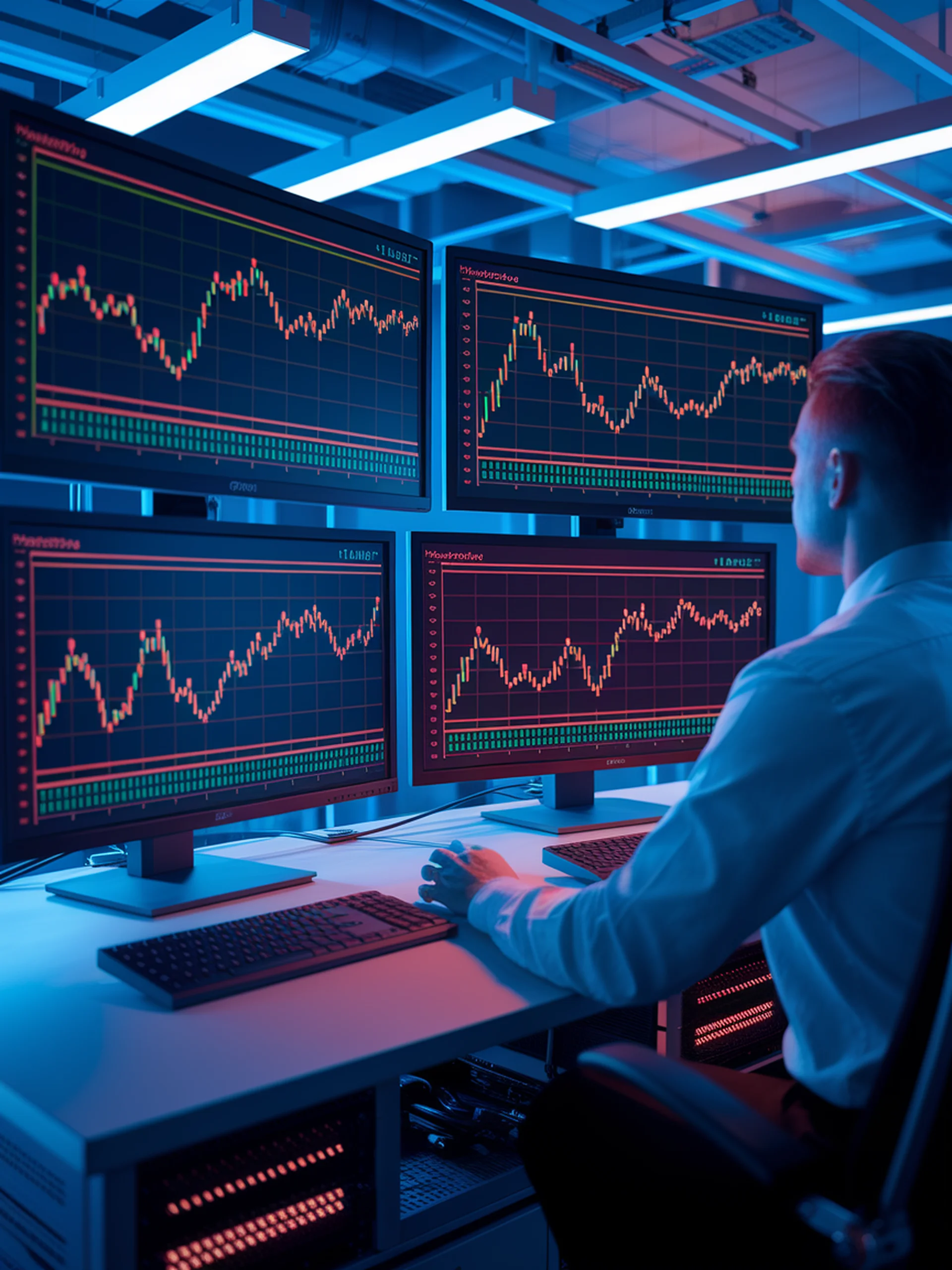
Smarter Anomaly Detection with MADLLM
Leveraging Language Models for Multivariate Time Series Analysis
MADLLM introduces a novel approach to multivariate anomaly detection by aligning time series data with pre-trained large language models through a triple encoding technique.
- Solves the modality gap between multivariate time series data and text-based LLMs
- Preserves critical inter-variable relationships that previous approaches missed
- Enhances security monitoring by detecting complex anomalies across multiple variables
- Demonstrates superior detection accuracy compared to traditional methods
Security Impact: By maintaining the relationships between variables, MADLLM enables more accurate detection of sophisticated security threats that manifest as subtle patterns across multiple indicators.
Original Paper: MADLLM: Multivariate Anomaly Detection via Pre-trained LLMs