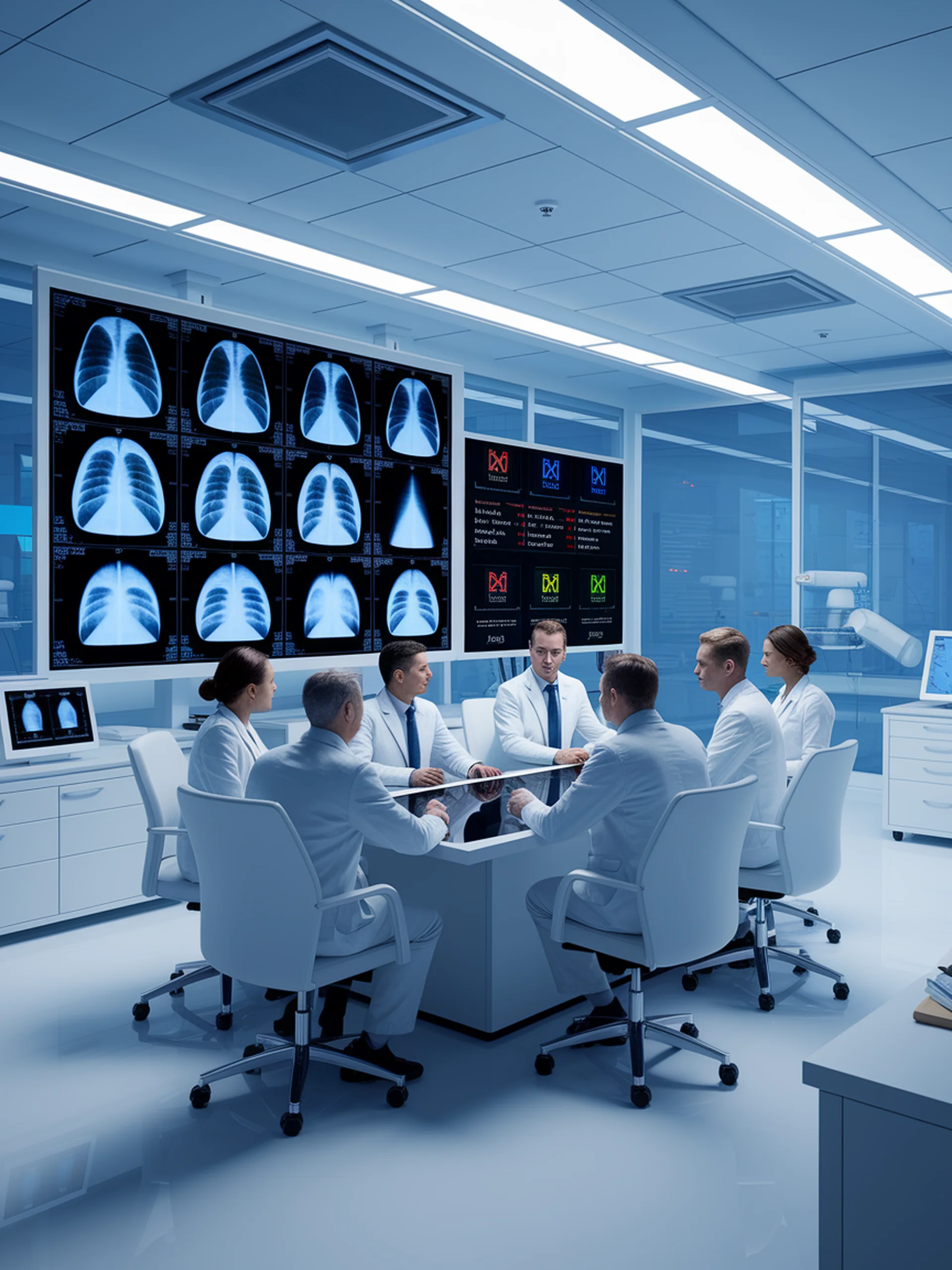
Reimagining Medical AI with Multi-annotation Data
Advancing multi-task capabilities in medical foundation models through data-centric innovation
This research presents a novel data-centric approach to medical AI by introducing an image-centric multi-annotation X-ray dataset (IMAX) that significantly improves multi-task learning capabilities.
- Creates a unified annotation framework that preserves image-task relationships for more effective multi-task learning
- Implements a novel progressive training strategy that balances single-task and multi-task learning objectives
- Demonstrates superior performance across multiple medical imaging tasks compared to conventional approaches
- Provides a scalable methodology for developing more capable medical foundation models
This innovation addresses a critical gap in medical AI by shifting focus from mere data scaling to intelligent data organization, enabling more accurate diagnosis and interpretation across multiple medical conditions simultaneously.