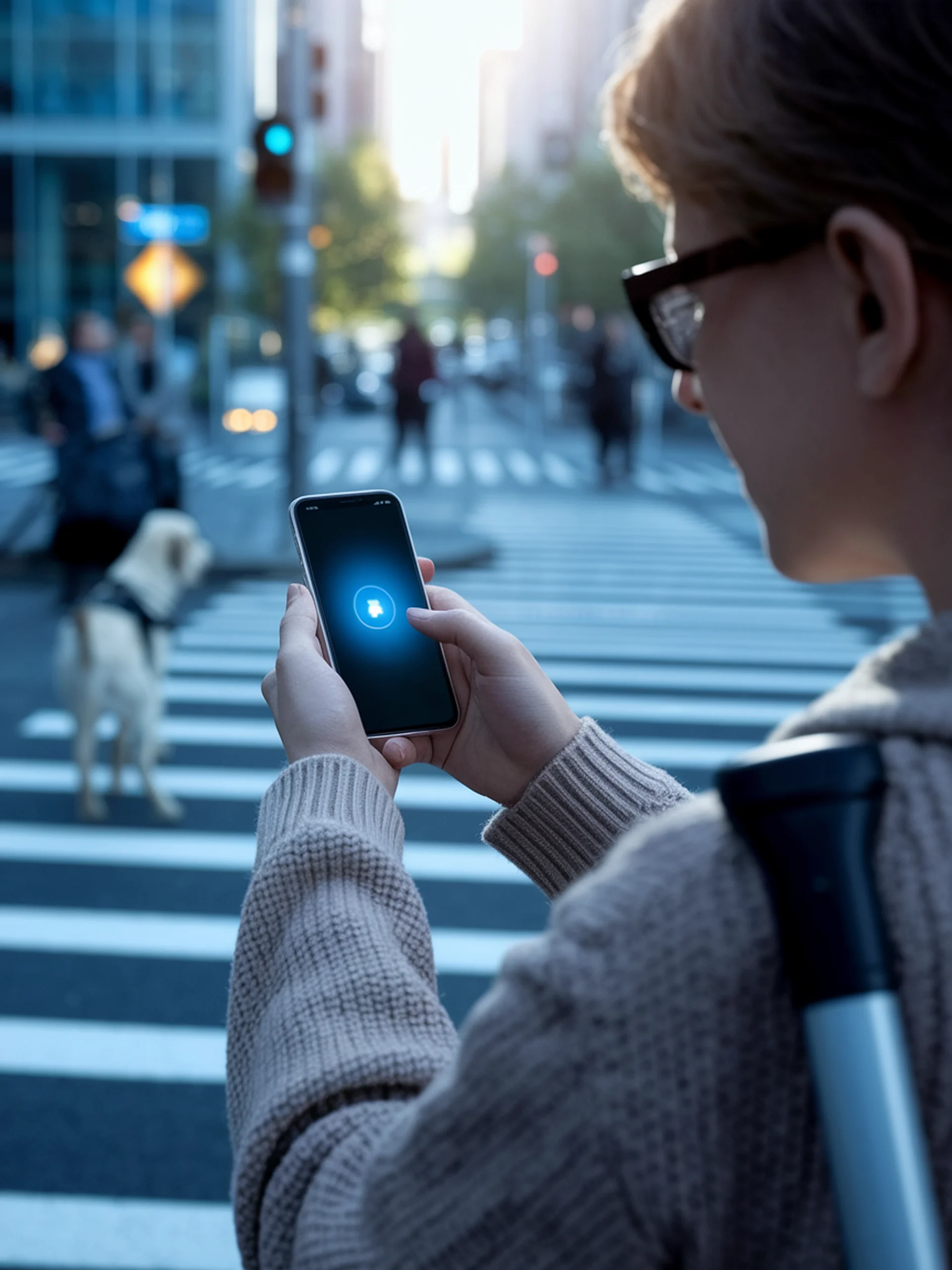
Optimizing AI Navigation Aids for the Visually Impaired
Understanding BLV User Preferences in LVLM Responses
This research evaluates how well Large Vision-Language Models (LVLMs) serve as navigational aids for Blind and Low-Vision (BLV) individuals, comparing automated metrics with actual user preferences.
Key Findings:
- LVLMs provide promising potential as navigational assistance tools for BLV users
- Standard automatic evaluation metrics do not fully align with BLV user preferences
- BLV users have specific preferences for response types and styles that must be considered
- Research highlights the need for specialized evaluation frameworks focused on real user needs
Medical Impact: This work bridges critical gaps in assistive healthcare technology by ensuring AI navigation systems actually serve the specific needs of visually impaired individuals, potentially improving independence and quality of life for millions worldwide.