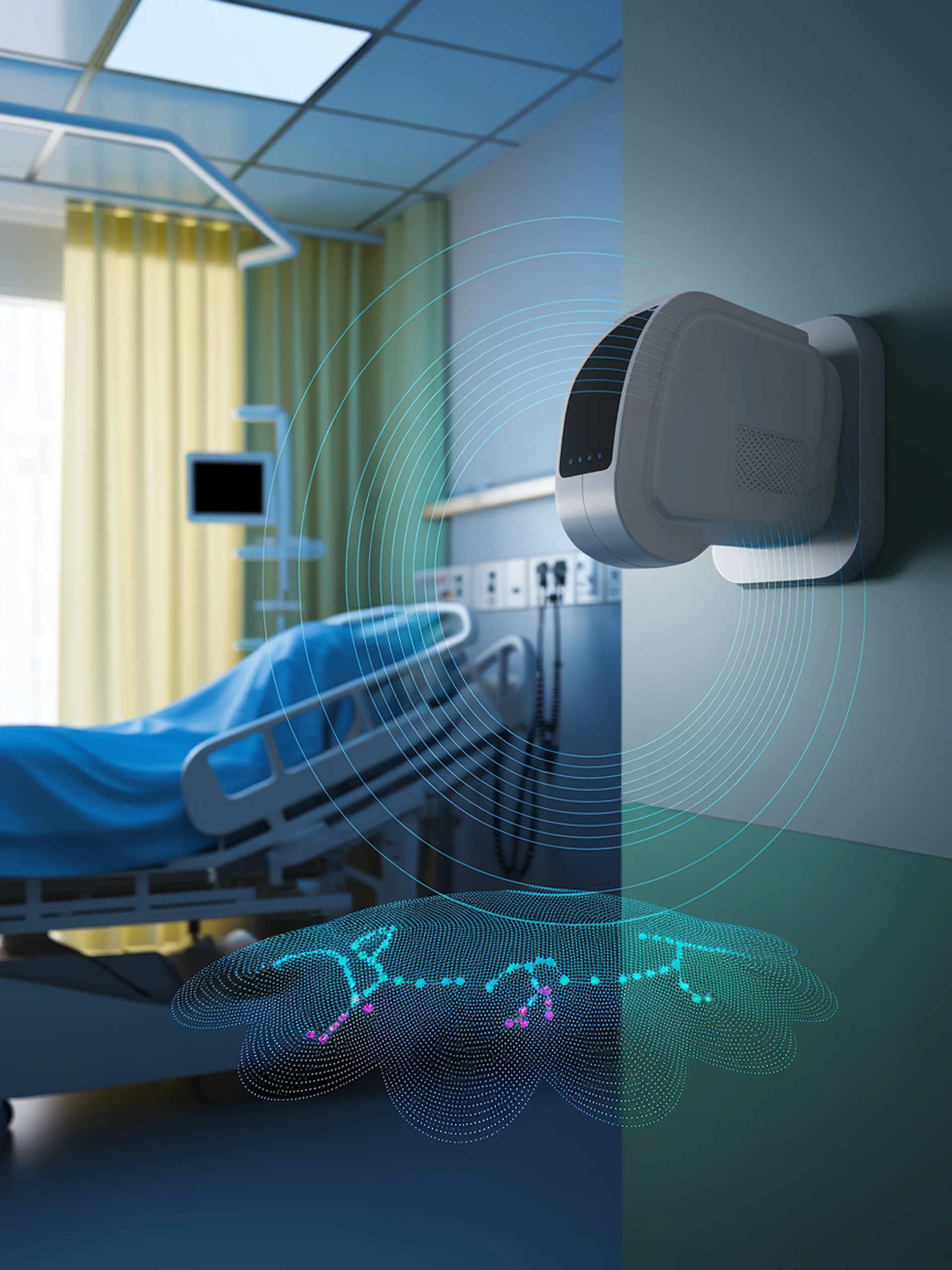
RadarLLM: Privacy-First Motion Understanding
Leveraging Large Language Models for Radar-Based Human Motion Analysis
This research introduces a novel framework that enables LLMs to understand human movement from privacy-preserving millimeter-wave radar data.
- Motion-guided radar tokenizer converts sparse point clouds into language-compatible tokens
- Multimodal LLM architecture bridges the gap between radar signals and semantic understanding
- Privacy-preserving solution for healthcare monitoring without capturing identifiable visual information
- Real-time capabilities with potential applications in patient monitoring and activity recognition
This breakthrough matters for healthcare settings where continuous monitoring is beneficial but privacy concerns limit camera-based solutions, enabling unobtrusive patient activity tracking and fall detection.