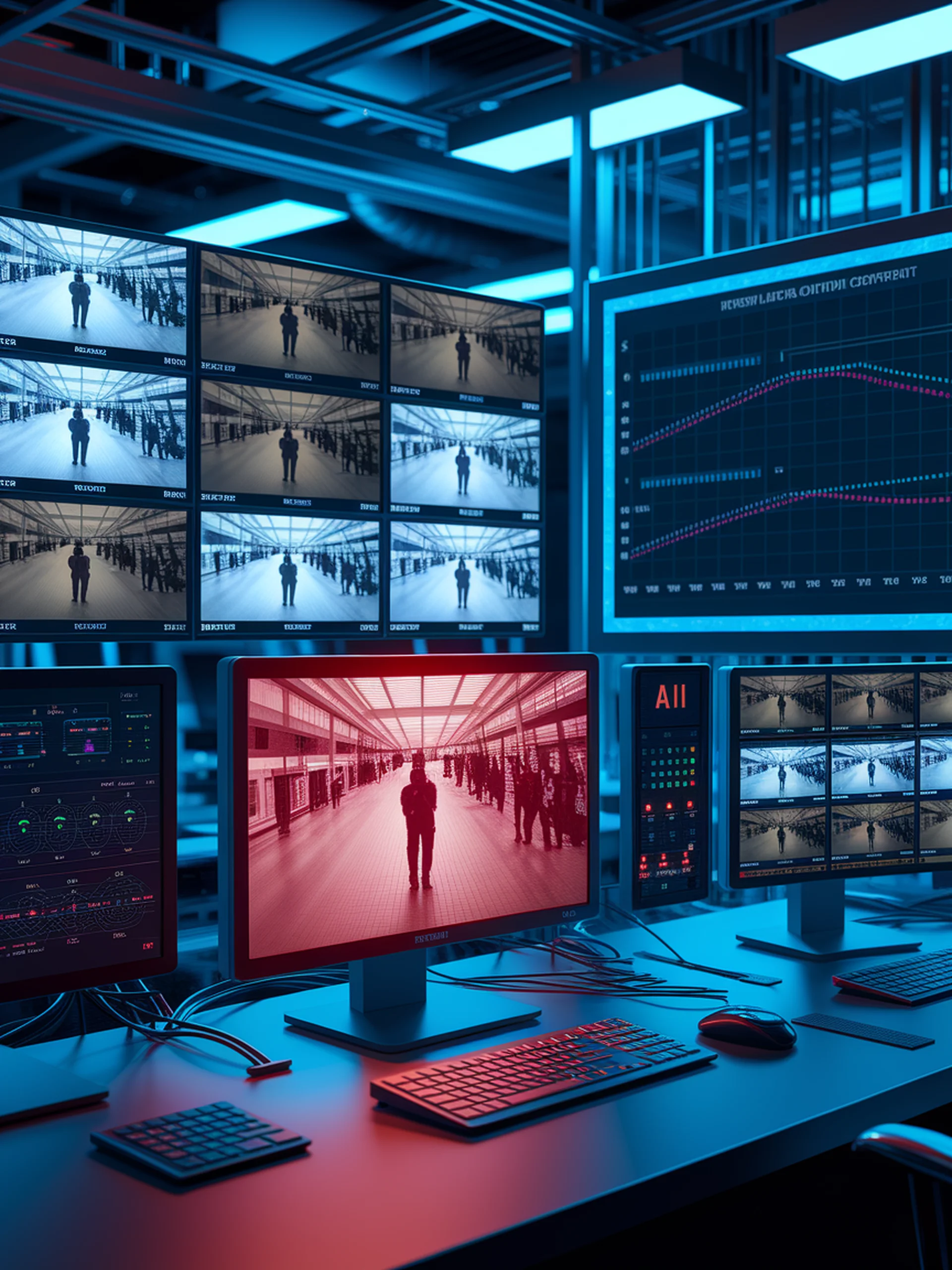
Next-Gen Video Anomaly Detection
Understanding anomalies at multiple time scales and contexts
Holmes-VAU introduces a groundbreaking approach to video anomaly detection that works across different temporal scales, from brief incidents to long-term patterns.
- Developed HIVAU-70k, a large-scale dataset with hierarchical annotations for video anomalies
- Created a multi-level understanding framework that can identify both short-term and long-term anomalous events
- Employs multimodal analysis combining visual and textual data for improved detection accuracy
- Delivers interpretable results that explain the nature of detected anomalies
This research significantly enhances security surveillance capabilities by enabling systems to understand complex anomalous patterns in real-world contexts, leading to more reliable threat detection and reduced false alarms.
Holmes-VAU: Towards Long-term Video Anomaly Understanding at Any Granularity