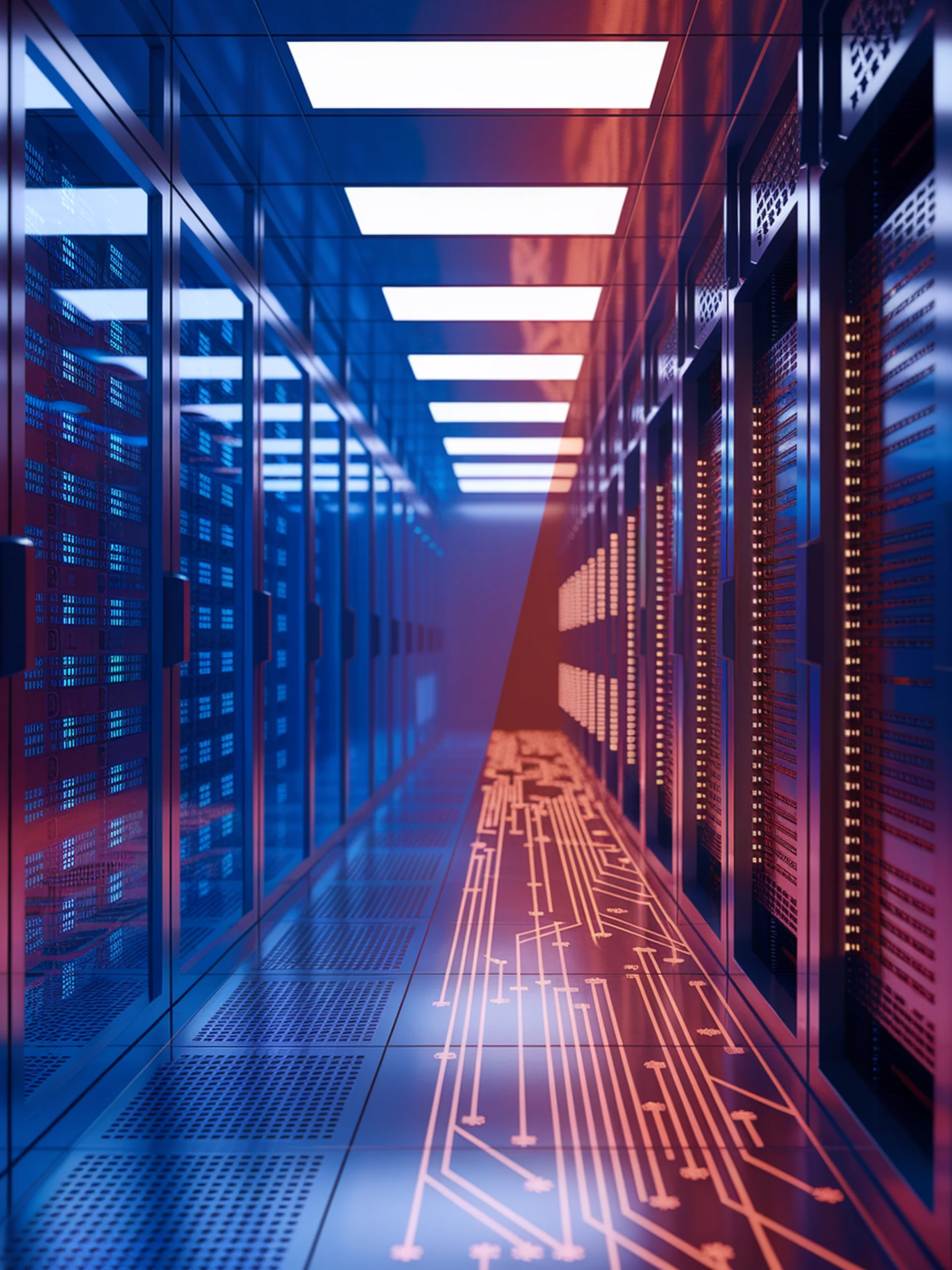
The Privacy-Performance Trade-off in LLMs
Why there's no perfect solution for private LLM inference
This research establishes a fundamental No Free Lunch Theorem for privacy-preserving LLM inference, demonstrating an inherent trade-off between privacy protection and model performance.
- Proves mathematically that perfect privacy preservation inevitably degrades model performance
- Establishes a theoretical framework for evaluating the balance between privacy and utility
- Demonstrates that different privacy mechanisms offer distinct trade-offs, with no universally optimal solution
- Provides guidance for selecting appropriate privacy mechanisms based on specific use cases
For security professionals, this research offers crucial insights for designing LLM systems with appropriate privacy safeguards while maintaining acceptable performance levels for business applications.