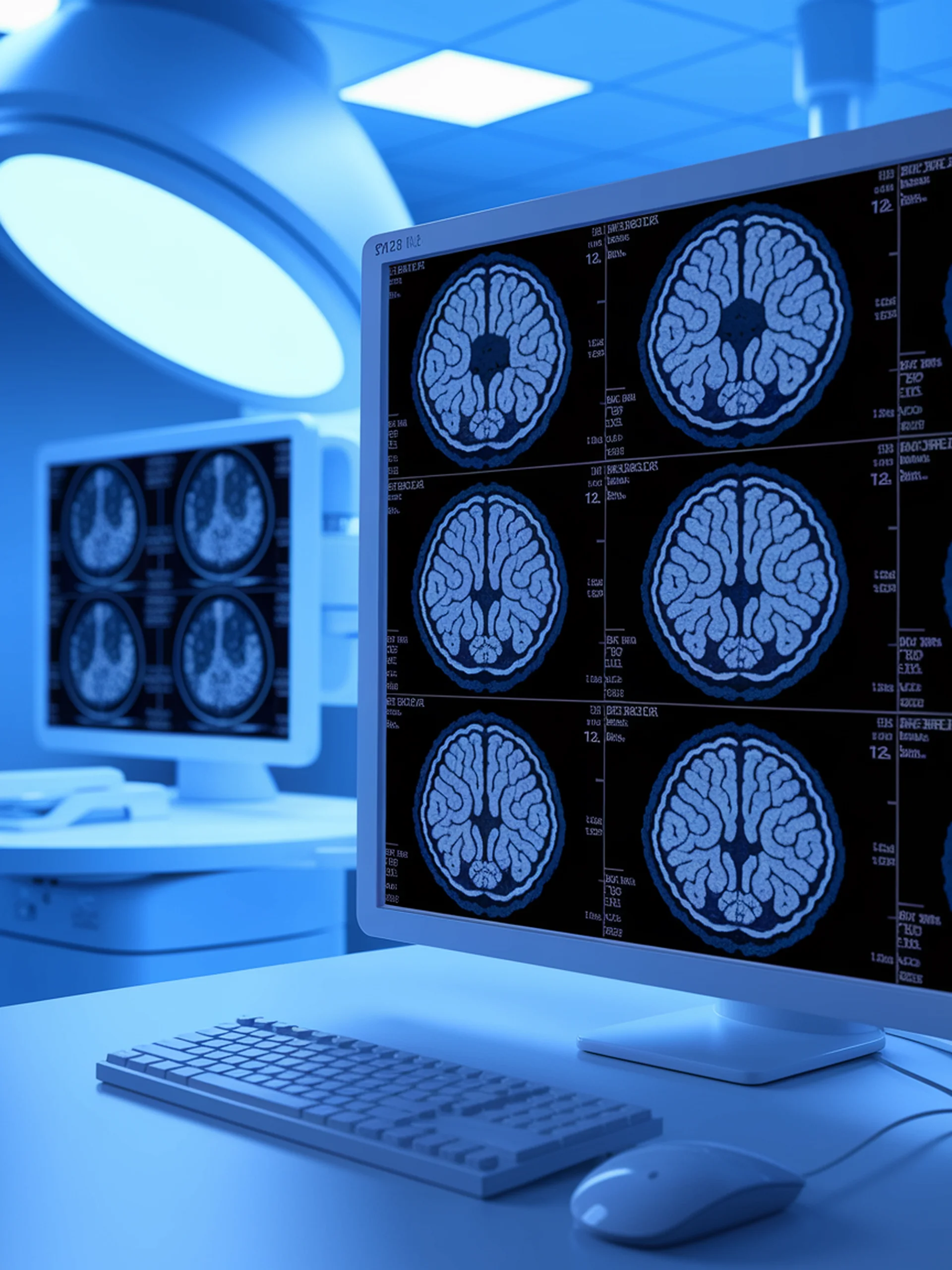
AXUNet: Advancing Brain Tumor Detection
Combining CNN and Self-Attention for Enhanced Medical Imaging
This research introduces a novel deep learning architecture that significantly improves brain tumor segmentation accuracy by combining Xception CNN with self-attention mechanisms.
Key Innovations:
- Integration of dot-product self-attention modules with Xception backbone for superior feature extraction
- Utilizes multiple MRI sequences (T1CE, T2, FLAIR) for comprehensive tumor analysis
- Trained on the BraTS 2021 dataset, incorporating diverse tumor presentations
- Architecture specifically optimized for medical imaging challenges
Clinical Impact: Accurate tumor segmentation directly impacts treatment planning and patient outcomes by enabling precise tumor boundary identification, potentially reducing surgical complications and improving targeted therapies.