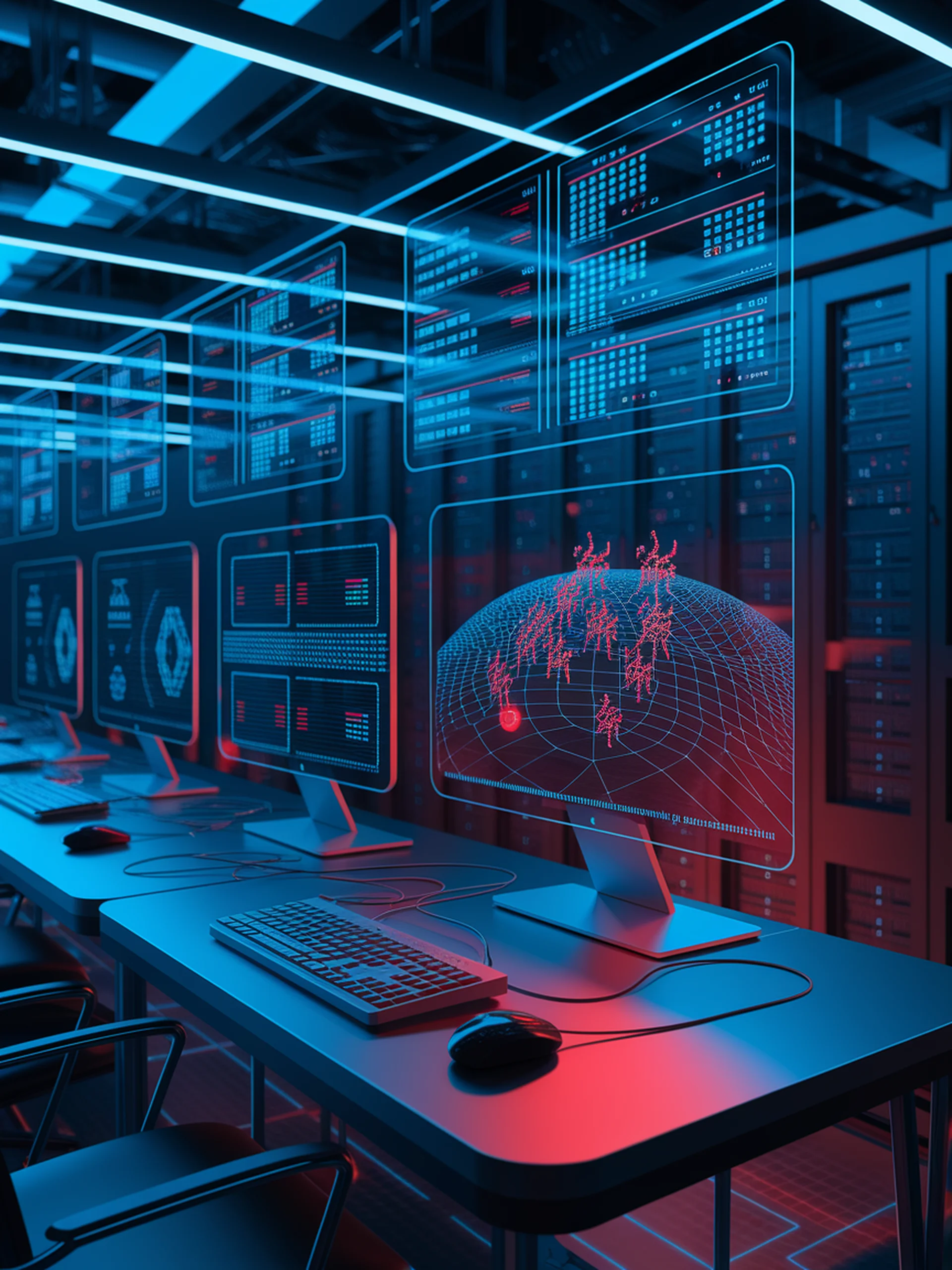
AnomalyGen: Revolutionizing Log-Based Security
Using LLMs to Generate Realistic Anomaly Data for Better Detection
AnomalyGen is the first automated framework that leverages large language models to generate high-quality, semantically meaningful log sequences specifically for anomaly detection tasks.
- Addresses critical dataset scarcity in log-based anomaly detection research
- Overcomes limitations of existing datasets: incomplete coverage, artificial patterns, and poor semantics
- Generates realistic anomaly scenarios with complete semantic context
- Enables more robust and reliable security monitoring systems
This research significantly advances security capabilities by providing high-fidelity synthetic data for training detection systems, potentially improving threat detection accuracy in production environments.
AnomalyGen: An Automated Semantic Log Sequence Generation Framework with LLM for Anomaly Detection