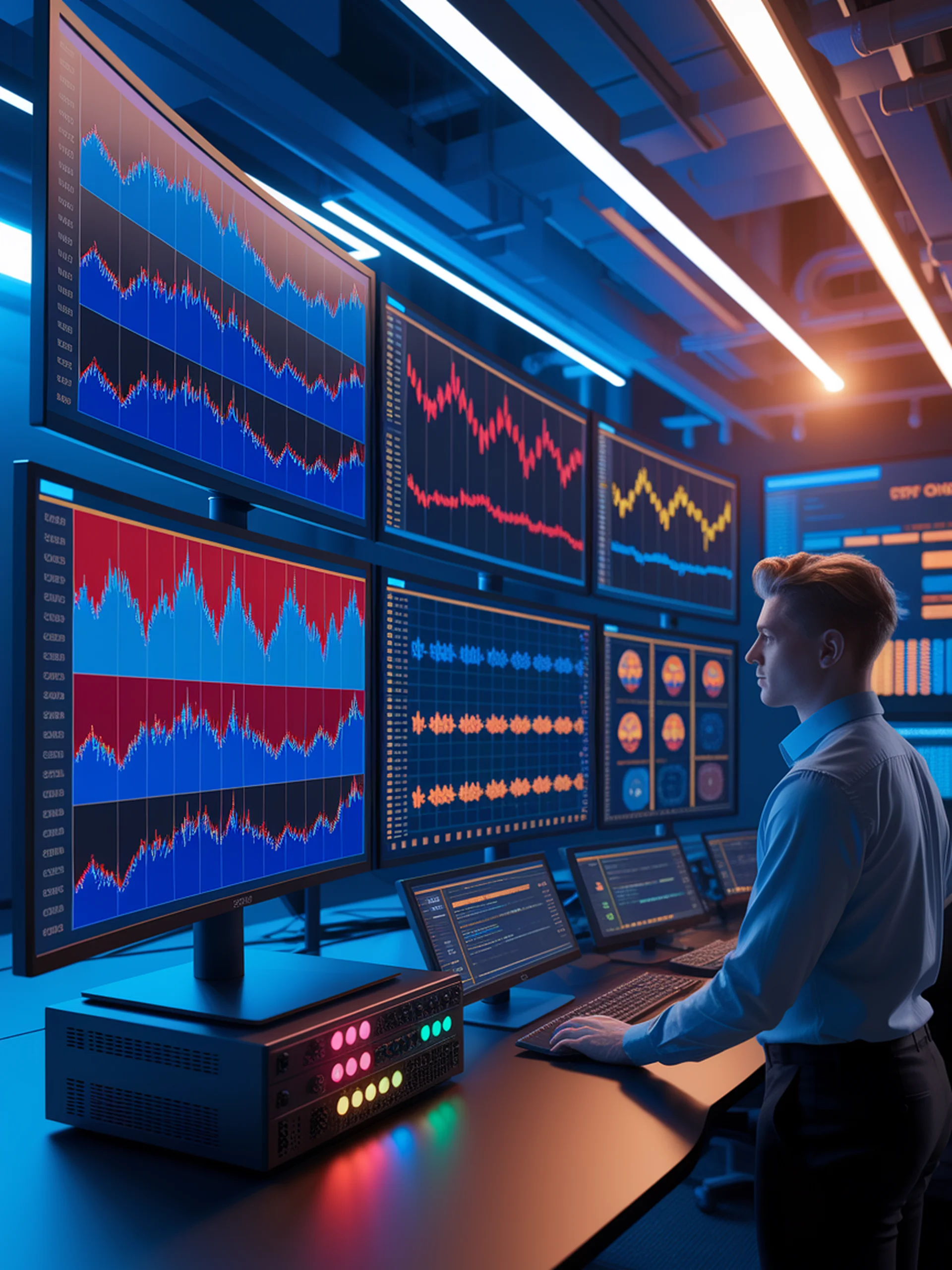
Visualizing Time Series Anomalies
Leveraging Multimodal LLMs for Enhanced Anomaly Detection
This research explores how multimodal large language models can detect anomalies in time series data through visual interpretation, similar to human analysis methods.
- Introduces a novel approach called VisualTimeAnoma that combines visual pattern recognition with language understanding
- Demonstrates how vision-language models can be applied beyond traditional text analysis to time-series data
- Shows potential for improved anomaly detection compared to conventional methods
- Offers new tools for security monitoring systems to identify unusual patterns
For security professionals, this research provides innovative techniques to enhance threat detection systems by leveraging AI's visual pattern recognition capabilities alongside textual analysis, potentially identifying security anomalies that traditional systems might miss.