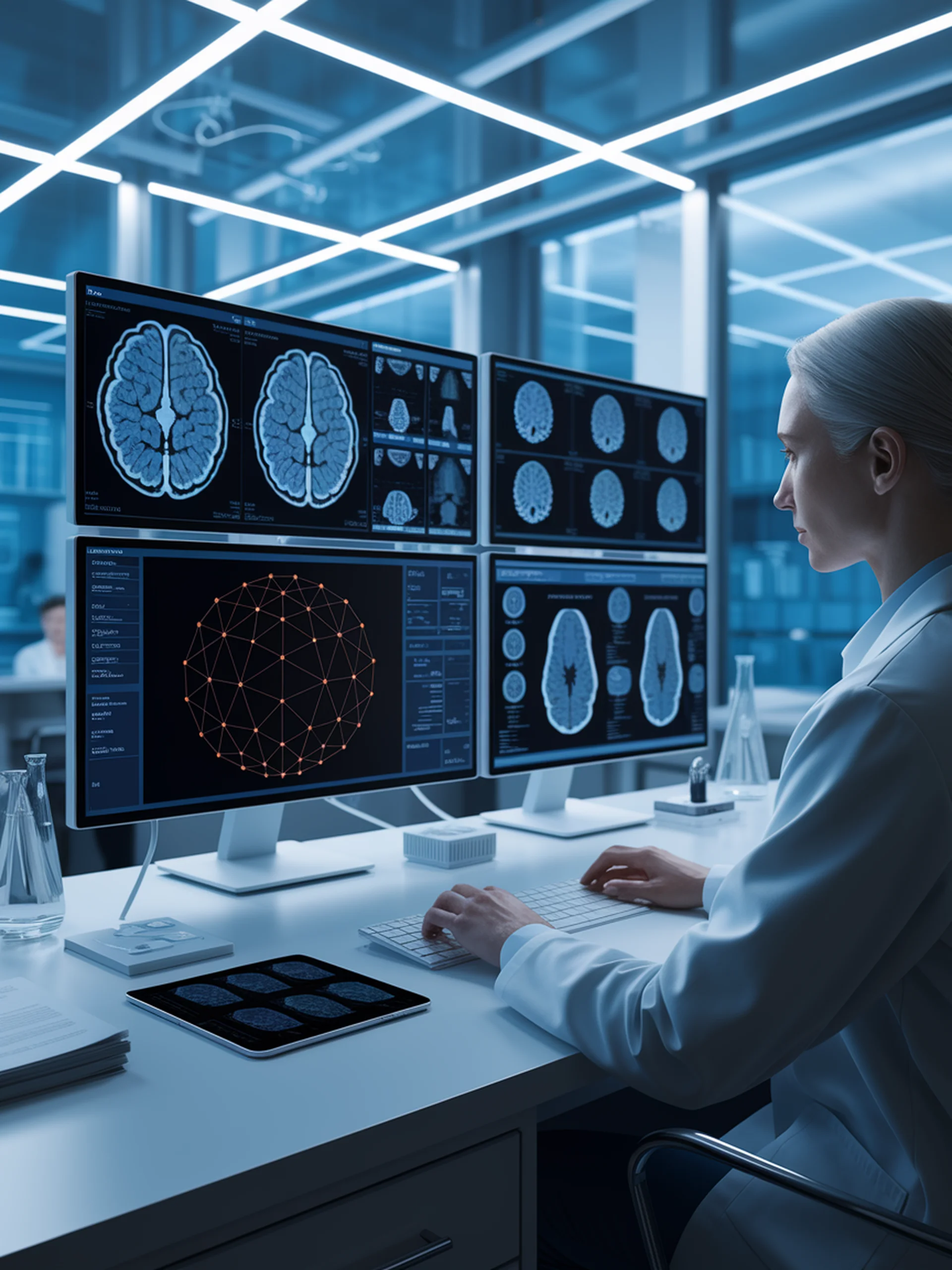
Improving Medical AI Consistency
How LLMs Generate Better Medical Question-Answering Systems
This research introduces a Semantically Equivalent Question Augmentation (SEQA) framework that enhances medical visual question-answering systems by addressing linguistic variability problems.
- Uses LLMs to generate diverse but semantically equivalent question phrasings
- Creates consistent responses across different ways of asking the same question
- Improves performance across multiple medical image datasets (SLAKE, VQA-RAD, PathVQA)
- Bridges critical semantic gaps in medical AI interpretation
This innovation matters because consistent, reliable medical AI is essential for clinical applications where answer reliability directly impacts patient care and decision support.
Bridging the Semantic Gaps: Improving Medical VQA Consistency with LLM-Augmented Question Sets