Anomaly Detection with LLMs
Research on using LLMs for zero-shot or few-shot detection of anomalies, outliers, and unusual patterns across various domains
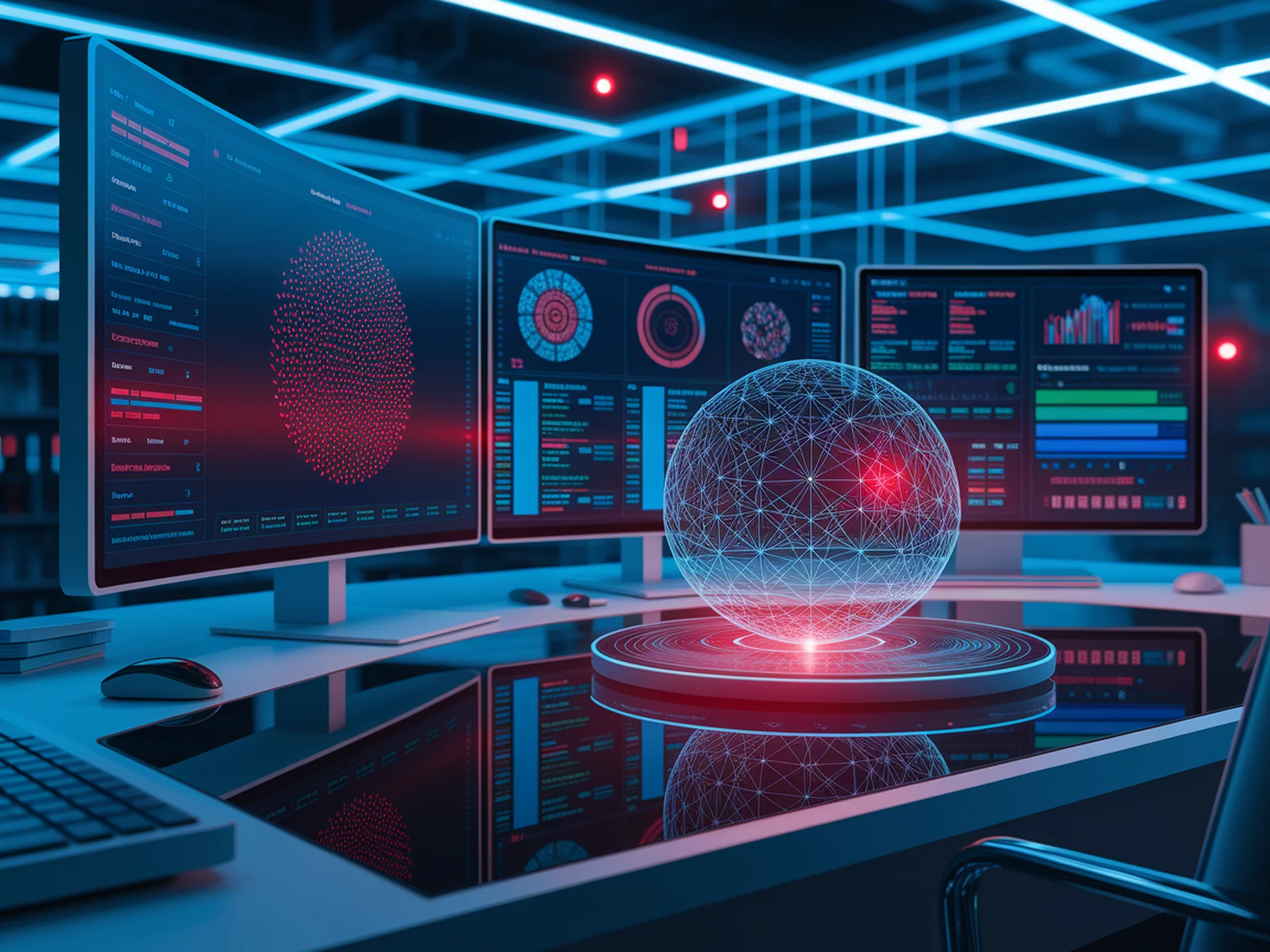
Anomaly Detection with LLMs
Research on Large Language Models in Anomaly Detection with LLMs
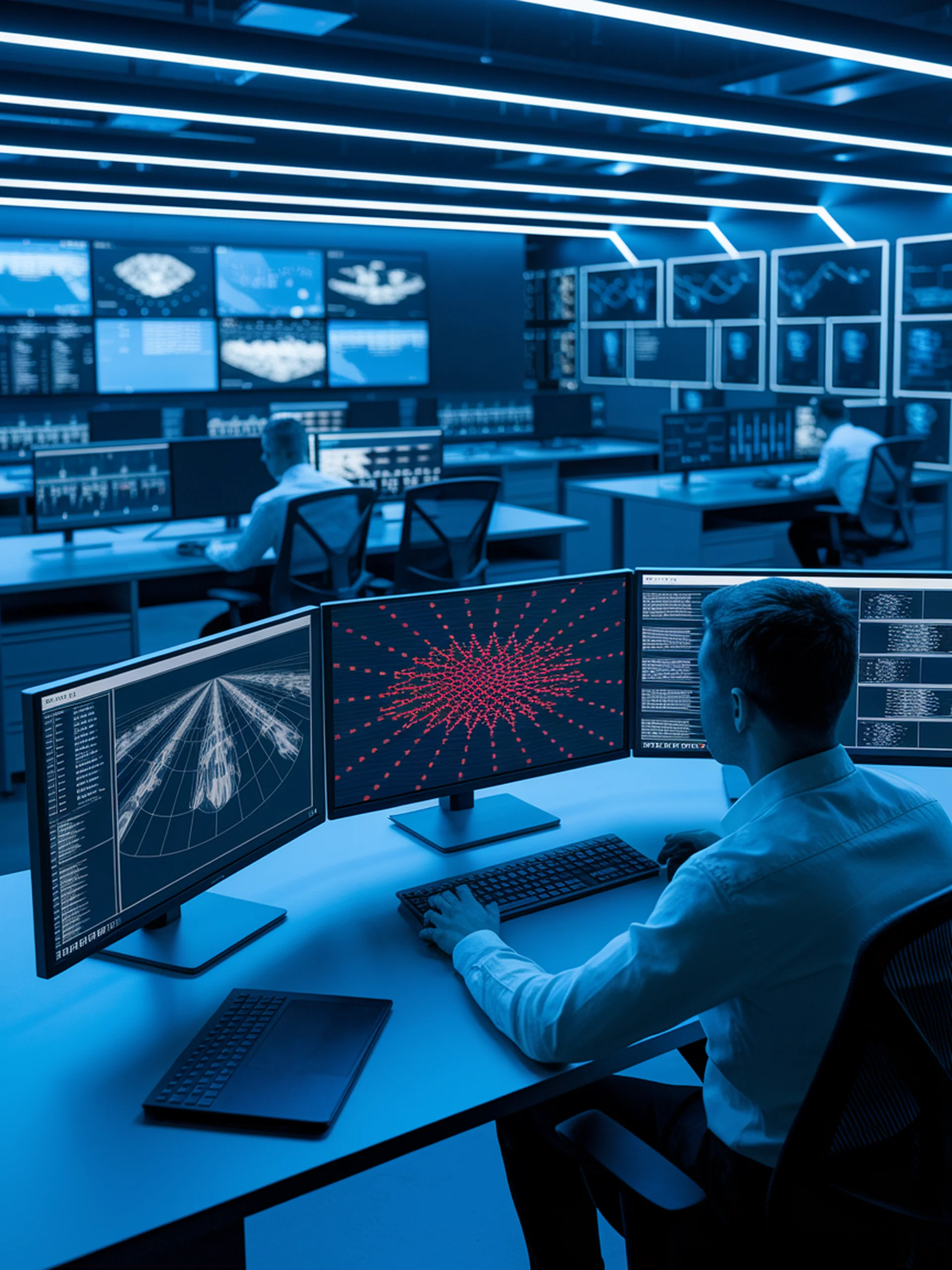
Zero-Shot Anomaly Detection with MLLMs
Detecting anomalies without prior training data
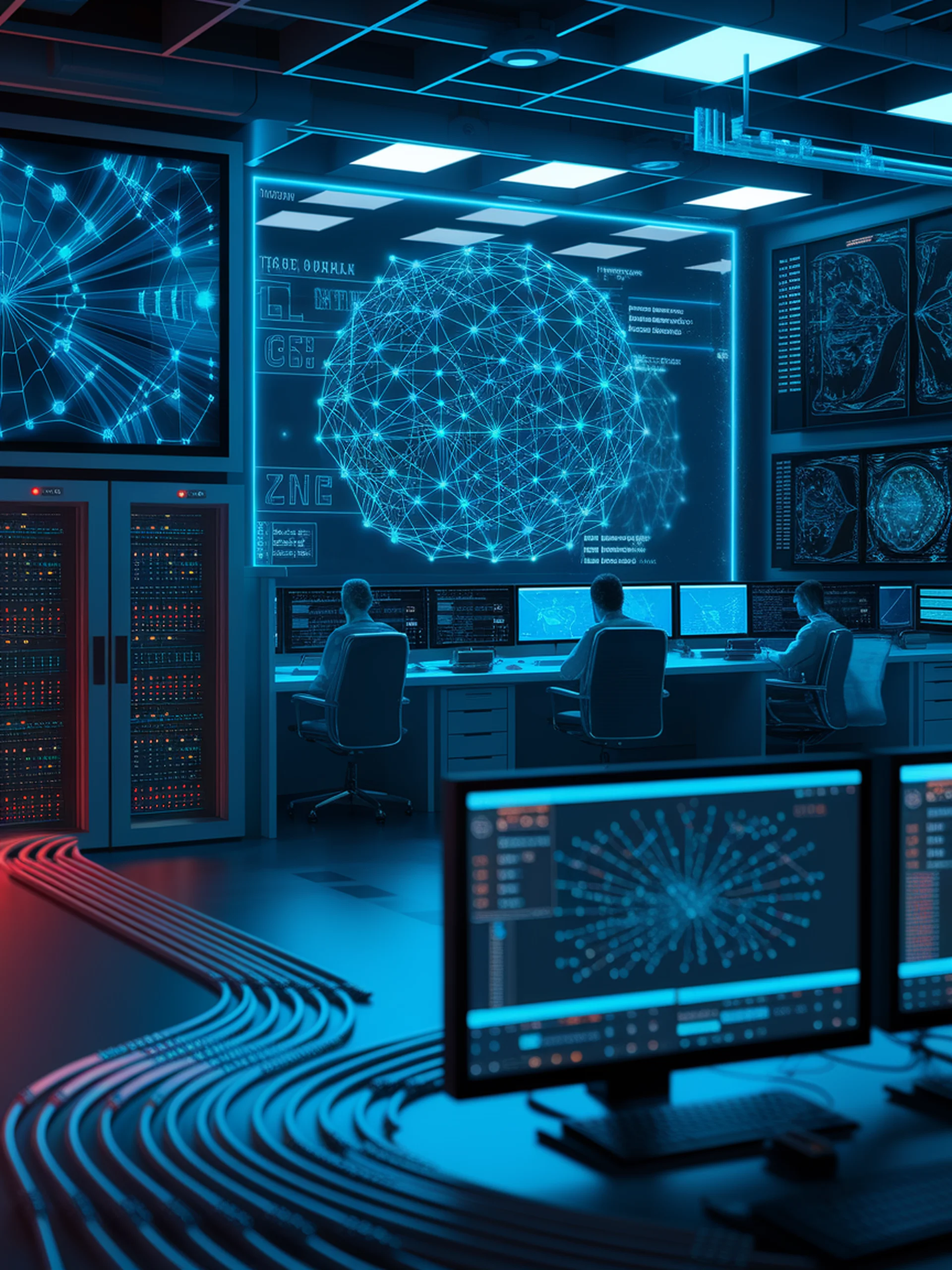
Detecting Advanced Cyber Threats with LLMs
Using AI language models to identify stealthy persistent threats
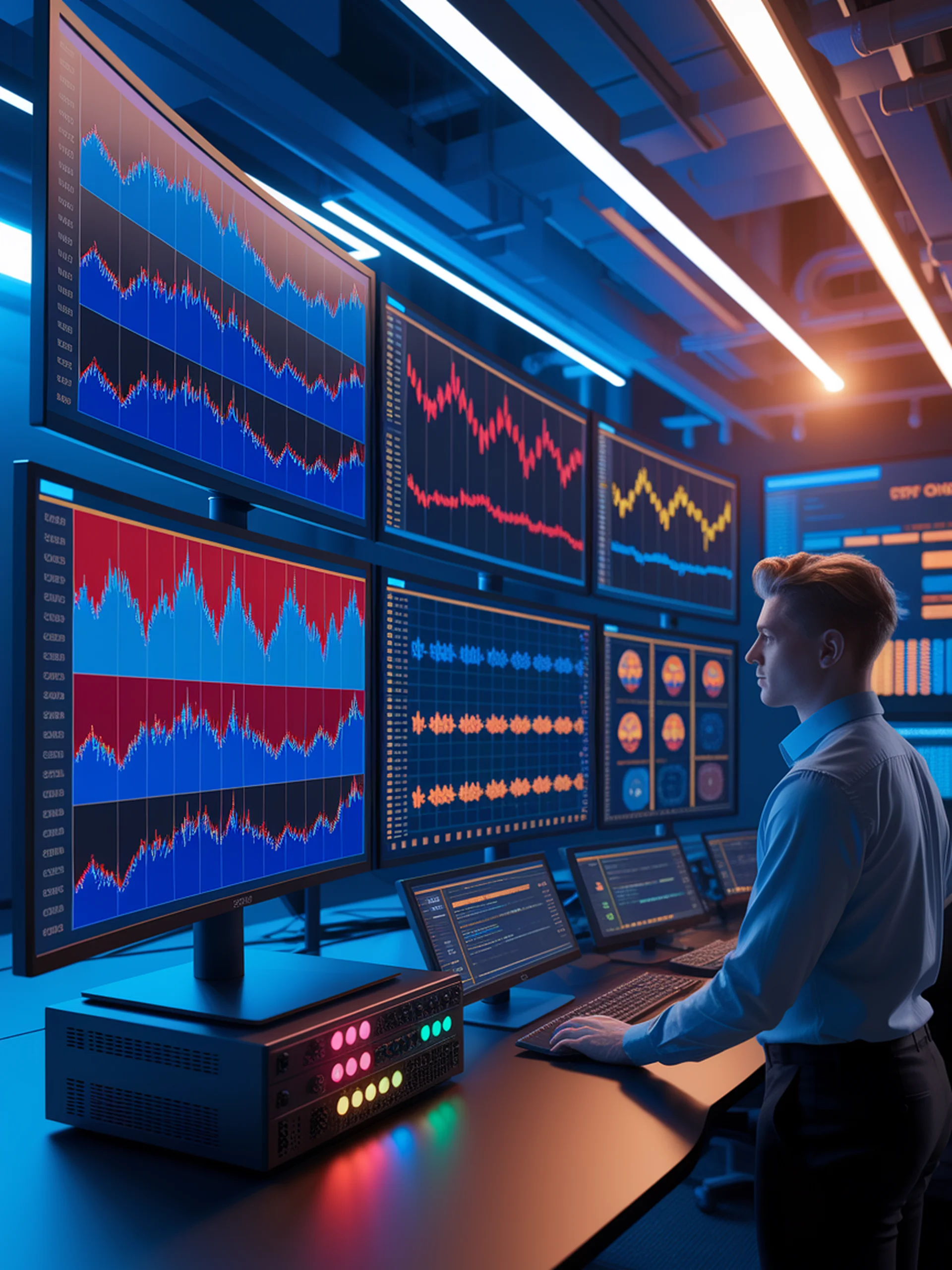
Visualizing Time Series Anomalies
Leveraging Multimodal LLMs for Enhanced Anomaly Detection
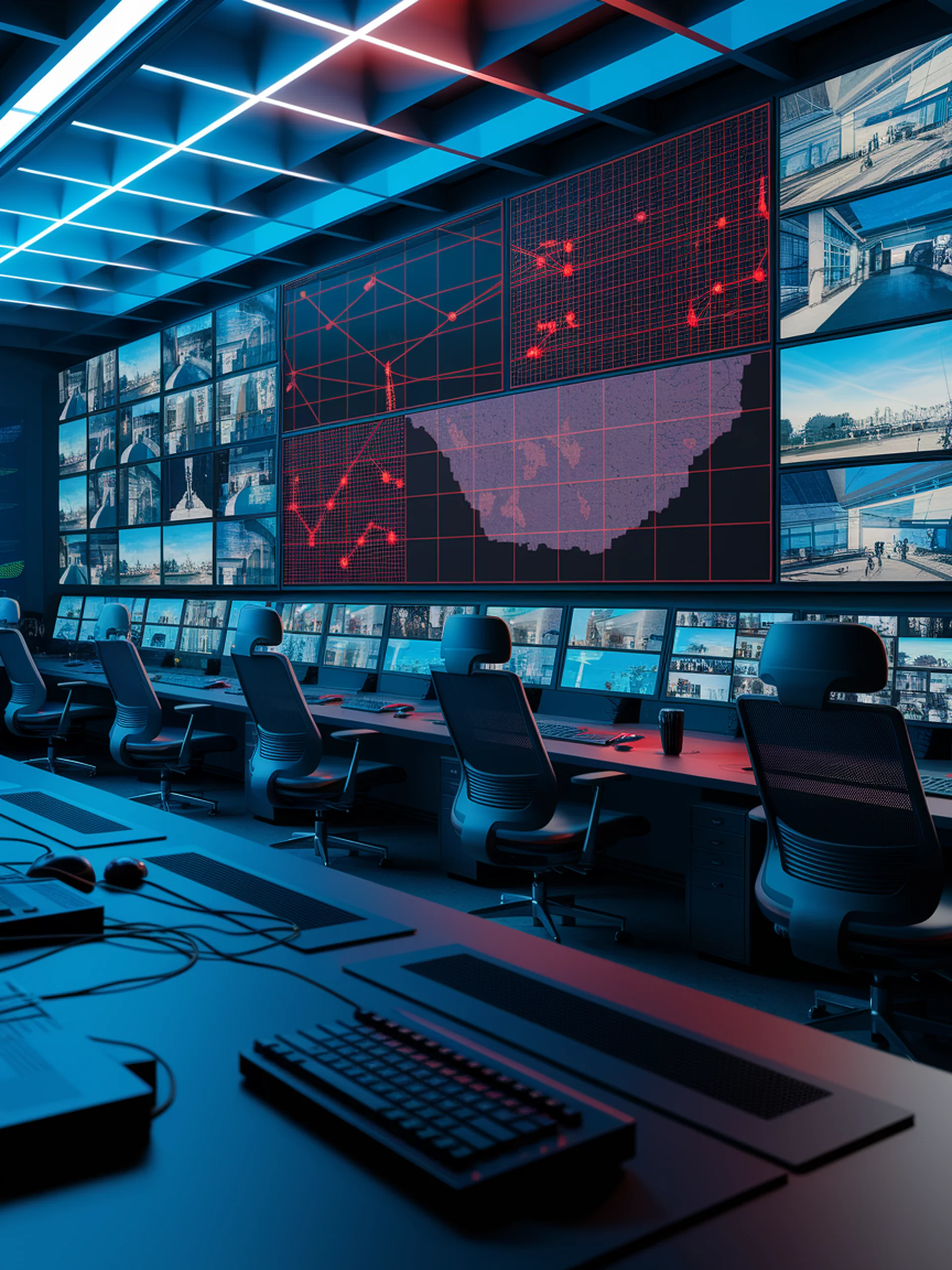
Sherlock: AI-Powered Video Anomaly Intelligence
Advanced LLM system for detecting and localizing abnormal events across multiple scenes
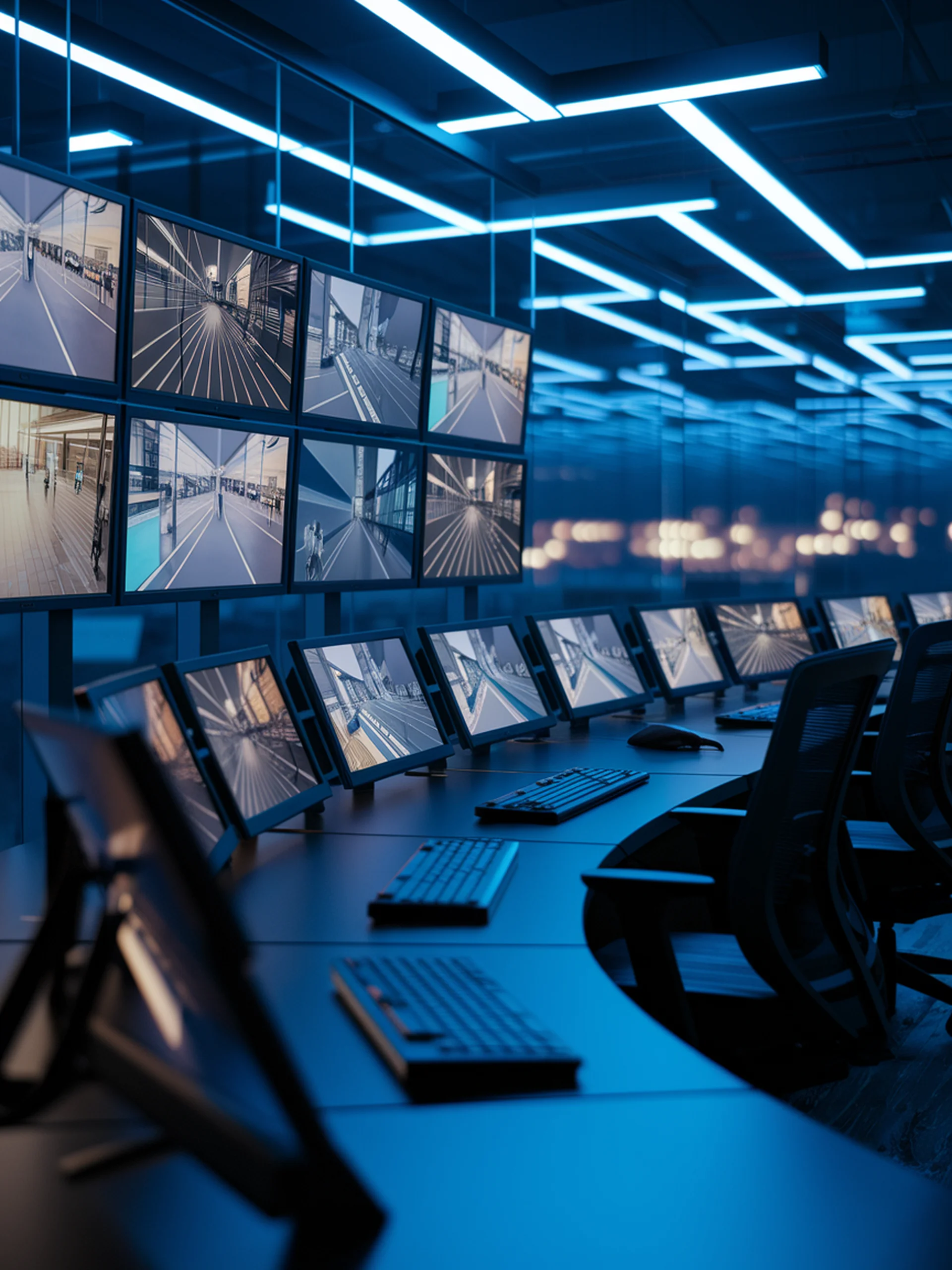
Zero-Shot Video Anomaly Detection
Customizable surveillance without retraining or specialized expertise
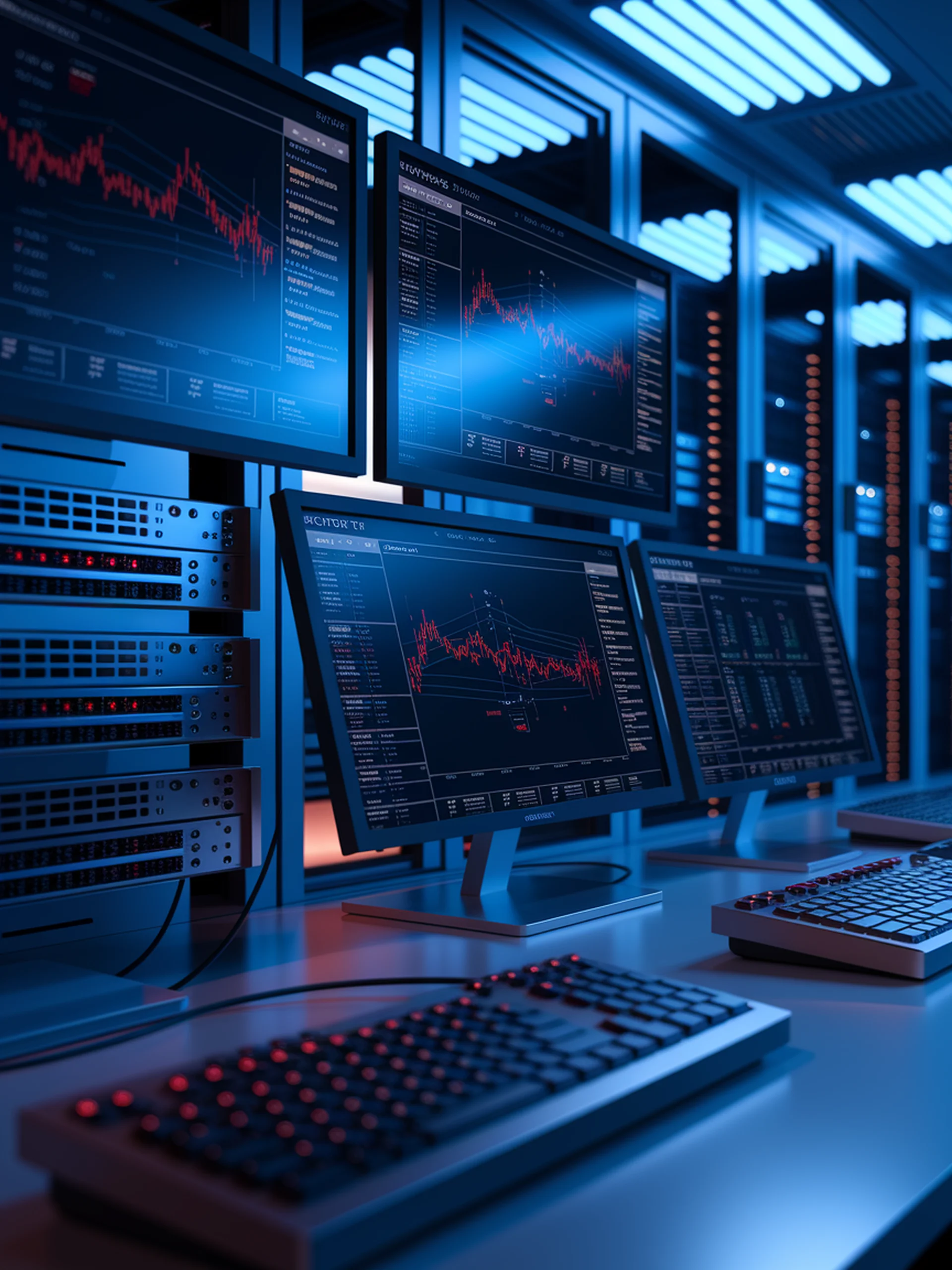
Efficient Log Anomaly Detection with LLMs
Using Parameter-Efficient Fine-Tuning for cost-effective security monitoring
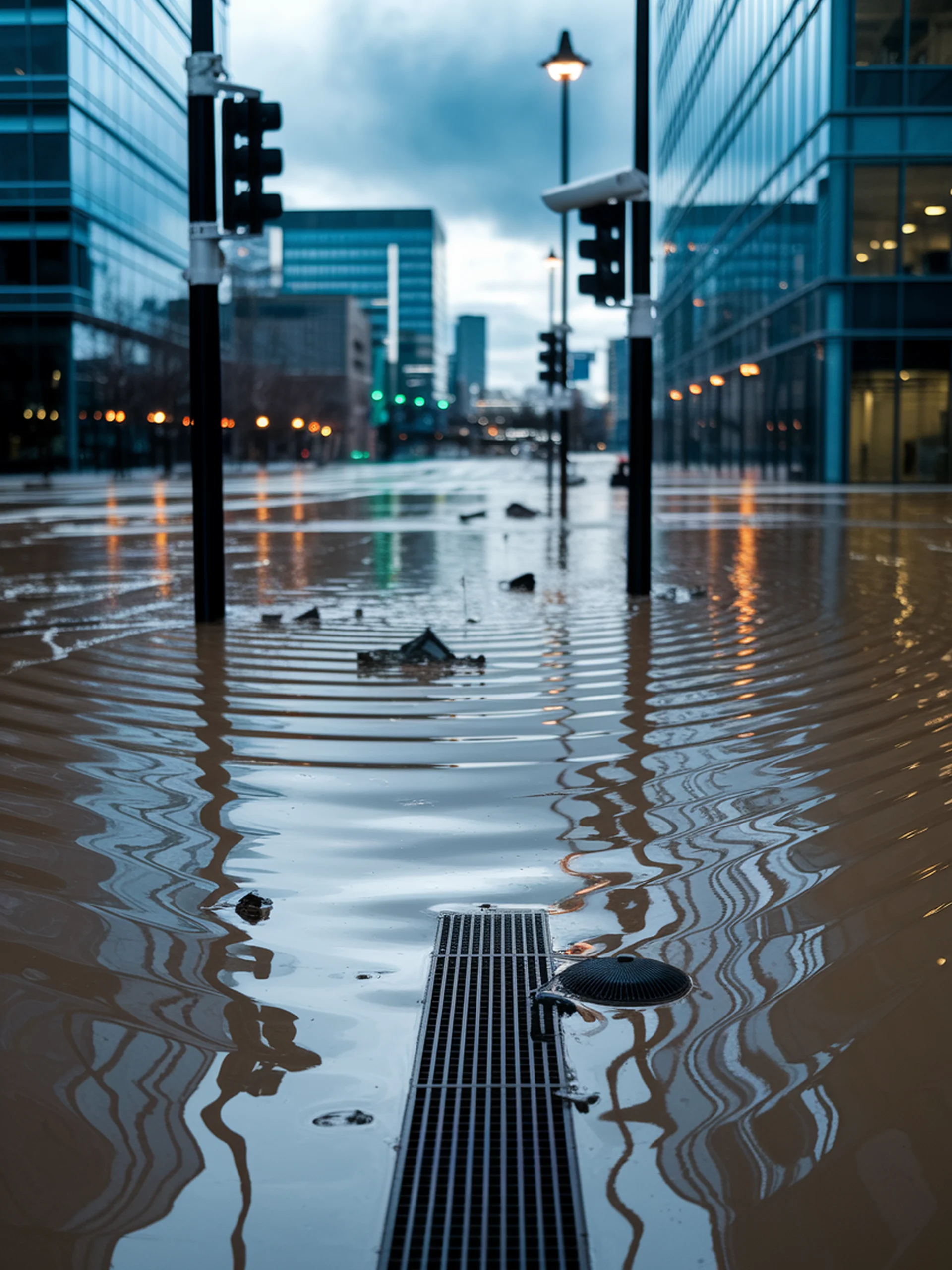
Smart Flood Detection Without Labels
Using Zero-Shot Learning & Bayesian Modeling for Urban Flooding
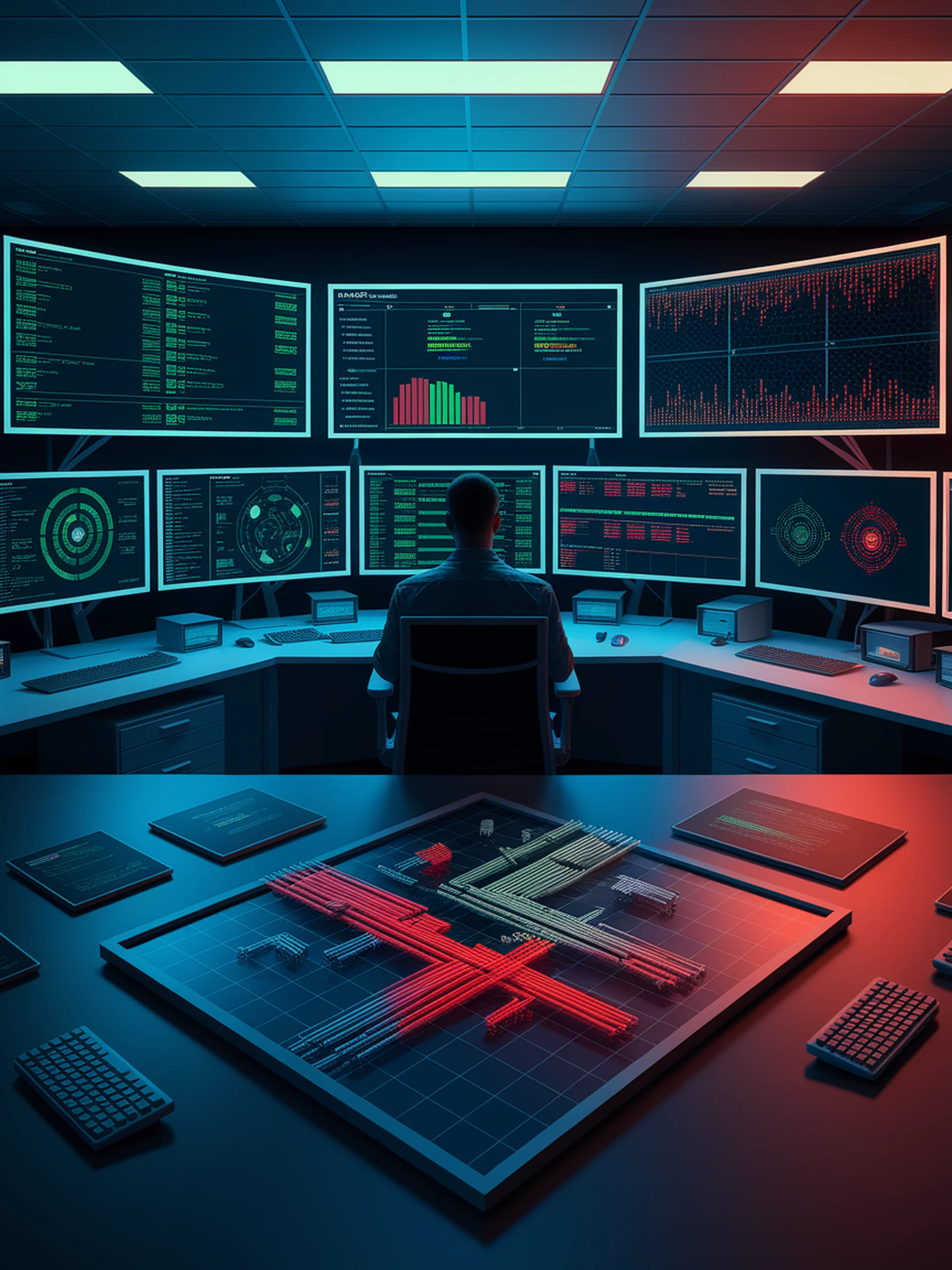
Smarter Log Anomaly Detection with LLMs
Leveraging LLaMA2 to enhance cybersecurity through intelligent log analysis
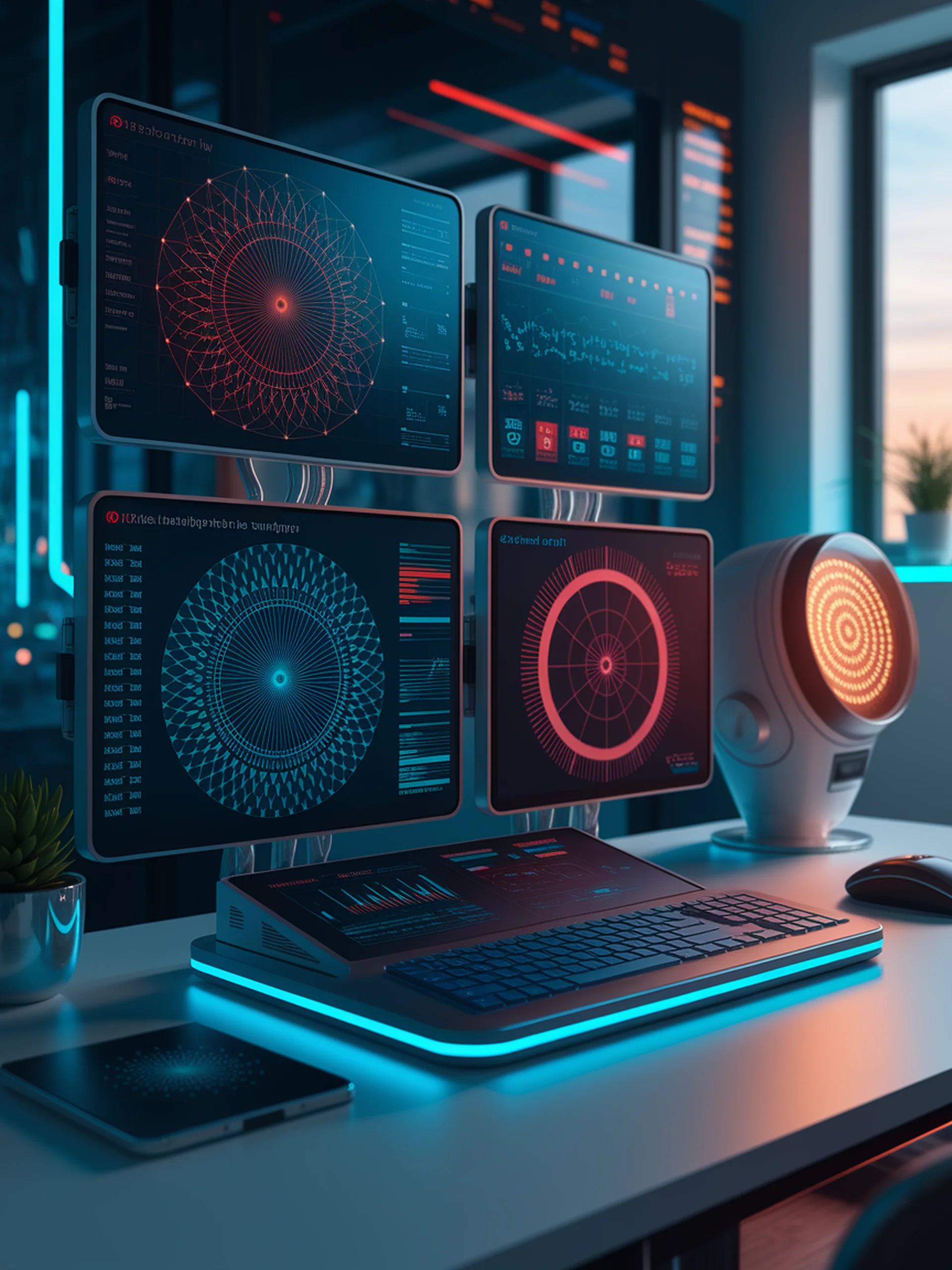
Improving AI Security Through Multi-Modal Detection
Enhancing out-of-distribution detection with cross-modal alignment
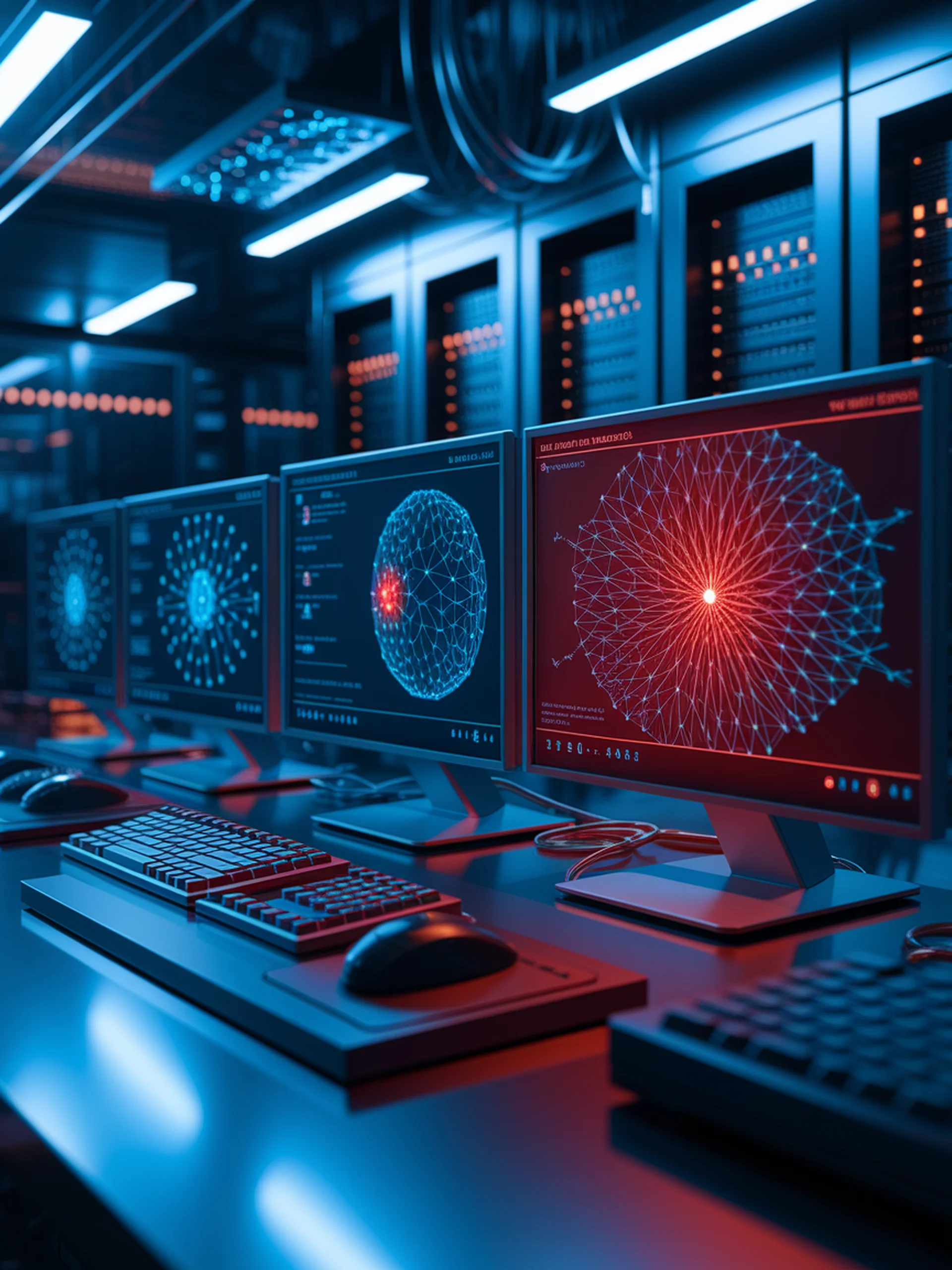
Detecting Ghost Behaviors in LLMs
A novel forensic approach for identifying abnormal LLM outputs
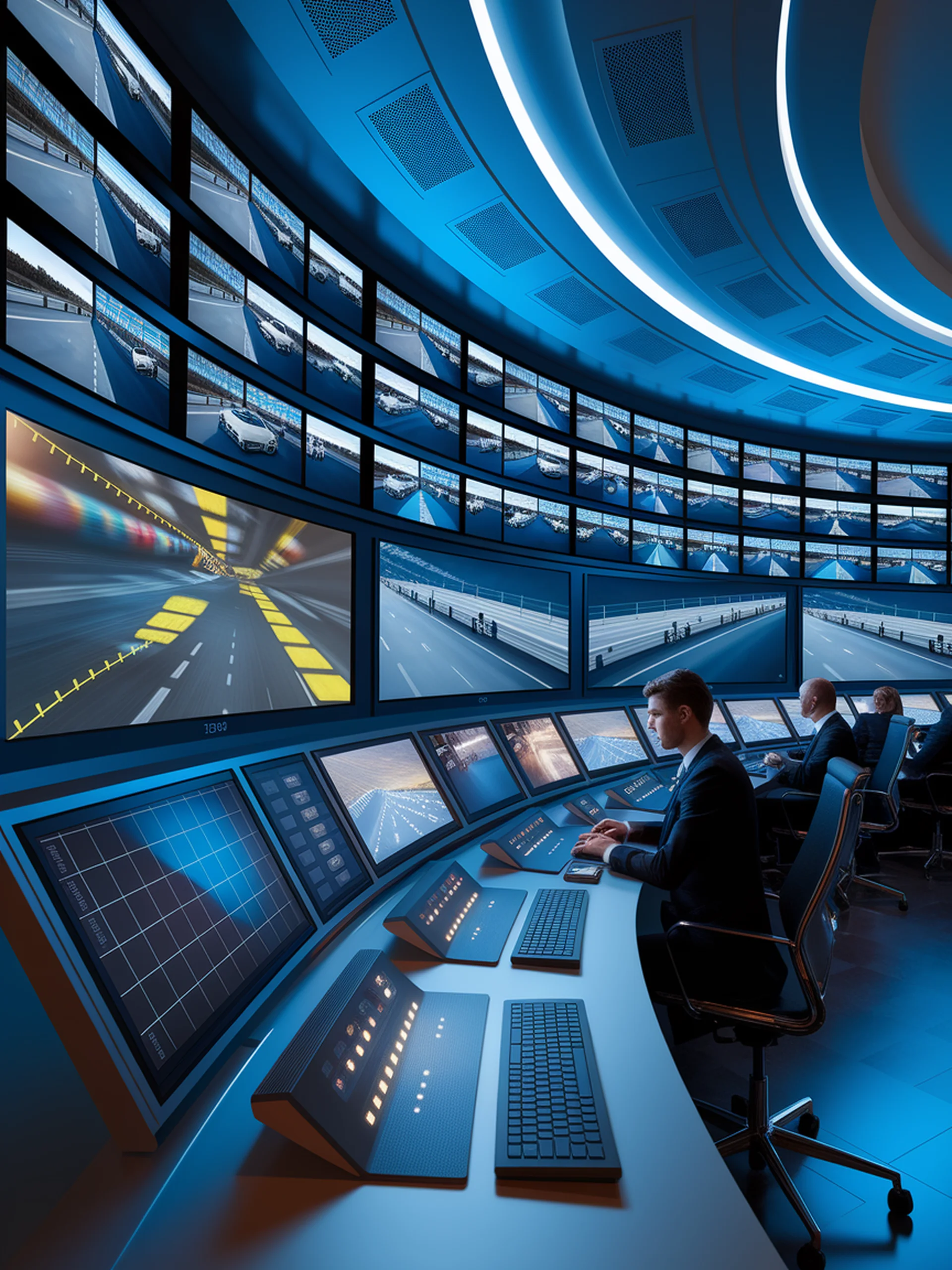
Precision Crash Detection for Traffic Safety
HybridMamba: Advanced temporal modeling for pinpointing crash moments
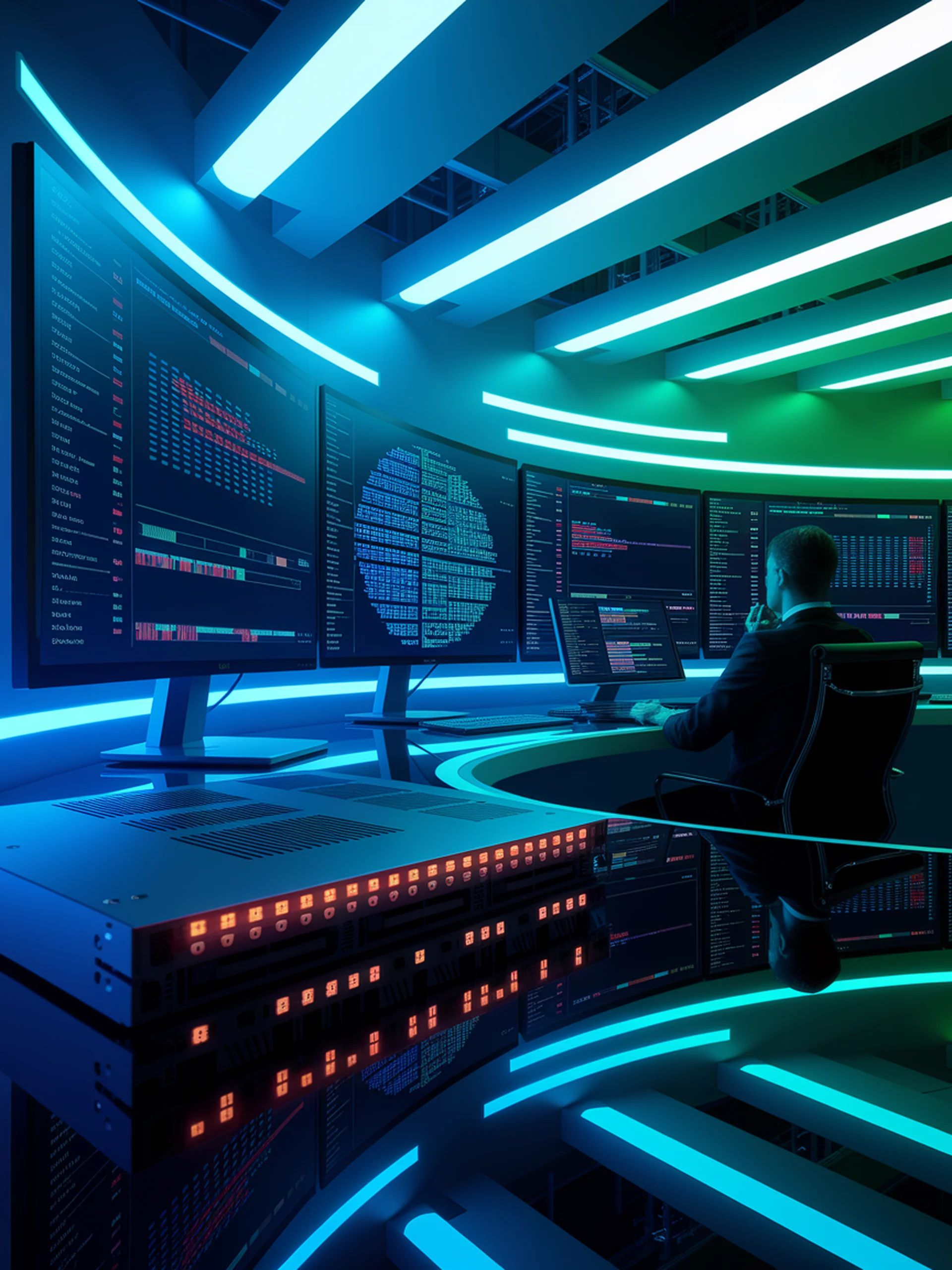
Revolutionizing Log Parsing with LLMs
Leveraging AI to transform unstructured logs into actionable security insights
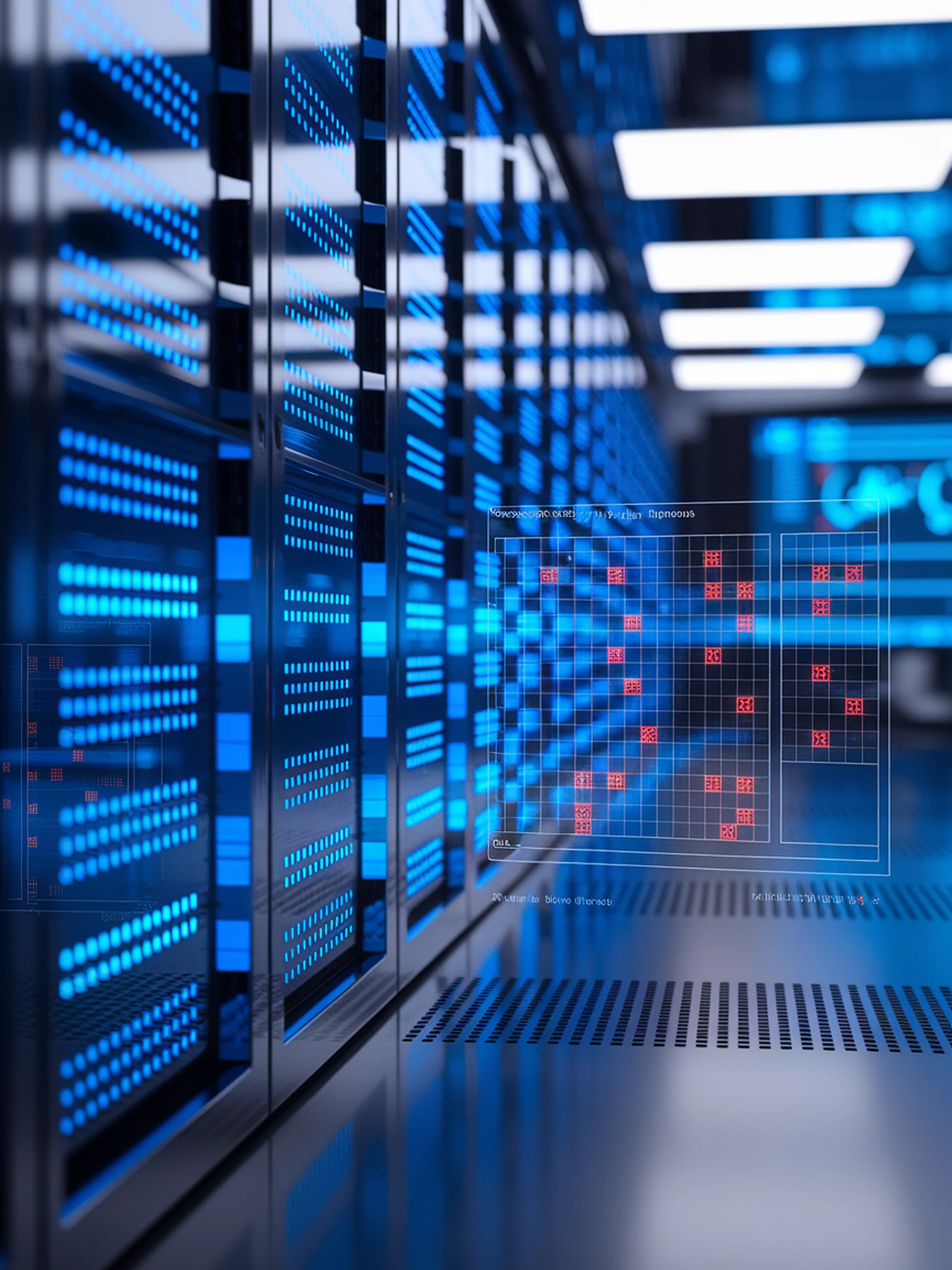
Zero-Shot Error Detection for Data Integrity
Harnessing LLMs to Detect Errors in Tabular Data Without Training
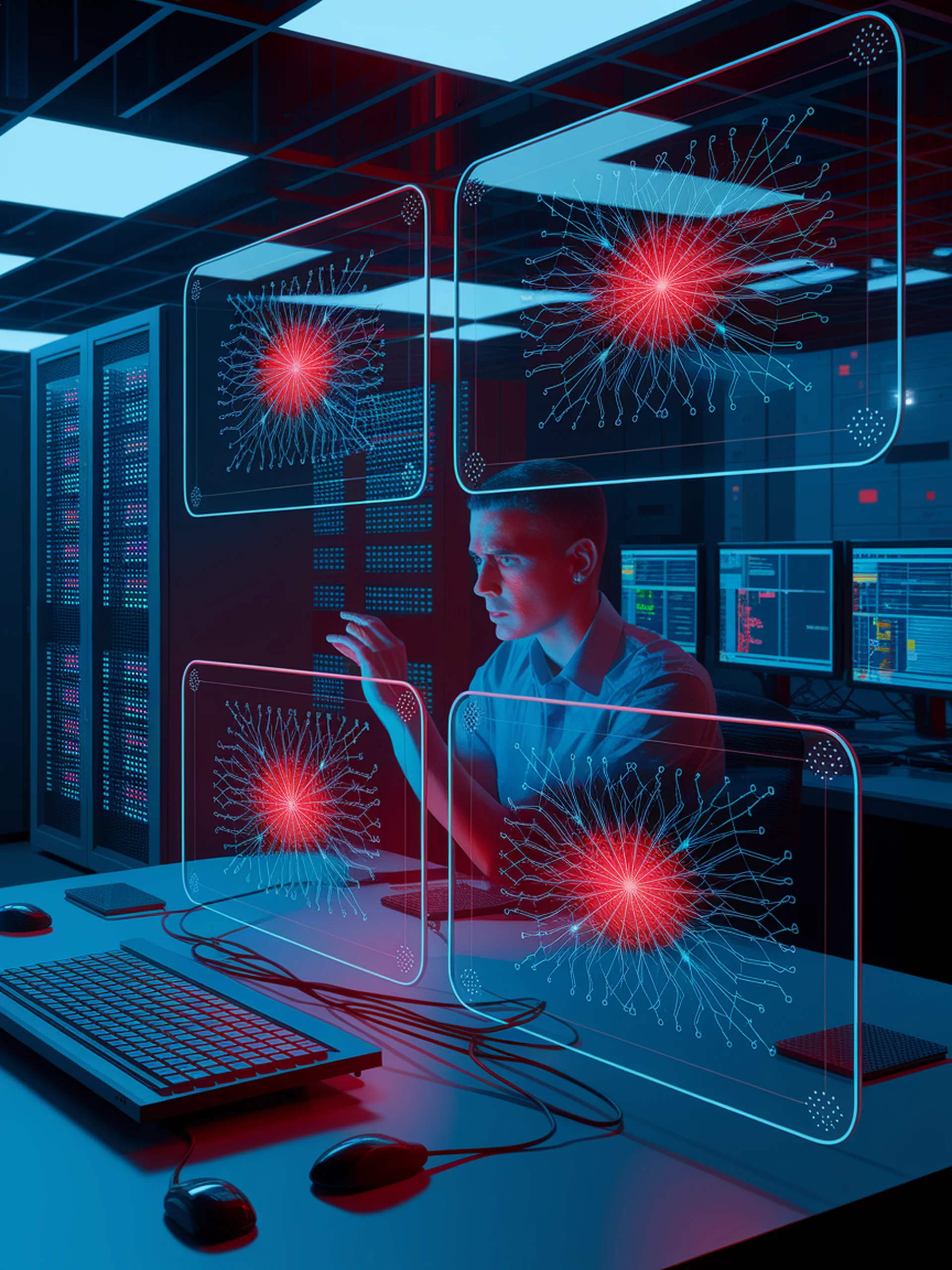
Detecting LLM 'Quirks' That Supervision Misses
Using model internals to identify unexpected anomalies in AI systems
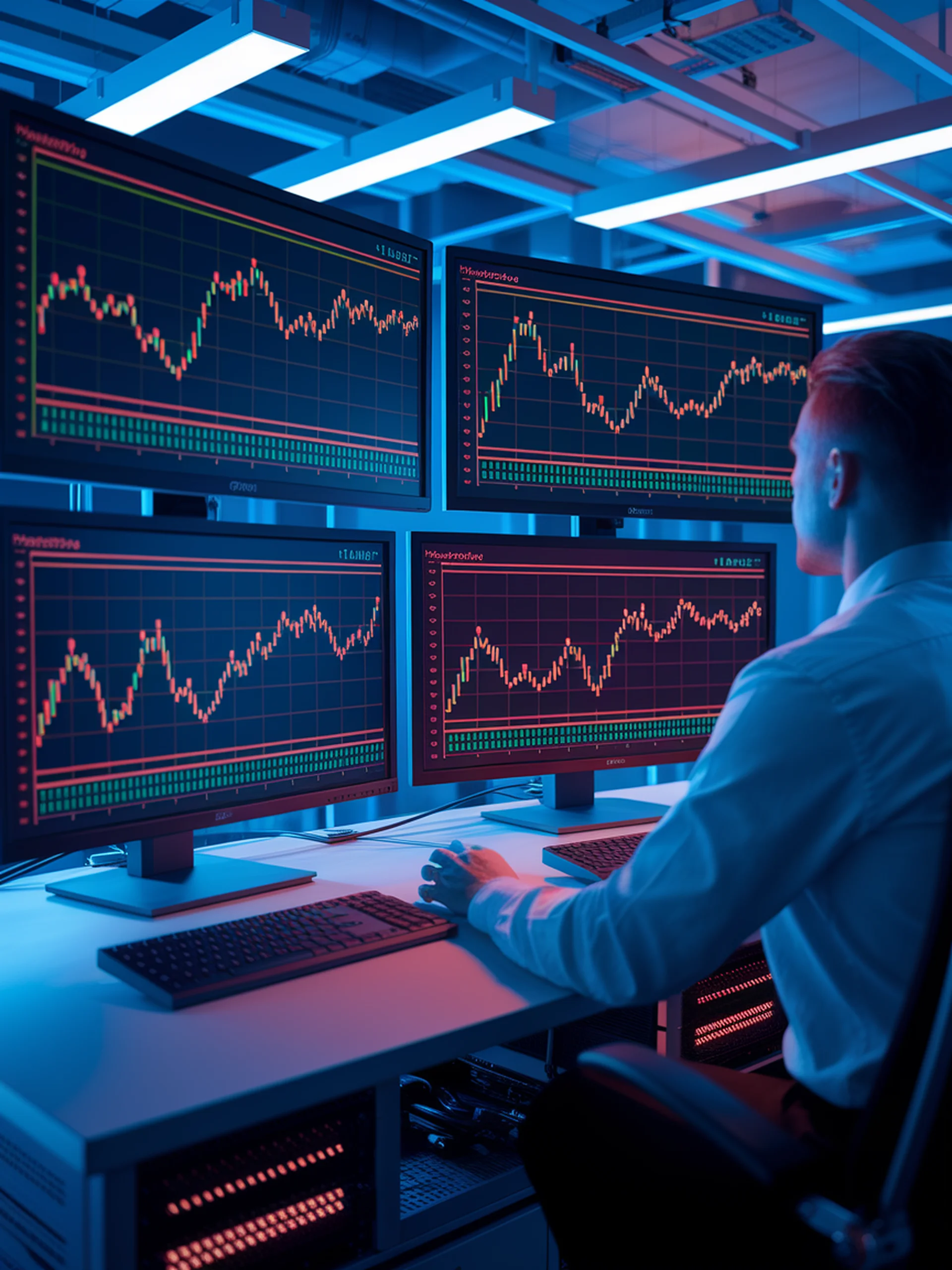
Smarter Anomaly Detection with MADLLM
Leveraging Language Models for Multivariate Time Series Analysis
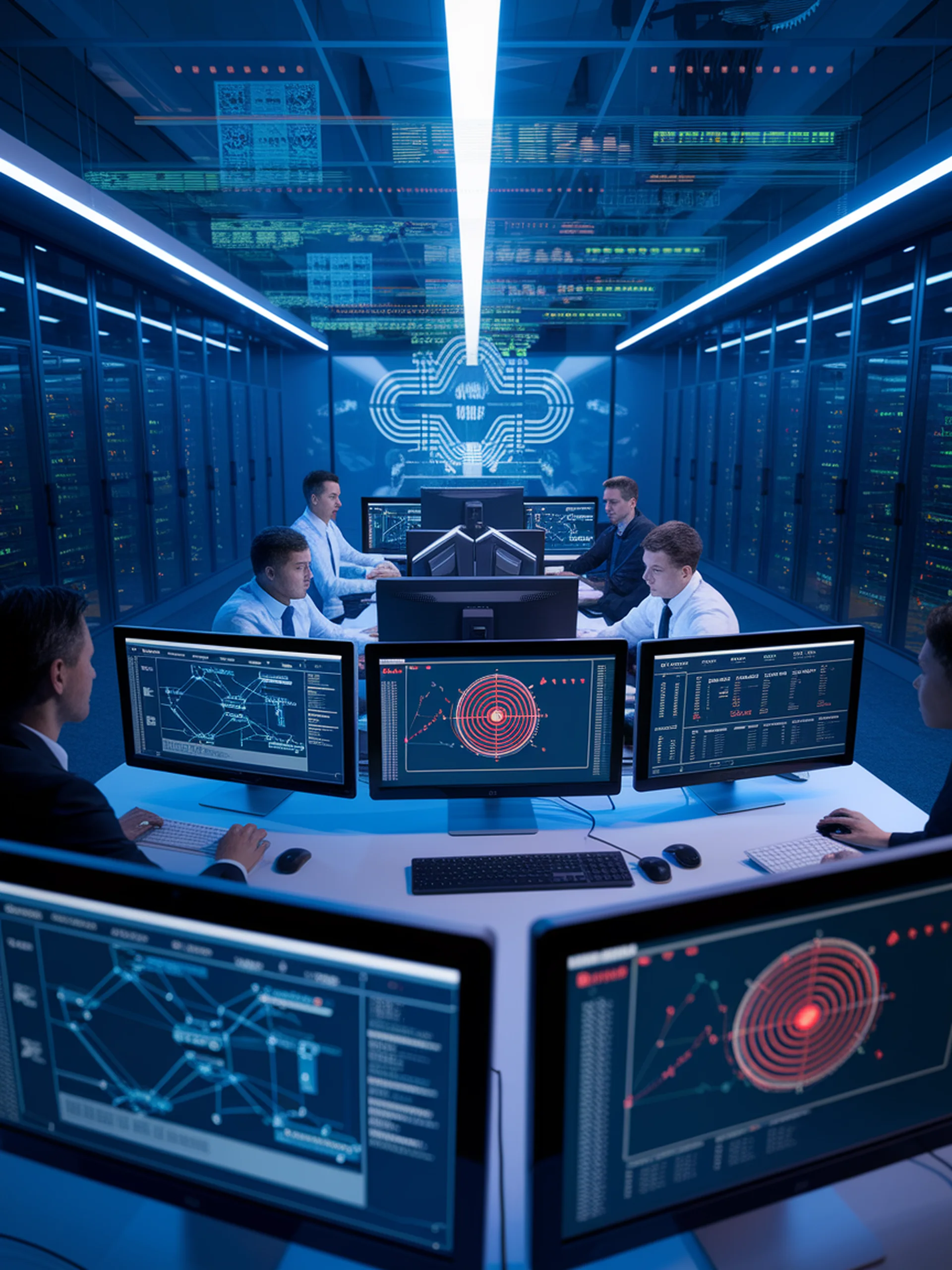
Automating Root Cause Analysis with AI
Using LLMs to speed up incident resolution in cloud systems
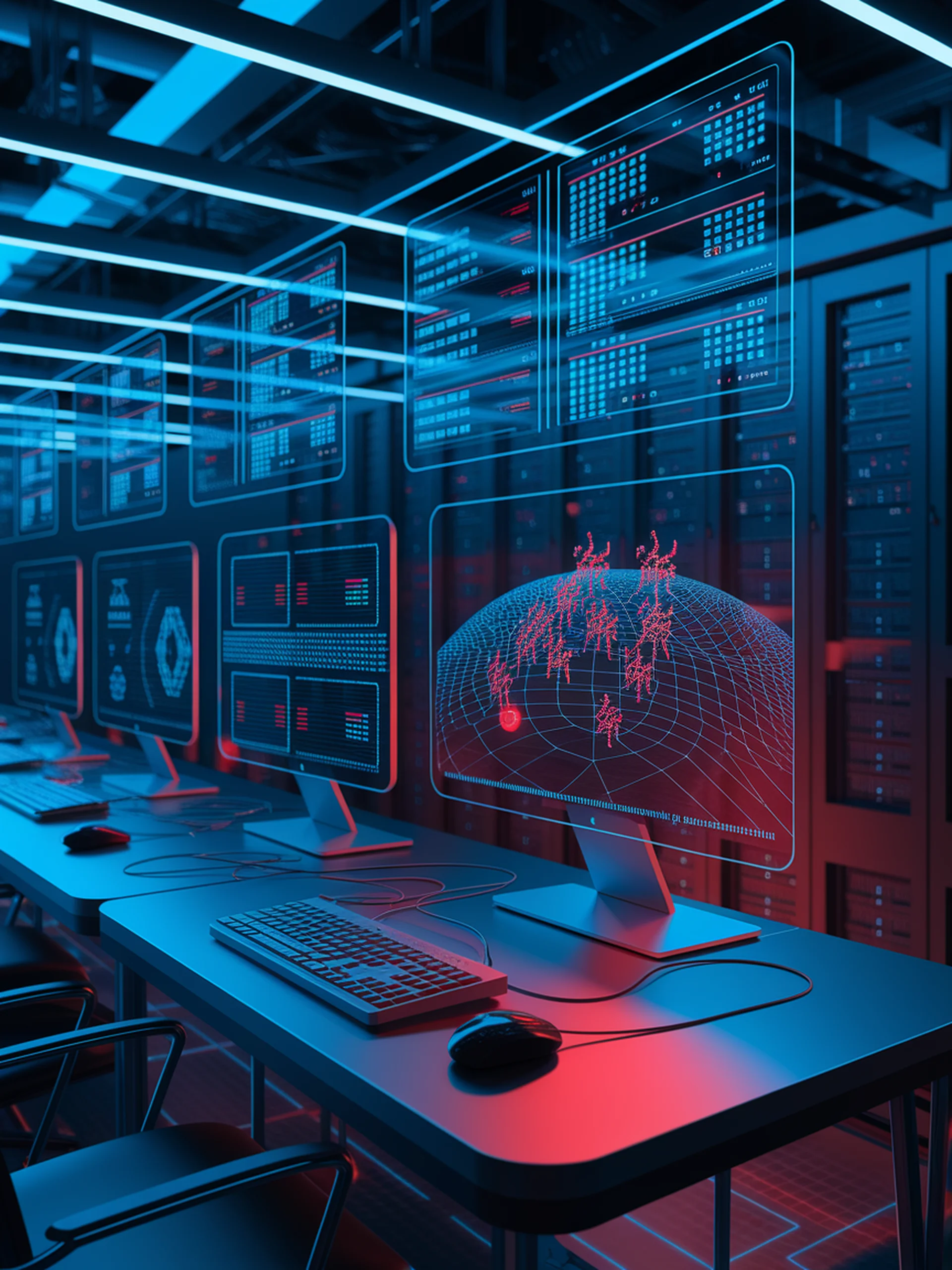
AnomalyGen: Revolutionizing Log-Based Security
Using LLMs to Generate Realistic Anomaly Data for Better Detection
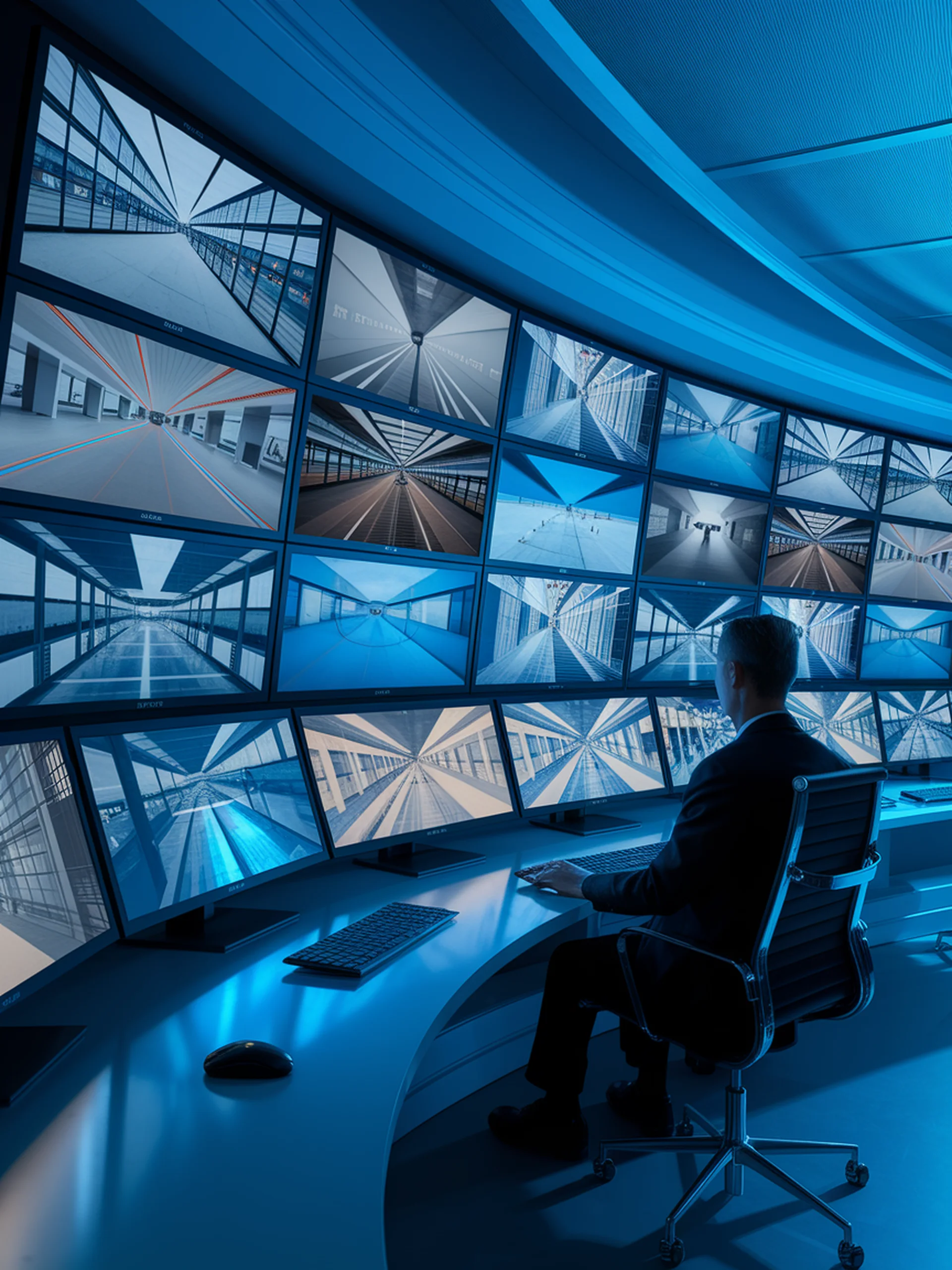