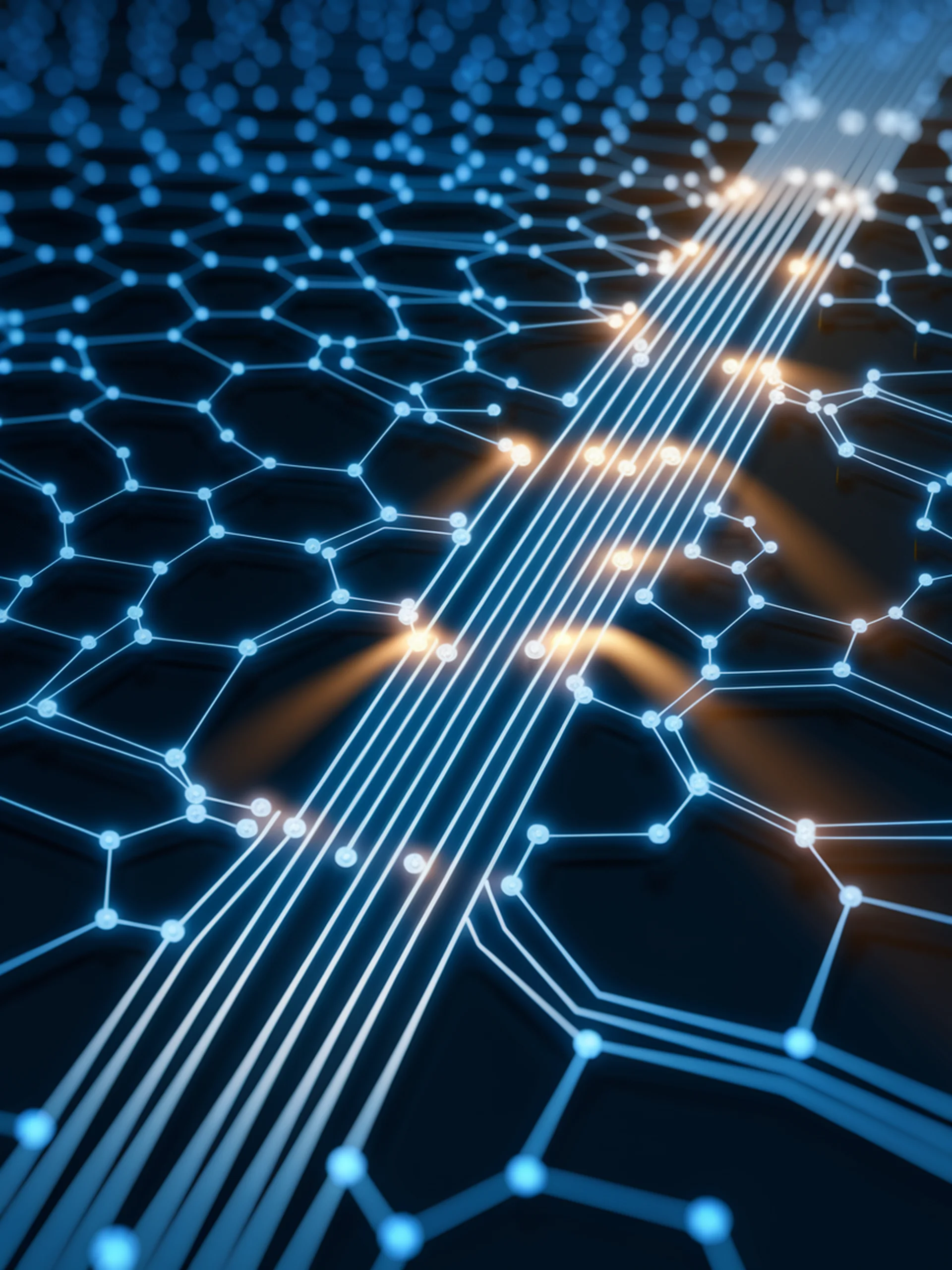
The Blind Spots in LLM Unlearning
Developing more robust evaluation frameworks for data removal
This research addresses critical gaps in how we evaluate machine unlearning methods for Large Language Models, essential for reliable data removal capabilities.
- Identifies limitations in current evaluation approaches for LLM unlearning
- Recommends more comprehensive assessment frameworks to measure true unlearning efficacy
- Proposes standardized methodologies to compare different unlearning techniques
- Emphasizes security implications of incomplete or inadequate unlearning
For security professionals, this work provides crucial insights into verifying that sensitive data is genuinely removed from models, protecting against data extraction attacks and ensuring regulatory compliance. Proper evaluation frameworks are essential as unlearning becomes a key component of responsible AI deployment.
Towards Effective Evaluations and Comparisons for LLM Unlearning Methods