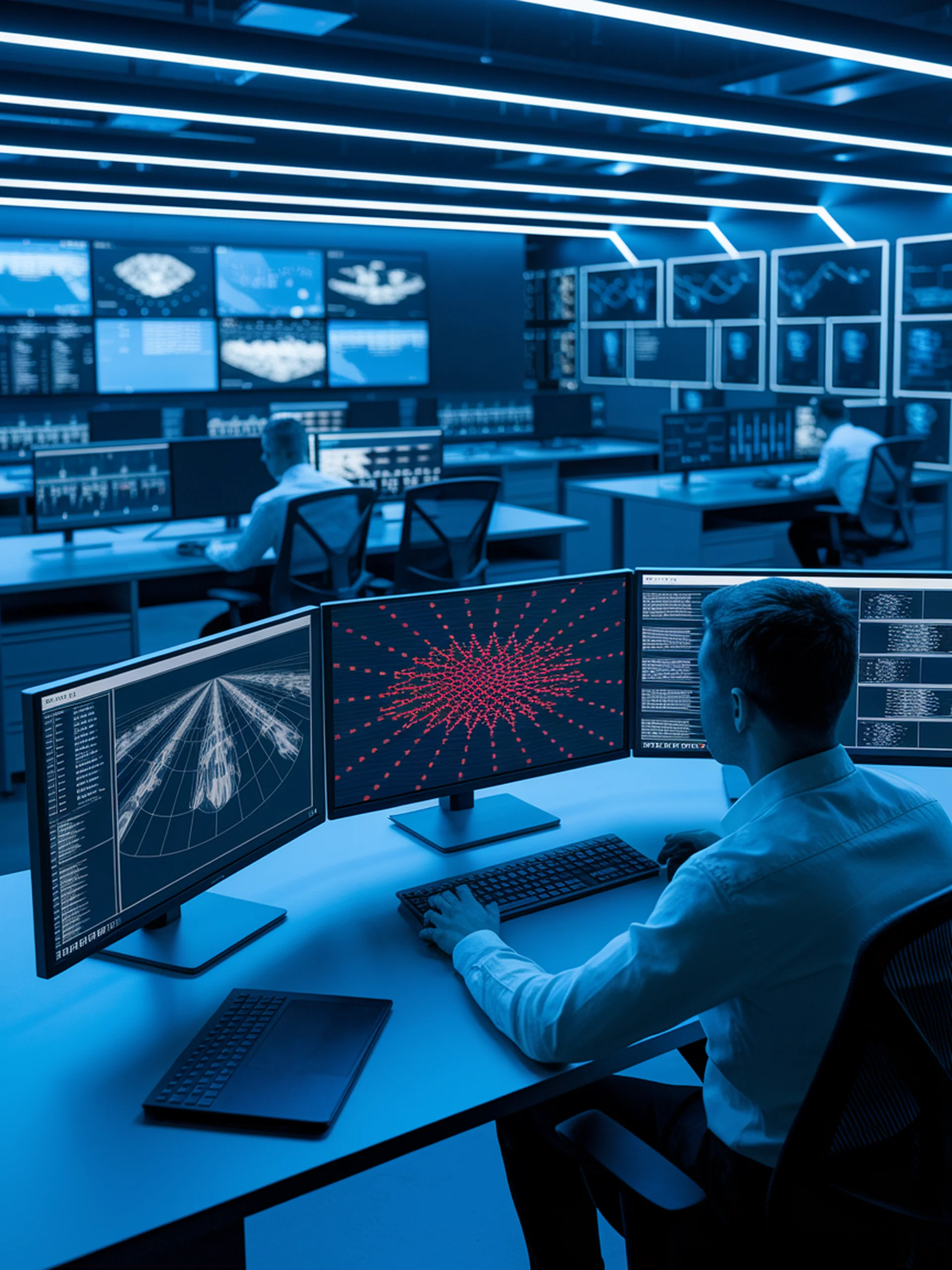
Zero-Shot Anomaly Detection with MLLMs
Detecting anomalies without prior training data
This research explores how Multimodal Large Language Models can detect and reason about anomalies in images without requiring training on normal samples.
- Introduces a novel zero-shot approach that makes anomaly detection practical for data-restricted scenarios
- Demonstrates MLLMs' capability to identify visual abnormalities across various contexts including security applications
- Presents a new framework and benchmark for evaluating anomaly detection performance
- Shows promising results for security applications where detecting unusual patterns with minimal training is crucial
For security professionals, this research offers a path to implement anomaly detection systems in environments where collecting comprehensive training data is challenging or impossible.
Towards Zero-Shot Anomaly Detection and Reasoning with Multimodal Large Language Models