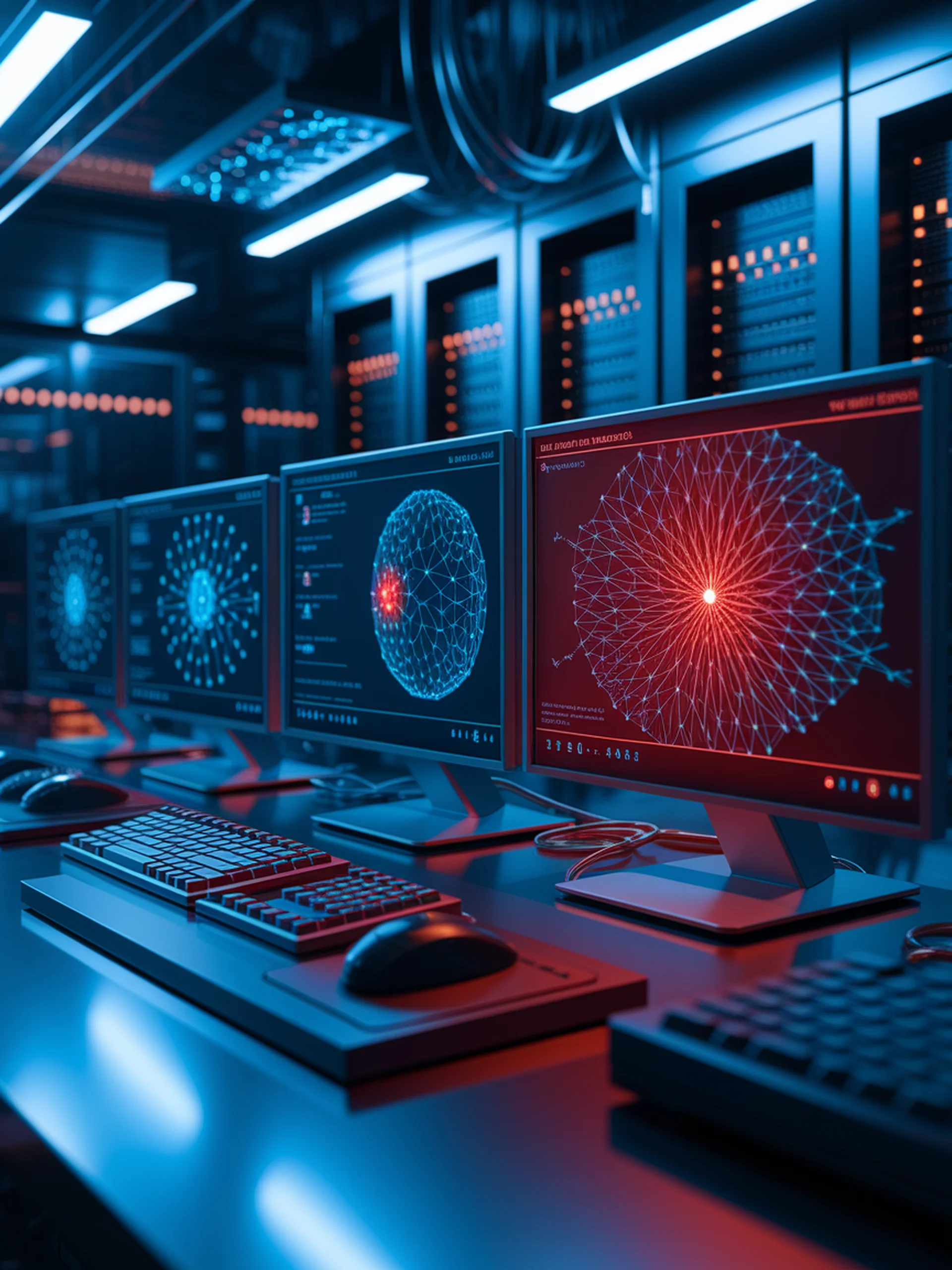
Detecting Ghost Behaviors in LLMs
A novel forensic approach for identifying abnormal LLM outputs
This research introduces a groundbreaking framework for detecting abnormal behaviors in Large Language Models by analyzing hidden state patterns, achieving over 95% detection accuracy.
- Identifies hallucinations, jailbreak attempts, and backdoor exploits through hidden state forensics
- Provides a practical solution for enhancing the security and reliability of LLM applications
- Addresses critical vulnerabilities being exploited by malicious actors in deployed systems
- Offers a new protective layer for organizations deploying LLMs in sensitive environments
This innovation is particularly valuable for security teams as it enables real-time monitoring of LLM outputs without requiring model retraining or architectural changes, significantly reducing security risks in production environments.