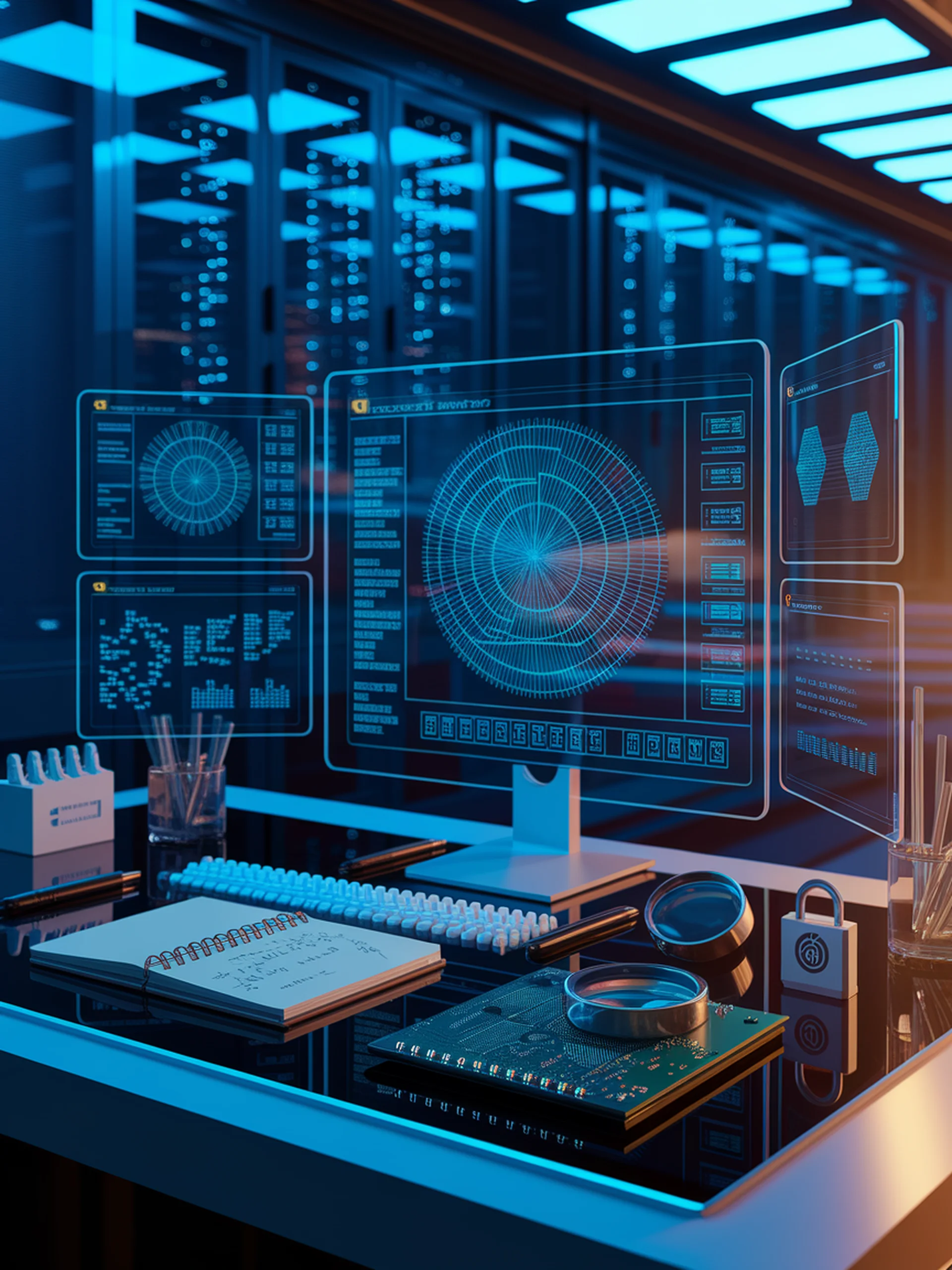
Unveiling the Black Box of LLM Training Data
A novel approach to detect data imprints in proprietary models
This research introduces techniques to identify training data used in proprietary LLMs without requiring access to the underlying model or data.
- Creates information-theoretic measures to detect memorized content in LLMs
- Enables external verification for potential copyright infringement
- Addresses critical transparency gaps in commercial AI systems
- Empowers data authors with greater agency over their content
For security professionals, this work provides crucial tools to audit AI systems, verify unauthorized data usage, and establish accountability in an increasingly AI-dependent landscape.
Information-Guided Identification of Training Data Imprint in (Proprietary) Large Language Models