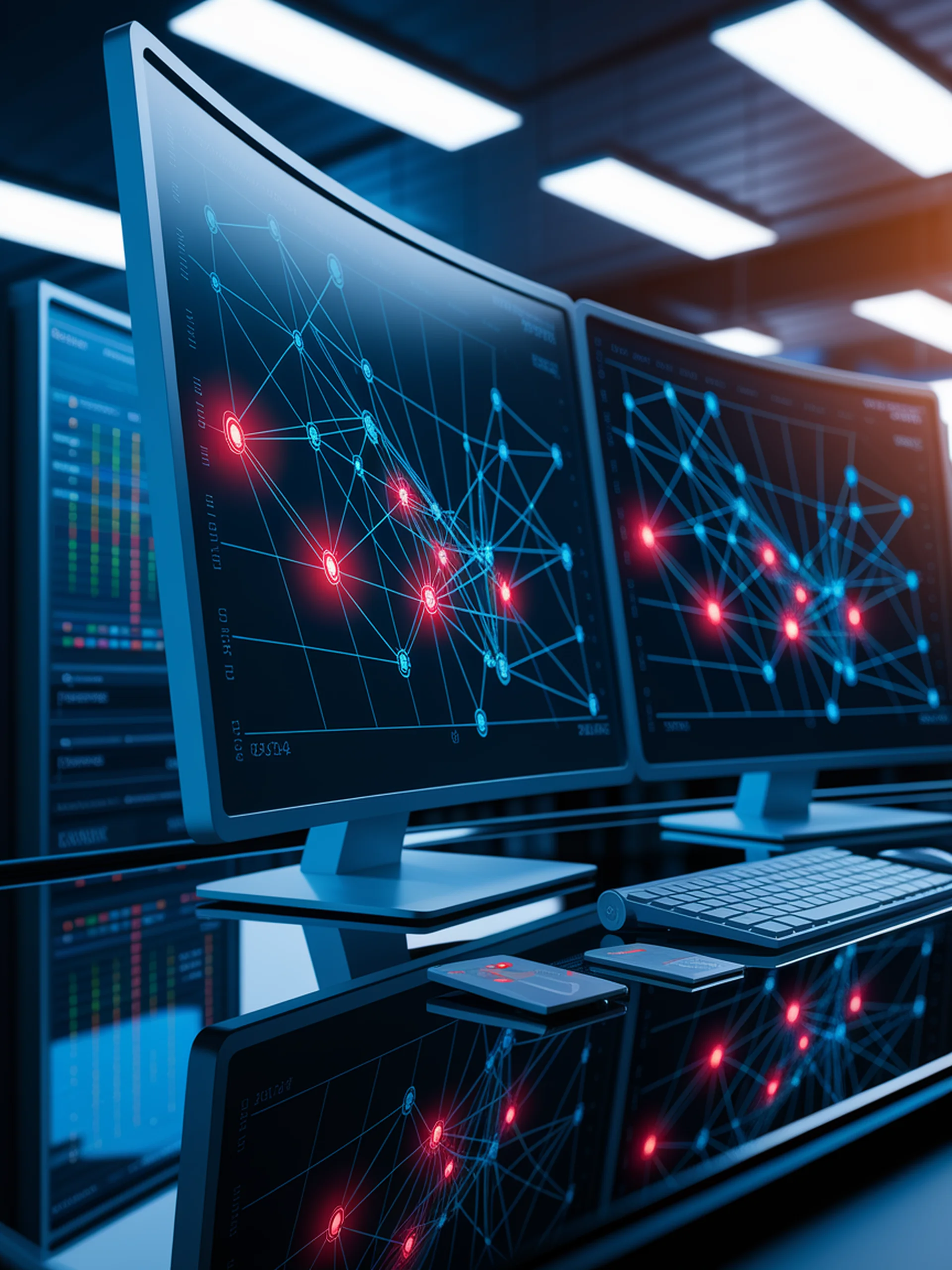
Smarter Anomaly Detection with Minimal Examples
Enhancing security systems through graph-based visual prompts
This research introduces a novel Kernel-aware Graph Prompt Learning approach that significantly improves anomaly detection with just a few normal samples.
- Leverages multi-layer visual interactions rather than relying solely on text prompts
- Captures intrinsic relationships between visual features using graph-based architecture
- Achieves superior anomaly detection performance with fewer examples
- Particularly valuable for security applications where anomalous data is rare
For security systems, this advancement enables more efficient threat detection with minimal training data, helping identify unusual patterns that could indicate security breaches or operational anomalies.
Kernel-Aware Graph Prompt Learning for Few-Shot Anomaly Detection