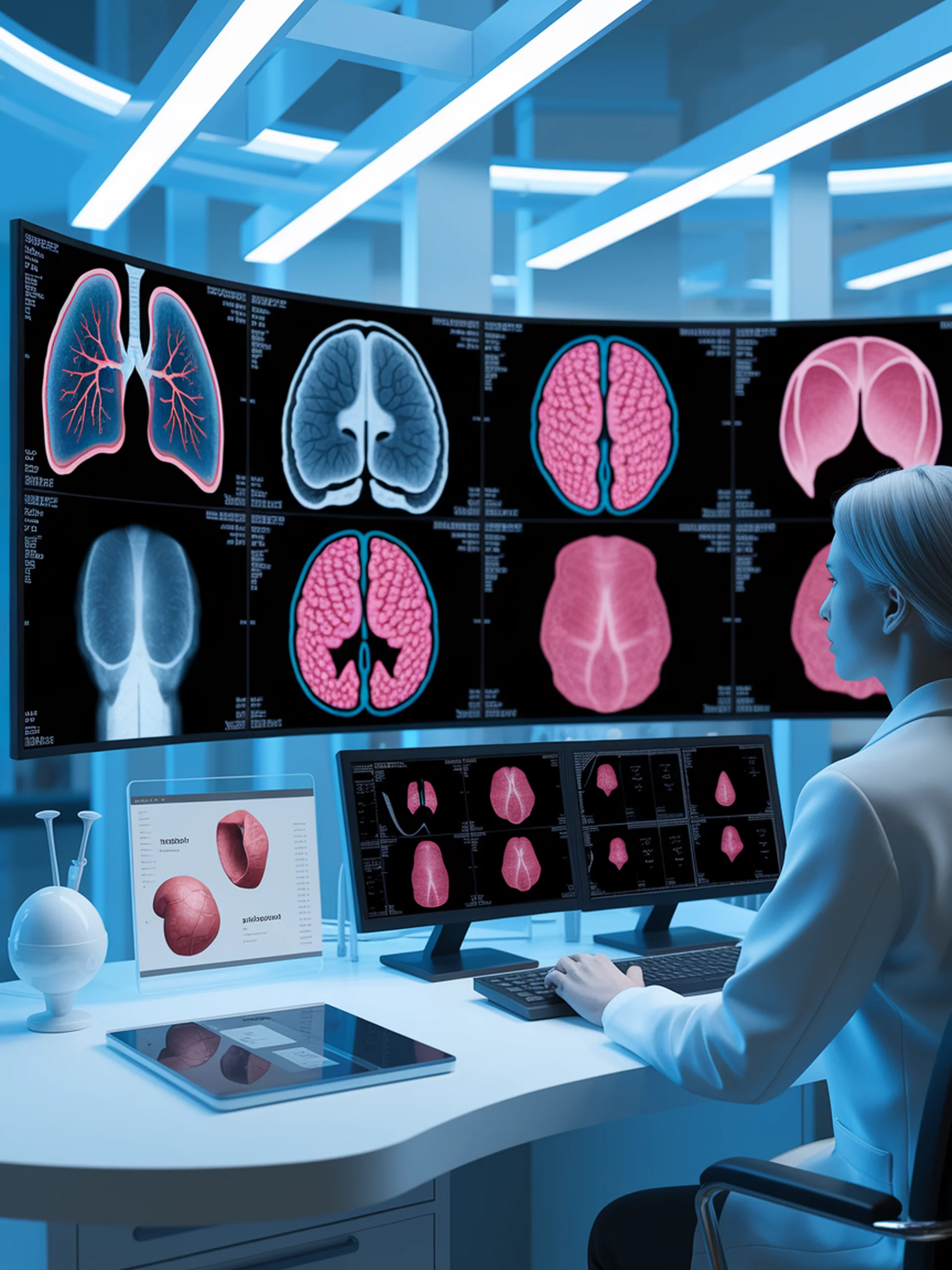
Revolutionizing Medical Image Segmentation
Semi-supervised learning with SAM reduces reliance on expert annotations
This research introduces a novel approach combining the Segment Anything Model (SAM) with preference optimization for medical imaging, dramatically reducing the need for expert annotations.
- Develops an efficient prompting strategy that automatically generates high-quality annotations
- Implements preference optimization that refines segmentation without human intervention
- Achieves superior performance across diverse medical imaging modalities (X-ray, ultrasound, CT)
- Demonstrates effectiveness for lung segmentation, breast tumor detection, and multi-organ segmentation
This advancement matters for healthcare by making accurate medical image segmentation more accessible, reducing costs and expert time requirements while maintaining high diagnostic quality.