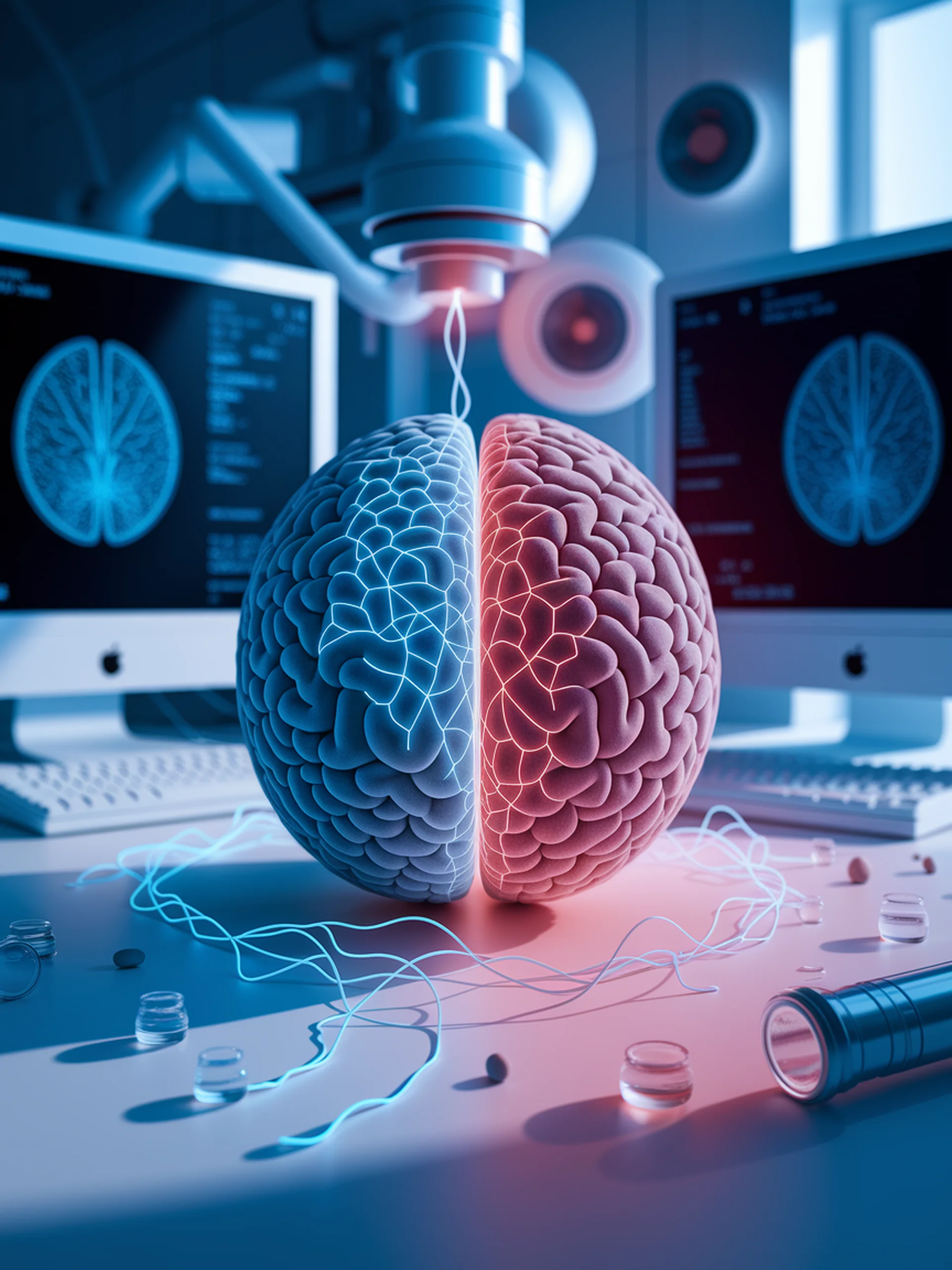
Enhancing Few-Shot Segmentation with LLMs
Bridging the gap between visual features and semantic understanding
DSV-LFS introduces a novel framework that combines large language models with visual features to improve few-shot semantic segmentation (FSS) performance on novel object classes.
- Utilizes LLMs to extract rich semantic cues that complement limited visual features
- Addresses the challenge of incomplete appearance representation in support images
- Achieves more robust generalization across varied domains and object classes
- Demonstrates effectiveness with minimal labeled examples, reducing annotation costs
This research represents a significant advancement in Computer Vision by enhancing models' ability to adapt to new classes without extensive retraining, making segmentation technology more flexible and practical for real-world applications.
DSV-LFS: Unifying LLM-Driven Semantic Cues with Visual Features for Robust Few-Shot Segmentation