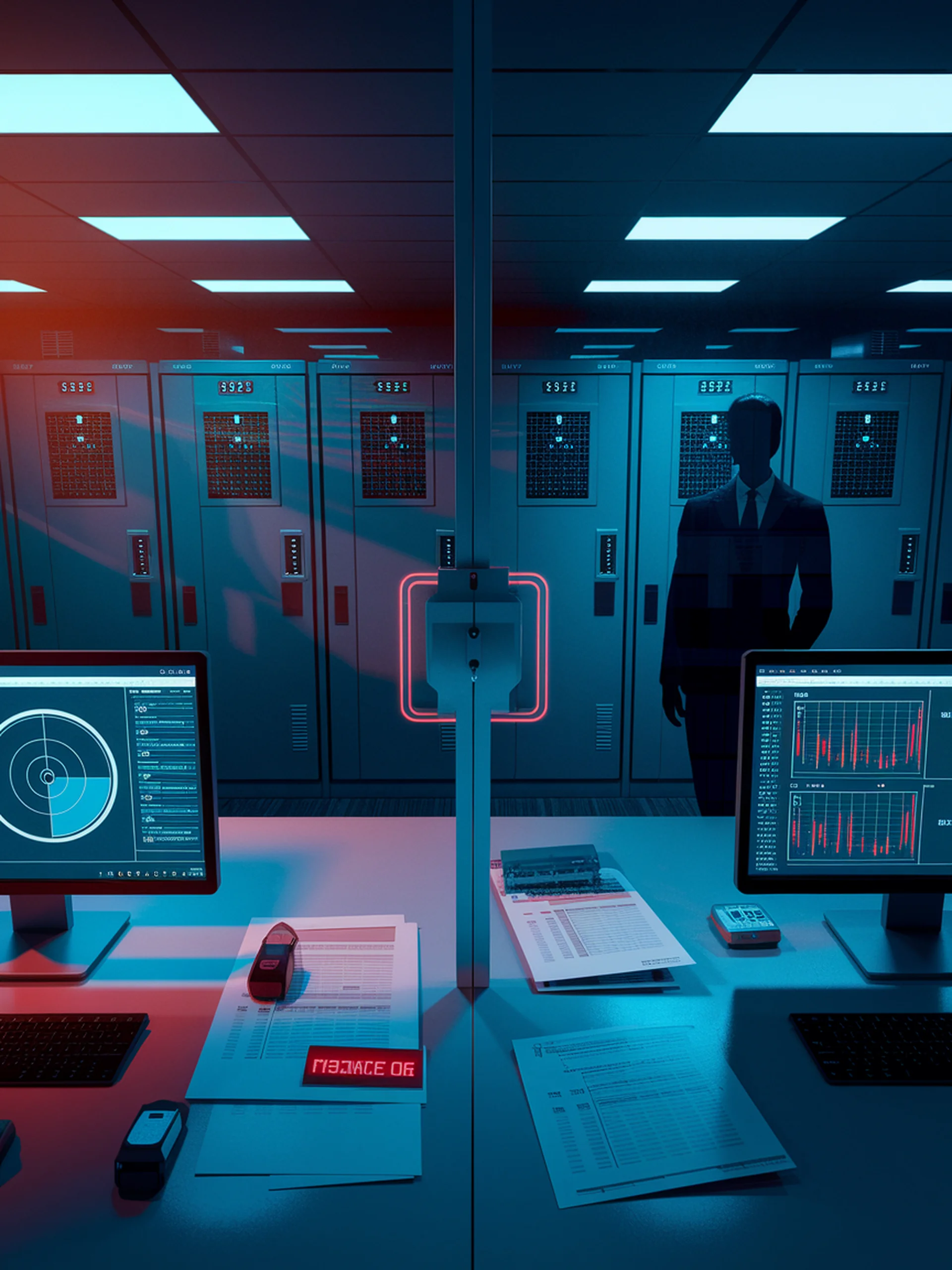
The Hidden Dangers of Private LLM Evaluations
Security risks in closed-door model assessments
This research exposes critical security vulnerabilities in private LLM evaluations conducted by third-party data curators, highlighting significant conflicts of interest and data integrity concerns.
Key Findings:
- Private evaluations lack transparency and accountability mechanisms, creating opportunities for manipulation
- Third-party evaluators face potential conflicts of interest when assessing competing models
- Data contamination and prompt leakage risks are amplified in closed evaluation environments
- Security vulnerabilities threaten the integrity of the entire LLM evaluation ecosystem
Why It Matters: As organizations increasingly rely on third-party evaluations for critical LLM deployment decisions, these hidden security risks could significantly impact product development trajectories and investment strategies across the AI industry.
Peeking Behind Closed Doors: Risks of LLM Evaluation by Private Data Curators