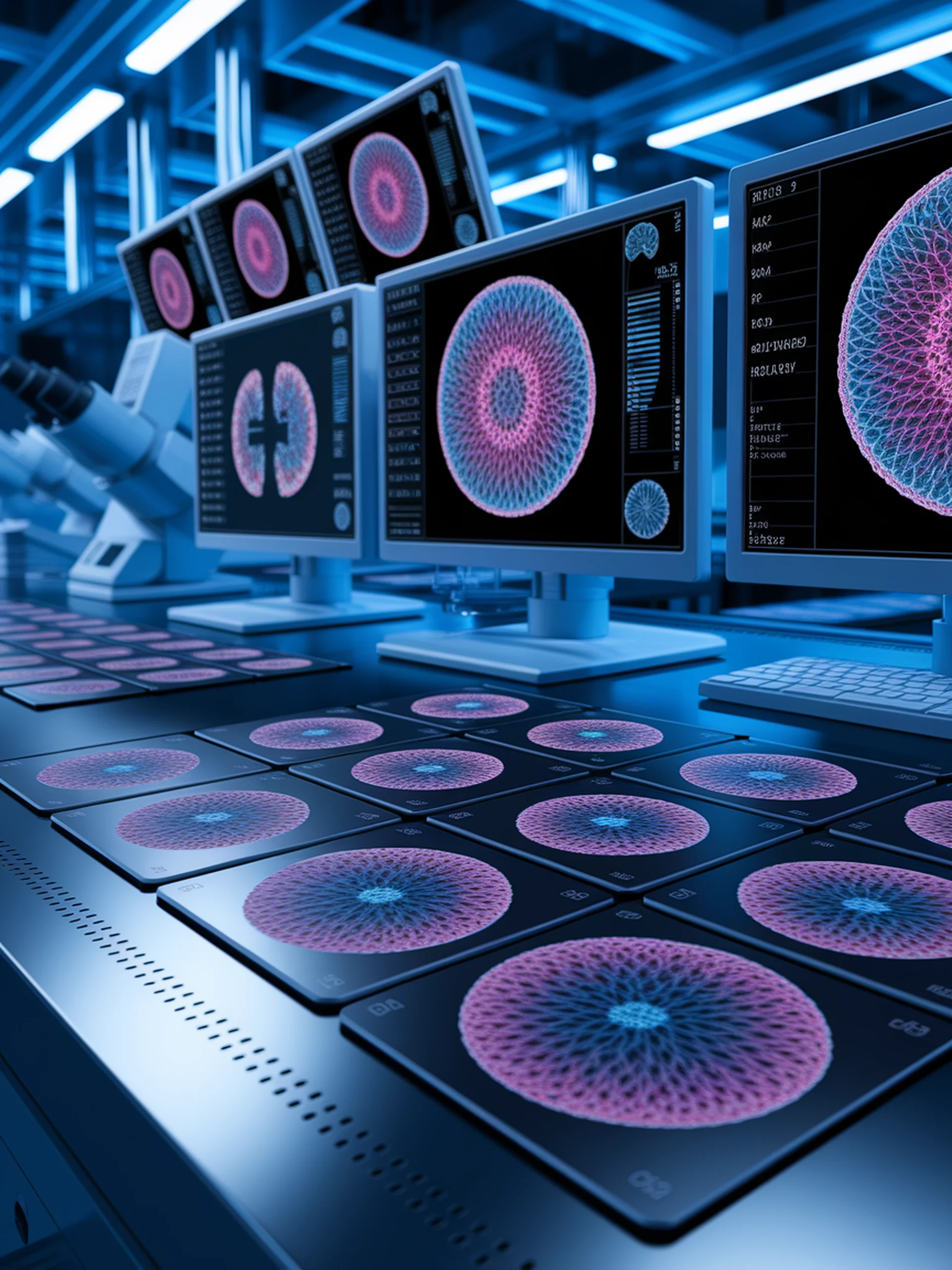
Unsupervised Slide Representation Learning in Pathology
Advancing computational pathology through cross-modal learning
This research introduces a novel unsupervised approach for learning whole slide image (WSI) representations in computational pathology without task-specific supervision.
- Develops Cross-Modal Prototype Allocation (CMPA) that leverages patch-text contrast for effective slide-level representations
- Demonstrates superior generalization compared to traditional multiple instance learning methods
- Achieves state-of-the-art performance on pathology tasks while requiring less specialized training
- Creates more transferable representations applicable across multiple diagnostic applications
This advancement matters for medical imaging by enabling more efficient and accurate pathology analysis while reducing the need for task-specific annotations—potentially accelerating diagnostic workflows in clinical settings.